Understanding IBM Data Lineage for Better Governance
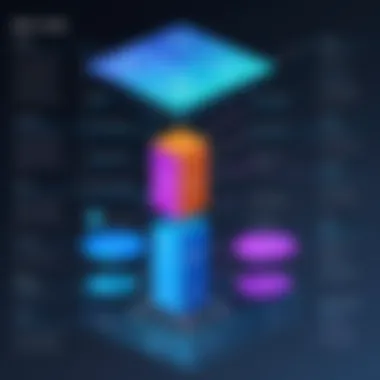
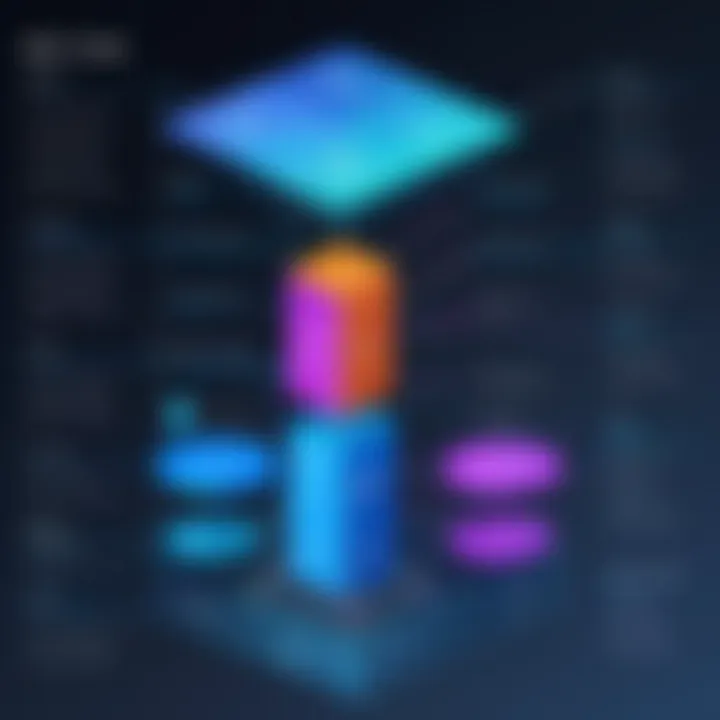
Intro
In todayโs data-driven world, understanding data lineage is critical for effective data governance and overall management. IBM Data Lineage stands out as an important tool within this realm, aiding organizations to trace the origin of their data and comprehend its transformation journey throughout various systems and processes. This exploration not only promotes transparency but also assures data quality and complianceโelements that are vital for informed decision-making in businesses.
This narrative evaluates the concepts, methodologies, tools, and best practices associated with IBM Data Lineage. The objective is to provide IT professionals, software developers, and decision-makers with a thorough understanding of its implications within the IBM ecosystem. By doing so, organizations are better prepared to tackle the complexities associated with data management.
Software Overview
Software category and its purpose
IBM Data Lineage belongs to the broader category of data management and governance solutions. Its primary purpose is to deliver insights into how data flows through an organization. By mapping data's life cycleโfrom creation to storage, processing, and usageโorganizations can gain a detailed understanding of their data landscape. This awareness aids in ensuring compliance with regulations and enhancing overall data quality.
Key features and functionalities
IBM Data Lineage is equipped with various features that cater to the needs of modern organizations:
- Data Mapping: Helps users visualize data flows and transformations, providing clarity on how data is interconnected.
- Impact Analysis: Enables organizations to assess how changes in data can affect downstream processes and systems.
- Data Quality Monitoring: Facilitates the tracking of data anomalies and standards, ensuring that data remains reliable and accurate.
- Collaboration Tools: Promotes communication among stakeholders, allowing them to share insights and work collaboratively on data management initiatives.
These features enhance the ability to maintain control over data assets and support effective decision-making processes.
Comparative Analysis
Comparison with similar software products
When examining IBM Data Lineage, it is essential to consider its standing against competing solutions in the market. Compared to other tools like Informatica and Collibra, IBM Data Lineage offers robust integration with IBM's suite of products and services, allowing for seamless operation within its ecosystem.
For instance, while Informatica provides strong data integration capabilities, IBM excels in its lineage tracking and reporting, thus enabling deeper insights into data transformations. Collibra, known for its governance focus, may not prioritize lineage as extensively as IBM does.
Market positioning and user demographics
IBM Data Lineage is primarily positioned for enterprises that heavily rely on data for strategic decision-making. Its users often include data architects, data stewards, compliance officers, and analytics professionals across various industries. Organizations ranging from large corporations to smaller firms benefit from its comprehensive lineage tracking features, making it a versatile solution.
