AWS Redshift Competitors: A Thorough Examination
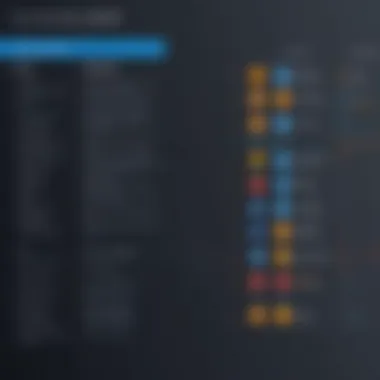
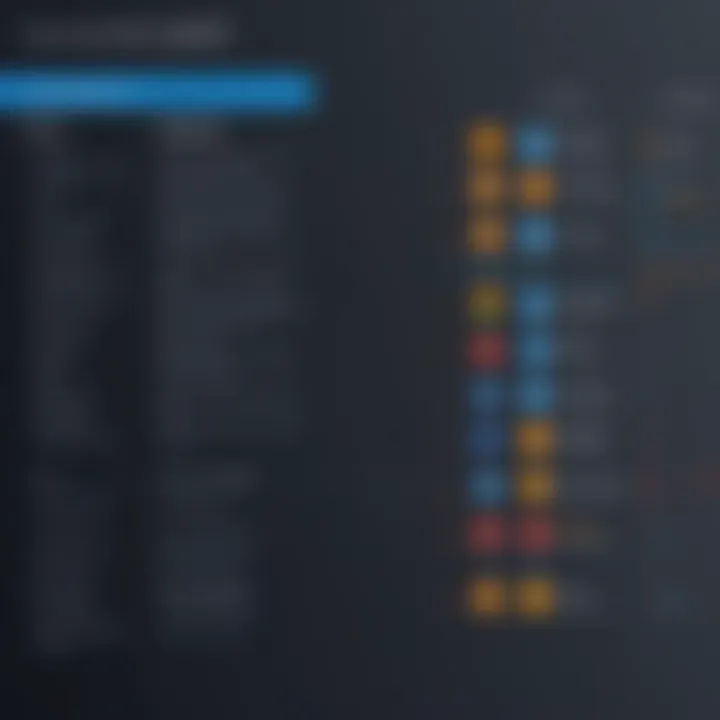
Intro
In the evolving world of data warehousing, AWS Redshift stands out as a robust solution for many organizations. However, as the data landscape changes, so do the alternatives and competitors that provide similar capabilities. Understanding these options is crucial for businesses aiming to optimize their data strategy. This article explores the significant competitors to AWS Redshift, evaluating their unique features, strengths, and potential weaknesses.
In this analysis, IT professionals and business leaders will find relevant insights that guide their decision-making process. By delving into the characteristics of various data warehousing solutions, we can unveil comparative advantages and drawbacks that may impact organizational objectives.
This overview sets the foundation for a more detailed examination, ensuring that any organization considering data storage solutions can make informed choices based on comprehensive knowledge of the current market.
Software Overview
Software category and its purpose
Data warehousing solutions serve a pivotal role in modern data management by allowing organizations to store, retrieve, and analyze large volumes of data efficiently. AWS Redshift, in particular, utilizes a columnar storage architecture that optimizes the performance of complex queries. Competitors in this space also aim to harness the power of data, each with their unique approach to storing and querying information.
Key features and functionalities
Competitors offer various features:
- Scalability: Solutions like Google BigQuery are known for their capacity to scale effortlessly, handling fluctuating workloads.
- Performance optimization: Snowflake boasts advanced optimization techniques, allowing rapid query execution.
- Integration capabilities: Azure Synapse integrates seamlessly with other Microsoft services, enhancing its appeal for businesses already entrenched in the Microsoft ecosystem.
These features serve as key differentiators, impacting the choice between different data warehousing platforms. Each competitor not only fulfills the fundamental requirements of data warehousing but also introduces unique functionalities that enhance user experience.