Understanding these elements helps professionals recognize the importance of data lineage in enhancing operational efficiencies and compliance standards within their organizations.
Prelims to Data Lineage
Data lineage is an essential concept in the field of data management that offers visibility into the life cycle of data as it moves through various systems. As organizations increasingly rely on data to drive decisions and strategies, understanding data lineage becomes crucial. It helps in tracing where data originated, how it transformed, and where it is used through multiple systems and applications. This insight not only supports data governance but also enhances data quality and compliance with regulations.
Definition and Importance
Data lineage refers to the process of recording the flow of data from its origin to its final destination. It includes the various transformations and processes that data undergoes throughout its journey. In essence, it provides a map of data paths, which can be invaluable for several reasons:
- Data Quality Assurance: Understanding the flow and transformations helps in identifying data quality issues early.
- Regulatory Compliance: Many industries have strict regulations regarding data usage and management. Knowledge of data lineage supports compliance efforts by ensuring traceability.
- Impact Analysis: Changes to data models or systems can have significant repercussions. Data lineage enables impact analysis by showing how changes may affect downstream applications or departments.
- Data Governance: Effective data lineage contributes to stronger governance practices by ensuring accountability and clarity in data usage across the organization.
Relevance in Modern Data Management
In today's data-driven landscape, the relevance of data lineage is underscored by the complexity of data environments. Organizations are increasingly utilizing advanced technologies such as cloud computing, big data analytics, and artificial intelligence. These changes have resulted in heightened complexities in managing data. Therefore, effective data lineage practices are vital for sustainable data management.
A few key aspects showcase its relevance:
- Integration of Diverse Data Sources: Data often comes from a multitude of sources. Data lineage provides clarity on how these diverse sources come together and interact within a system.
- Evolving Data Usage: As businesses change, so does their data usage. Data lineage allows organizations to adapt their data management strategies based on current needs.
- Enhanced Collaboration: Understanding data flow promotes collaboration between teams by establishing a common understanding of data processes and governance strategies.
"With the flood of data available today, organizations that harness the power of data lineage can achieve a competitive edge through higher efficiency and better compliance."
Overview of IBM Data Lineage
IBM Data Lineage represents a vital function in the realm of data management, specifically tailored to support robust data governance strategies. Understanding the core aspects of IBM's data lineage tools is paramount for organizations as they navigate an increasingly complex data landscape. This overview aims to provide clarity on the key components and functionalities associated with IBM Data Lineage, highlighting its significance in enhancing data governance, compliance, and operational efficiency.
Core Features of IBM Data Lineage
IBM Data Lineage encompasses several key features that facilitate comprehensive understanding and traceability of data across its lifecycle. These features include:
- Data Path Visualization: This feature allows users to visualize data flow from origin to destination. It presents a clear pathway of data transformation, making it simpler to identify potential issues or inconsistencies that may arise during data processing.
- Automated Lineage Capture: With automated lineage capture, organizations can efficiently track data lineage without extensive manual labor. This feature not only saves time but also reduces the potential for human error.
- Impact Analysis: This capability helps in identifying how changes in one aspect of the data ecosystem can affect other components. It allows users to anticipate consequences and enables informed decision-making regarding data management.
- Integration with Governance Policies: IBM's lineage tools are designed to align with organizational governance policies, ensuring compliance with data regulations and standards. This integration is crucial for maintaining data integrity and security.
These core features collectively empower enterprises to maintain high data quality, enhance compliance efforts, and ultimately improve overall business intelligence.