Comparative Analysis
Comparison with similar software products
When contrasting AWS Redshift with its competitors, the discussion often focuses on performance, pricing, and ease of use. For example, while AWS Redshift has a strong pricing model based on reserved instances, Google BigQuery employs a pay-as-you-go pricing structure enabling better cost management for variable workloads.
In terms of performance, Snowflake is designed for high concurrency and quick query responses, making it an attractive option for data-intensive organizations.
Market positioning and user demographics
Organizations choosing their data warehousing solutions often align their choice with their existing technological investments and the skillsets of their teams. Redshift finds favor among large enterprises with significant Amazon Web Services setups, while Snowflake reaches users from varied backgrounds because of its cloud-agnostic nature. The typical user demographics demonstrate a range of sectors: from finance and healthcare to retail and tech.
The choice of data warehousing solution can significantly impact many aspects of business operations, from cost to performance.
By analyzing these dimensions, organizations will gain clarity regarding which solution aligns most closely with their objectives.
Understanding AWS Redshift
AWS Redshift serves as a pivotal element in modern data warehousing solutions. Understanding its features, capabilities, and limitations is essential for any organization looking to optimize its data management strategy. Each aspect of Redshift elucidates the performance metrics and operational efficiencies that are central to data analytics.
This section will explore the definition, key attributes, and the relative strengths and weaknesses of AWS Redshift. It is crucial for stakeholders to grasp how this service compares with its alternatives to make informed decisions that align with their business goals.
What is AWS Redshift?
AWS Redshift is a fully managed data warehouse service designed by Amazon Web Services. It enables organizations to efficiently analyze large volumes of data using SQL and various business intelligence tools. The service operates on a cluster-based architecture, allowing for scalable and cost-effective storage. Users can query petabytes of structured and semi-structured data quickly by simply adjusting their cluster's configuration based on operational demand.
Key Features of AWS Redshift
AWS Redshift has several notable features:
- Columnar Storage: Redshift stores data in a column-oriented format, which enhances query performance and speeds up data retrieval.
- Massively Parallel Processing (MPP): The MPP architecture enables multiple nodes to work in parallel, making data processing efficient.
- Scalability: Users can easily scale their Redshift clusters up or down to meet changing workloads.
- Integration: It integrates seamlessly with a variety of data sources and tools, including Amazon S3, Microsoft Excel, and Tableau.
- Data Compression: Redshift supports advanced data compression techniques to reduce storage costs.
- Security Features: Redshift provides security with features like data encryption at rest and in transit, as well as VPC isolation.
Strengths and Limitations
AWS Redshift holds several strengths that contribute to its popularity:
- Performance: Its speedy query performance is a significant advantage, particularly for large data sets.
- Cost-Effectiveness: It offers competitive pricing models, making it accessible for various organizations.
- Ease of Use: The user interface is friendly for those familiar with SQL, thus facilitating easy adoption and use.
However, there are also limitations to consider:
- Limited Real-Time Processing: Redshift is optimized for analytical workloads but may not be ideal for real-time data processing.
- Complexity in Management: While AWS manages much of the infrastructure, users need some expertise for optimal configuration and management.
- Concurrency Issues: High concurrent workloads can degrade performance, affecting user experiences.
Understanding these various aspects of AWS Redshift will provide a solid foundation for discussing its competitors later in this article. Recognizing both its capabilities and limitations will help guide organizations in selecting data warehousing solutions that are best suited to their unique needs.
Major Competitors to AWS Redshift
In the constantly evolving landscape of data warehousing, recognizing the major competitors to AWS Redshift is essential for businesses seeking effective solutions for managing and analyzing large data sets. Each of these alternatives offers unique capabilities that cater to different organizational needs. Understanding these competitors allows decision-makers to weigh their options carefully. This examination equips IT professionals and business leaders with insights to align their data management strategies with current technologies.
Google BigQuery
Overview of Google BigQuery
Google BigQuery is a serverless data warehouse solution that stands out for its scalability and speed. It utilizes a fully managed architecture, which means users do not have to worry about maintenance, updates, or infrastructure management. Its ability to handle very large datasets with ease positions it as a robust alternative to AWS Redshift. Additionally, the integration with other Google Cloud services makes it a compelling choice for companies already using the Google ecosystem.