Integration with IBM Products
IBM Data Lineage is designed to seamlessly integrate with various IBM products, enhancing the overall data management ecosystem. Some notable integrations include:
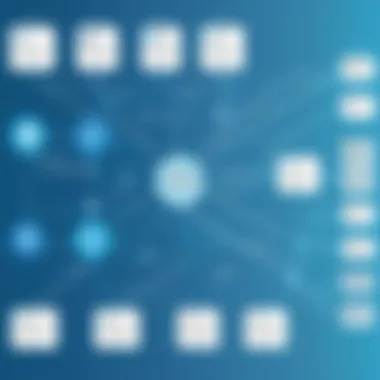
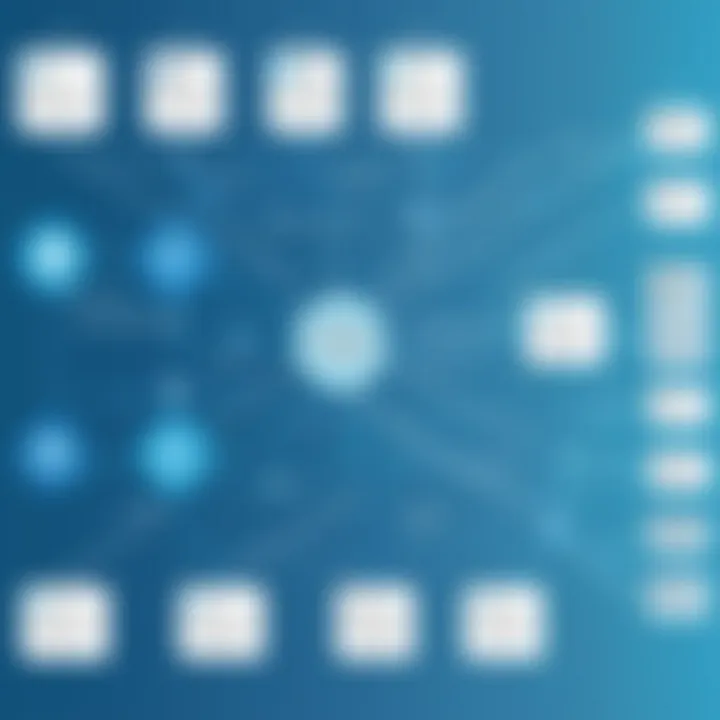
- IBM Watson Knowledge Catalog: This product allows for the effective cataloging of data and metadata, supporting data discoverability and usability. It connects with IBM Data Lineage to ensure that users have access to comprehensive lineage information when consulting data assets.
- IBM InfoSphere Information Governance Catalog: Integrating with this governance tool enables an organization to maintain a library of data lineage assets, promoting better data stewardship and compliance tracking.
- IBM Cloud Pak for Data: This integrated data and AI platform facilitates collaboration across teams and allows for systematic data management. The ability to leverage data lineage within this platform enhances the understanding of data flows and transformations, aiding in effective decision-making processes.
In summary, IBM Data Lineage establishes a robust framework that not only supports data governance but also promotes a deeper understanding of data transformation processes. As organizations work with complex data environments, effective data lineage becomes indispensable for maintaining accountability and ensuring optimal data quality.
Key Components of Data Lineage
Understanding the key components of data lineage is essential for organizations aiming to optimize their data management strategies. The intricate nature of data flow, coupled with transformation processes, affects decision-making and compliance with regulations. These components include data flow and transformation, metadata management, and data quality metrics.
Data Flow and Transformation
Data flow involves the path that data takes from its source through various processes until it reaches a final destination. Tracking this journey is crucial. To ensure accuracy, organizations must establish clear mapping of data flows. Each transformation, whether it is simple filtering or complex aggregations, needs documentation. This clarity fosters better understanding and governance.
When transformations happen, they can alter data meaning and usability. Hence, documenting these changes becomes vital. Effective tools can assist in visualizing these flows, making abstract processes comprehensible. Moreover, understanding where data originates helps in tracing back discrepancies, ensuring accountability within the data lifecycle. The key benefit of detailed data flow documentation is improved visibility into how data is transformed through its lifecycle.
Metadata Management
Metadata holds significant importance in data lineage as it provides context for understanding datasets. It can be considered as data about data. In managing large volumes of information, metadata clarifies the origin, structure, and usage of data. Thus, it becomes an essential component in tracking and governing data adequately.
Proper metadata management allows organizations to maintain an accurate inventory of their data assets. For instance, good metadata practices enable quick identification of data sources and lineage paths. Without effective metadata, organizations may struggle with data quality and compliance. Having a centralized metadata repository simplifies tracking and enhances accessibility and utility of data.
"Metadata is not just an aspect of data but a crucial enabler of effective data management and lineage tracing."
Data Quality Metrics
Data quality metrics gauge the reliability and accuracy of data throughout its lifecycle. This component is indispensable for enforcing a high standard of data integrity. Organizations can measure data quality through various dimensions such as completeness, consistency, and timeliness. Regular assessment of these factors ensures that data remains fit for purpose, which is critical for informed decision-making.
Establishing specific metrics provides a framework for evaluating the overall health of data. For example, measuring the percentage of accurate entries can help identify redundancies and errors. Techniques like data profiling can assist in comprehensively analyzing datasets. By implementing a robust set of metrics, organizations are better positioned to maintain trust in their data assets while satisfying compliance requirements.