Key Features
One of the key features of Google BigQuery is its capacity for real-time analytics. Users can run SQL-like queries against massive amounts of data without worrying about performance bottlenecks. The pay-as-you-go model is another attractive aspect, allowing organizations to be billed only for the data they analyze. This can lead to significant cost savings compared to traditional databases. Additionally, its machine learning tools enable users to generate predictive insights directly within the platform, thus enhancing its analytics capabilities.
Comparative Strengths
Google BigQuery shines when it comes to handling large volumes of data quickly and efficiently. The built-in machine learning functionalities and seamless integration with Google's AI tools provide added value. A potential weakness, however, is its dependency on Google Cloud infrastructure, which could lock users into a specific ecosystem, limiting flexibility.
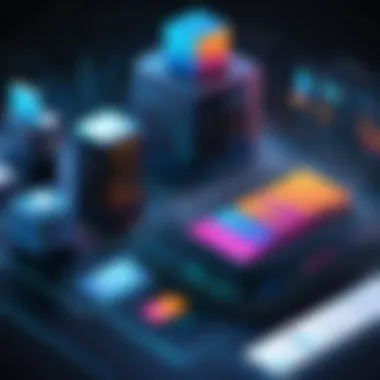
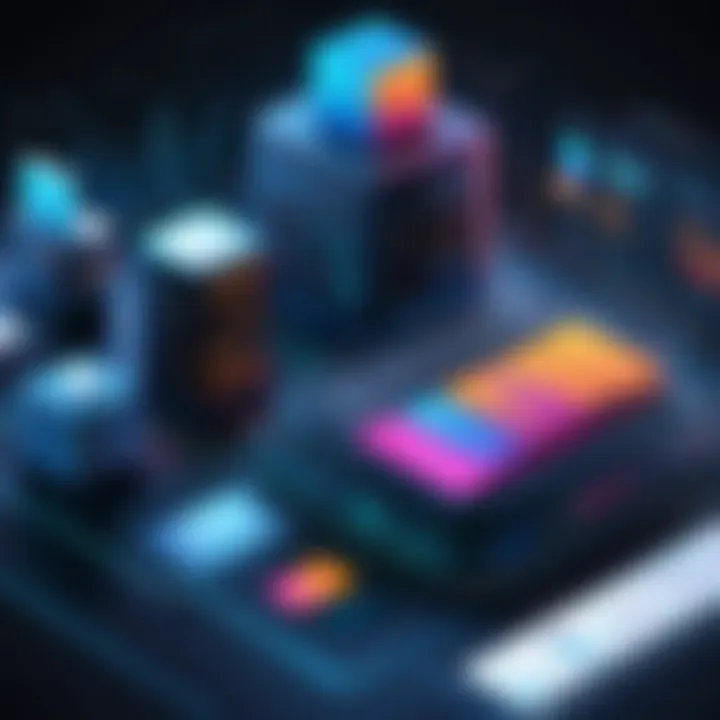
Use Cases
Organizations looking for fast analytics and real-time data processing often find Google BigQuery to be a suitable choice. It is particularly popular among companies in media and entertainment. These sectors require rapid data insights to make informed decisions. Its ability to effortlessly integrate with various Google services only enhances its attractiveness.
Microsoft Azure Synapse Analytics
Overview of Azure Synapse
Microsoft Azure Synapse Analytics combines big data and data warehousing into one solution. It connects various analytic services and provides a more holistic view of data management. The integration with Azure services accelerates data movement and analytics processes, making it a capable contender against AWS Redshift. Moreover, its support for various programming languages fosters versatility in data analytics.
Key Features
A significant feature of Azure Synapse is its capability to analyze both relational and non-relational data types. Users can create complex queries that pull from both structured and unstructured data sources, making it a versatile tool for modern analytics. The incorporation of Synapse Pipelines enables automated data actions and workflows, enhancing productivity and speed.
Comparative Strengths
Azure Synapse stands out for its flexibility and strong integration capabilities. By allowing an easy transition between data lakes and warehouses, it supports various analytics approaches. However, it might require steep learning curves for users unfamiliar with the Azure environment, which could be a consideration for potential adopters.
Use Cases
Organizations involved in comprehensive analytics projects, such as financial services and healthcare, often lean towards Microsoft Azure Synapse. Its capacity for unified analytics across diverse data sources supports detailed insights needed in these industries.
Snowflake
Overview of Snowflake
Snowflake is a cloud-based data warehousing platform designed for ease of use and scalability. Its architecture allows for automatic scaling, which makes it well-suited for high-demand environments. Snowflake’s approach to data sharing also facilitates collaboration across different teams without compromising data governance, a noteworthy characteristic in today’s collaborative work landscape.
Key Features
A standout feature of Snowflake is its ability to separate storage and compute resources. This allows users to scale them independently based on workload, leading to cost savings and improved performance. The platform also supports a multi-cloud strategy, giving users the flexibility to run on AWS, Azure, or Google Cloud—thus preventing vendor lock-in.
Comparative Strengths
Snowflake's user-friendly interface and powerful data sharing capabilities enhance its position in the market. Its unique architecture is beneficial for businesses that require agile data operations. However, users may find pricing to be a challenge, as costs can accumulate with extensive data processing and storage needs.
Use Cases
Companies in the e-commerce and retail sector frequently adopt Snowflake for its strong data sharing capabilities and real-time analytics. Its effective handling of large datasets makes it ideal for organizations that rely on timely decision-making based on data insights.
IBM Db2 Warehouse on Cloud
Overview of IBM Db2
IBM Db2 Warehouse on Cloud offers a fully managed data warehousing solution with powerful analytics capabilities. It emphasizes robustness and performance, suitable for enterprises with extensive data needs. The built-in data protection and security features make it an option for organizations concerned about data security and compliance.
Key Features
Key features of IBM Db2 include its advanced in-database analytics functionality and strong integration with AI and machine learning technologies. It facilitates high-performance analytics over structured and unstructured data, which can accelerate business processes, especially in data-intensive environments.
Comparative Strengths
IBM Db2 is particularly noted for its security protocols, making it ideal for sectors such as finance and healthcare where data sensitivity is crucial. However, it might not be as user-friendly for companies without a strong technical background, potentially making the onboarding process challenging.
Use Cases
Businesses dealing with finance and healthcare often opt for IBM Db2 due to its compliance capabilities and security. Its advanced analytics tools also benefit organizations seeking to derive insights from diverse datasets.
Teradata Vantage
Overview of Teradata
Teradata Vantage is designed for large-scale enterprise analytics. It provides an integrated platform for data analytics and empowers organizations to make complex data-driven decisions. The emphasis on multi-cloud compatibility gives Teradata a strategic edge in the market.
Key Features
One defining characteristic of Teradata Vantage is its capability for real-time analytics combined with advanced data management. The platform supports a wide spectrum of analytical workloads, making it a versatile choice for various applications. These features enhance decision-making speed in complex enterprise environments.
Comparative Strengths
Teradata Vantage excels in providing a comprehensive solution for large organizations that require complex data operations. However, the infrastructure setup and maintenance might demand higher resources and expertise, which can be a barrier for smaller businesses.
Use Cases
Organizations across various sectors dealing with extensive data flows, such as telecommunications and retail, find Teradata Vantage to be an optimal choice. Its capability to manage significant data loads and complex analytics supports informed decision-making.
Emerging Alternatives
The emergence of alternatives to AWS Redshift signifies a critical shift in the landscape of data warehousing solutions. As businesses evolve, their data needs frequently become more complex. Organizations are actively looking for tailored solutions that not only satisfy current demands but also scale with growing data requirements. This section examines several emerging technologies that present unique offerings and competitive advantages when compared to AWS Redshift.
By exploring these emerging alternatives, organizations can better understand how to leverage diverse technologies to achieve efficiency, flexibility, and innovation in their data strategies. Each alternative presents its own strengths that might align closely with specific business objectives or operational challenges. A thorough exploration of these alternatives is essential for IT decision-makers and business leaders, who must consider both immediate needs and long-term goals when investing in data warehousing solutions.
Apache Druid
Overview of Apache Druid
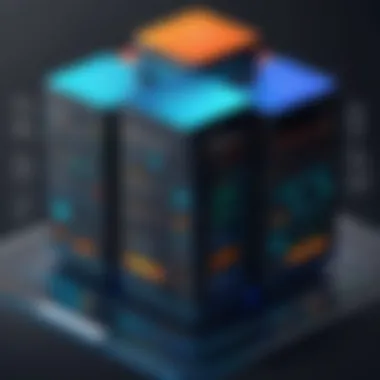
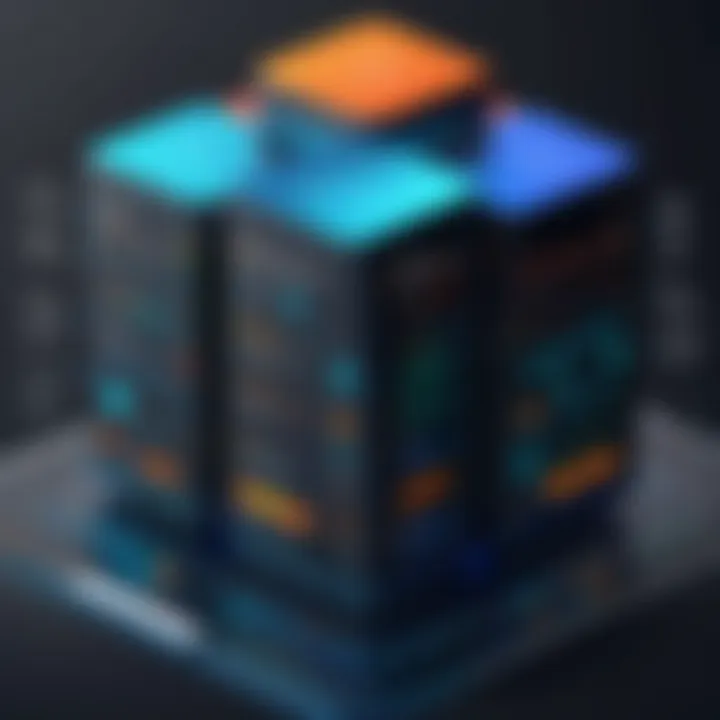
Apache Druid is an analytic data store designed for online analytical processing (OLAP). Its architecture focuses on the needs of real-time data ingestion and fast query performance. Druid is particularly notable for its ability to handle large volumes of streaming data while supporting sub-second query response times. This makes it a popular choice for applications that require immediate insights from fast-changing datasets.
One key characteristic of Druid is its multi-dimensional data model, which allows users to easily slice and dice data across various dimensions, facilitating quick and effective analytics. The unique feature of Druid lies in its data partitioning approach, which optimizes for both storage and query performance. Its ability to support both batch and real-time processing gives it an edge in environments where immediate data accessibility is crucial.
Key Features
Druid boasts several features that distinguish it from other data storage solutions. These include:
- Real-Time Data Ingestion: Druid can ingest data in real-time, making it suitable for time-sensitive applications.
- Fast Aggregation Queries: Its design allows for rapid responses even when executing complex queries with large datasets.
- Scalability: Druid's architecture is designed to handle growing data loads without significant slowdown in performance.
These features are particularly beneficial for organizations that require speed and flexibility in their analytical processes. However, one disadvantage can be the complexity involved in setting up and maintaining a Druid cluster, which may require substantial technical expertise.
Comparative Strengths
When compared with AWS Redshift, Druid’s primary strength is its focus on real-time data processing.
- Speed: Druid often outperforms Redshift in query response times, especially under heavy load.
- Flexibility: Druid's ability to quickly process streaming data is a significant advantage for dynamic datasets.
- Cost-Effectiveness: For workloads focused on fast queries and real-time analytics, businesses may find Druid to be a more economical option compared to Redshift.
However, users may find that Redshift’s integration with other AWS services can lead to enhanced overall performance in different contexts, making the choice dependent on specific use cases.
Use Cases
Druid is ideal for interactive analytics, where users require immediate answers to complex queries. Common use cases include:
- Dashboards and Reporting: Organizations can provide real-time analytics dashboards that reflect the most current data.
- IoT Analytics: Druid excels at processing and analyzing streaming data from Internet of Things devices.
- Log and Event Analysis: Its ability to handle time series data makes it suitable for analyzing logs and event data in real-time.
Despite its advantages, companies might face challenges in setting up the infrastructure and making it compatible with existing systems. Therefore, it's crucial to ensure that Druid aligns with the organization’s technical capabilities.
Cloudera Data Warehouse
Overview of Cloudera
Cloudera Data Warehouse is built on top of the Apache Hadoop ecosystem, focusing on providing a scalable and flexible solution for data warehousing. Its hybrid architecture allows organizations to store data either in the cloud or on-premises, catering to various data governance and regulatory needs. Cloudera emphasizes easy management and integration with other tools commonly used by data teams.
One prominent feature of Cloudera is its support for multiple data formats and storage policies, giving users the freedom to manage resources effectively based on their requirements. This creates a highly adaptable environment for organizations that deal with diverse datasets.
Key Features
Cloudera incorporates a variety of essential features, such as:
- Support for Multiple Data Sources: Cloudera can connect to various data sources, facilitating a comprehensive data ecosystem.
- Robust Security Features: Its built-in security measures are designed to ensure compliance with data regulations.
- Extensive Analytical Tools: Integration with a wide range of analytics tools enhances its usability in data processing and visualization.
These features make it an appealing choice for enterprises looking to centralize their data infrastructure. However, the complexity of its architecture may intimidate smaller organizations or those lacking extensive IT resources.
Comparative Strengths
Cloudera offers notable strengths when compared to AWS Redshift:
- Flexibility: Its ability to run either on-premises or in the cloud provides organizations with options suited for various infrastructure needs.
- Comprehensive Data Governance: The deep integration with Hadoop enables sophisticated data management strategies.
- Support for Large Datasets: Cloudera is adept at handling vast amounts of data, making it suitable for industries dealing with large-scale datasets.
On the downside, users may find Redshift to be easier to set up and manage, particularly if they are already embedded within the AWS ecosystem.
Use Cases
Cloudera Data Warehouse is commonly employed in use cases that require extensive data governance and flexibility in deployment. These include:
- Data Lakes: Cloudera often serves as a management solution for data lakes, allowing for efficient data storage and processing.
- Enterprise Data Management: Its tools are beneficial for developing a centralized data management approach.
- Advanced Analytics: Organizations utilize Cloudera to run advanced analytical models on large datasets, making it useful for deep data insights.
While Cloudera has significant advantages, the initial learning curve and setup process should be weighed against organizational readiness.
Vertica
Overview of Vertica
Vertica is a columnar storage platform designed for big data analytics. Its architecture prioritizes performance, enabling fast query execution through advanced compression techniques and optimizations tailored for analytical workloads. Vertica is often praised for its ability to efficiently handle large volumes of structured and semi-structured data.
A distinctive feature of Vertica is its focus on providing a high level of performance with a relatively low infrastructure footprint. This attribute can be extremely valuable for organizations with limited resources but extensive analytical needs.
Key Features
Several key features set Vertica apart:
- Columnar Storage: This storage model significantly enhances query performance by enabling efficient data access.
- Advanced Compression: Vertica's compression methods help reduce storage costs and improve retrieval times.
- Elasticity: It provides elastic scaling, allowing organizations to increase or decrease capacity as required.
These features make Vertica appealing for businesses needing effective data management solutions while keeping costs in check. However, smaller companies might struggle with the investment required for comprehensive implementation.
Comparative Strengths
Vertica stands out in the area of performance:
- Fast Query Performance: When handling large datasets, Vertica can deliver impressive query response times.
- Efficient Storage Use: The columnar storage format combined with compression techniques leads to efficient use of storage resources.
- Strong Analytics Capabilities: Vertica offers powerful analytic functions that support complex calculations and data manipulation.
In contrast, AWS Redshift may still hold advantages in integration with the AWS ecosystem and ease of accessibility for users familiar with cloud-native solutions.

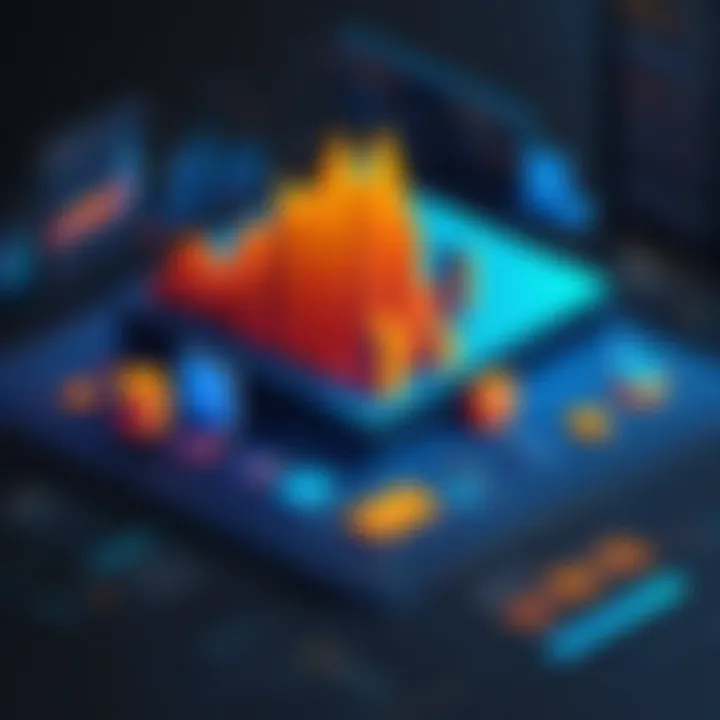
Use Cases
Vertica finds its application in many data-driven environments. Typical use cases include:
- Business Intelligence: Organizations utilize Vertica for advanced reporting and dashboarding for quick insights.
- Data Science Projects: Its ability to handle extensive datasets makes it ideal for data science and machine learning applications.
- Telecommunications Analytics: Vertica is commonly used to analyze vast amounts of call data records in the telecommunications industry.
Despite its merits, the initial setup time and complexity can pose challenges for teams. Thus, proper planning and resources are key to leveraging Vertica effectively.
Factors to Consider in Choosing a Data Warehouse
Choosing an appropriate data warehouse goes beyond just evaluating a single solution. It requires a comprehensive analysis of various factors that can greatly impact the performance and suitability of the warehouse for specific business needs. In the landscape of increasingly diverse data warehousing options, these factors provide a foundational understanding for IT professionals and business leaders alike.
Making an informed decision necessitates careful contemplation of cost, performance, scalability, integration capabilities, and user experience. This approach should not only reflect on immediate needs but also consider long-term implications.
"The right data warehouse can significantly optimize data handling and analysis within your organization."
Cost Factors
Cost is often the primary consideration when evaluating data warehousing solutions. Understanding the pricing model of each service can reveal hidden expenses related to storage, query processing, and data transfer. For instance, AWS Redshift has pricing based on node types and usage patterns. Therefore, businesses must calculate their expected data workload to estimate costs accurately.
Moreover, consider potential cost savings offered by different vendors. Some may provide free tiers or more flexible pricing structures that can accommodate your requirements as they grow. Look for:
- Upfront and recurring costs
- Discounts for longer commitments
- Penalties for exceeding storage limits
Performance Metrics
Performance metrics are essential in ensuring that a data warehouse can handle the required workloads efficiently. This includes query response times, data load times, and the capability to process concurrent queries. Each solution will present different performance benchmarks.
Evaluating performance often involves:
- Conducting load testing to check responsiveness
- Reviewing case studies or customer testimonials
- Analyzing benchmarks provided by vendors as well as independent analysts
Scalability and Flexibility
In a constantly evolving business environment, scalability is critical. Organizations must adopt systems that can scale with their changing needs. A data warehouse should support growing data volumes and user loads without a dip in performance.
Flexibility in data integration is also vital. Businesses often employ multiple tools and platforms. Hence, the ability of a data warehouse to easily integrate with existing systems can ensure smooth data workflows. Key aspects include:
- Vertical and horizontal scaling support
- Options for on-premise and cloud-based integration
Integration Capabilities
Integration capabilities refer to how well a data warehouse can connect with other tools and platforms. As companies leverage a variety of applications for data ingestion, reporting, and analytics, seamless integration becomes crucial. A strong integration ecosystem can:
- Reduce data silos
- Streamline workflows
- Enhance overall data usability
Look for data warehouses with:
- Pre-built connectors for common applications
- API support for custom integrations
- Data migration tools for easier transitions
User Experience and Support
A user-friendly interface is essential for encouraging team adoption of a data warehousing solution. Consider the ease of use for both technical and non-technical users. Additionally, evaluate the level of customer support offered by each vendor.
Support options, training resources, and community access can significantly enhance the user experience. These may include:
- Documentation and tutorials
- Access to customer service representatives
- Community forums for troubleshooting
Taking these factors into account will lay a robust foundation for selecting a data warehouse that aligns well with your organizational goals and operational requirements. An informed choice here can lead to increased efficiency and a stronger data analysis capability and can also save costs long-term.
Final Considerations and Recommendations
When evaluating data warehousing solutions such as AWS Redshift and its competitors, it becomes clear that the decision-making process involves multiple critical dimensions. The final recommendations not only synthesize the information provided earlier in this article but also serve to guide organizations in making the best choice for their data needs. It is imperative to engage in comprehensive and methodical analysis before committing to a platform. This allows for alignment with organizational strategic goals, optimization of costs, and enhancement of performance outcomes.
Building a Long-Term Strategy
Establishing a long-term strategy for data warehousing is essential for organizations seeking sustainability and growth. Organizations should define their data requirements and anticipate future needs. A forward-thinking approach involves considering scalability and potential shifts in data types or volumes. By identifying how data will grow, businesses can select a solution that accommodates this expansion and avoids costly migrations later on.
Key elements to consider in building a long-term strategy include:
- Scalability: Ensure the choice of data warehouse can efficiently handle increased data loads.
- Flexibility: A model that accommodates various analytical computing needs will support diverse business functions.
- Integration: Analyze how well the chosen data platform integrates with existing systems.
Aligning Business Goals with Data Warehouse Selection
It is crucial that the chosen data warehousing solution aligns with specific business goals. Failing to align these elements can lead to inefficiencies and missed opportunities. A clear understanding of business objectives helps in crafting use cases. When evaluating competitors, consider how their features contribute to your overall goals.
Consider these factors during dissection:
- Cost Efficiency: Does the solution provide a clear return on investment?
- Operational Compatibility: Is the architecture supportive of current practices and systems in place?
- Analytics Support: Does the system facilitate not just storage, but effective data analysis that drives decision-making?
Continuous Evaluation and Adaptation
Once a data warehousing solution has been implemented, the journey does not end. Continuous evaluation and adaptation are critical for maintaining optimal performance. Periodic reassessments of the chosen solution ensure it still meets the evolving needs of the organization. Evaluate performance metrics regularly and gather feedback from users.
Main aspects to monitor include:
- Performance: Is the data warehouse providing the speed and efficiency necessary for analytics tasks?
- Technological Advances: Stay informed about new features or technologies that competitors may introduce.
- Cost Changes: Watch for shifts in pricing strategies that could impact overall cost-effectiveness.
"In an ever-changing technological landscape, flexibility and continuous improvement can set an organization apart from its competitors."
In summary, final considerations and recommendations play a vital role in ensuring that the choice of a data warehouse aligns with strategic objectives, remains adaptable to changes, and supports long-term growth. This approach mitigates future risks associated with data storage and processing.