Benefits of Implementing Data Lineage
Implementing data lineage brings significant advantages for organizations striving for effective data governance. It enables a systematic approach to understanding the flow of data throughout its lifecycle. This understanding is crucial for ensuring data quality and compliance in an increasingly complex data environment. The benefits of applying data lineage practices are multifaceted, impacting both operational efficiencies and strategic decision-making.
Enhanced Data Governance
Enhanced data governance is a primary benefit of data lineage. It allows organizations to monitor the flow of data across various systems, providing visibility into the origins and transformations of that data. This oversight encourages accountability. When data lineage is effectively utilized, organizations can maintain a clear understanding of who is responsible for data, how it is used, and the implications of any changes made.
It also aids in defining data ownership and usage policies. Clear roles and guidelines help mitigate risks related to data misuse. Moreover, robust data governance frameworks support compliance aims, ensuring that data practices align with industry standards and regulations.
Compliance and Regulatory Adherence
The second notable benefit becomes evident when considering compliance and regulatory adherence. In industries like finance and healthcare, strict regulations govern data handling and privacy. Implementing data lineage provides a structured view that ensures compliance with these laws. Organizations can easily trace data back to its source and document its handling processes. This documentation is valuable during audits and assessments, demonstrating the organization's commitment to regulatory standards.
Furthermore, the ability to visualize data changes and transformations makes it easier to identify non-compliance risks. Companies can quickly assess and rectify any issues before they escalate into larger problems. Thus, adherence to compliance not only safeguards legal standing but strengthens stakeholder trust.
Improved Data Discovery and Accessibility
Another significant aspect of data lineage implementation is improved data discovery and accessibility. When data lineage is documented, it becomes easier for users to understand where specific data resides and how it can be accessed. This clarity enhances decision-makers' ability to locate the necessary information quickly. In fast-paced business environments, having timely access to accurate data is paramount.
With robust data lineage, organizations can unlock the hidden value within their datasets. This increased accessibility promotes a data-driven culture, encouraging teams to leverage data in their daily operations. It also facilitates better collaboration among departments as they share insights based on accessible data sources.
Additionally, it helps in aligning data to business goals, ensuring that teams focus on the right data sets that have the most impact on strategic objectives.
Implementing data lineage is not just a technical upgrade; it is a strategic imperative.
Incorporating these elements into data management strategy enhances overall operational effectiveness. The journey toward improving data governance, ensuring compliance, and enhancing data accessibility through data lineage cultivates a more resilient organizational framework.
Challenges in Data Lineage Implementation
In the pursuit of implementing data lineage, organizations often confront a series of obstacles that may hinder their efforts. Understanding these challenges is crucial for navigating through the complexities tied to data management. The implementation phase demands not only technical abilities but also an awareness of the data environment as well as the resources required to facilitate effective data lineage practices. Addressing these challenges can lead to improved data quality, compliance, and overall governance.
Complexity of Data Environments
Data environments have become increasingly multifaceted. This complexity is primarily due to the diverse systems and technologies that organizations integrate into their operations. Each system might handle data differently, resulting in a myriad of data formats and structures. Generally, organizations possess data across various platforms, including on-premise databases and cloud services. This fragmentation poses significant challenges in establishing a unified understanding of data lineage.
For instance, unraveling the flow of data between systems requires deep technical knowledge and a clear mapping of how data moves through these systems. The more intricate the environment, the more difficult it becomes to ensure that data lineage is accurately tracked. Without the right tools, organizations may struggle to gain insights into data origins and transformations.
Integration Across Diverse Systems
Organizations often use various software solutions for different functions, creating silos of data that can be hard to connect. Integrating these diverse systems becomes a significant challenge. When data flows between these systems, it may lose its context or even be altered unintentionally, complicating the understanding of data lineage.
Effective integration is paramount for achieving a holistic view of data movement and transformation. Relying solely on manual processes can introduce errors, increasing the likelihood of misinterpretation. Automation plays a vital role in addressing this issue. Automated solutions can streamline the integration process, ensuring that data remains accurate and consistent across systems. However, organizations must invest time and resources to implement such solutions successfully.
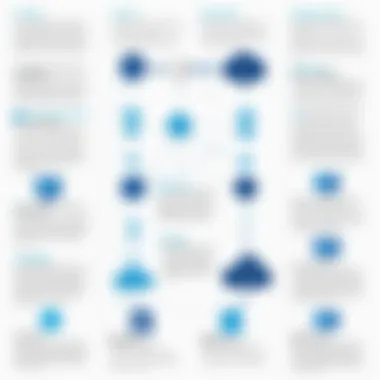
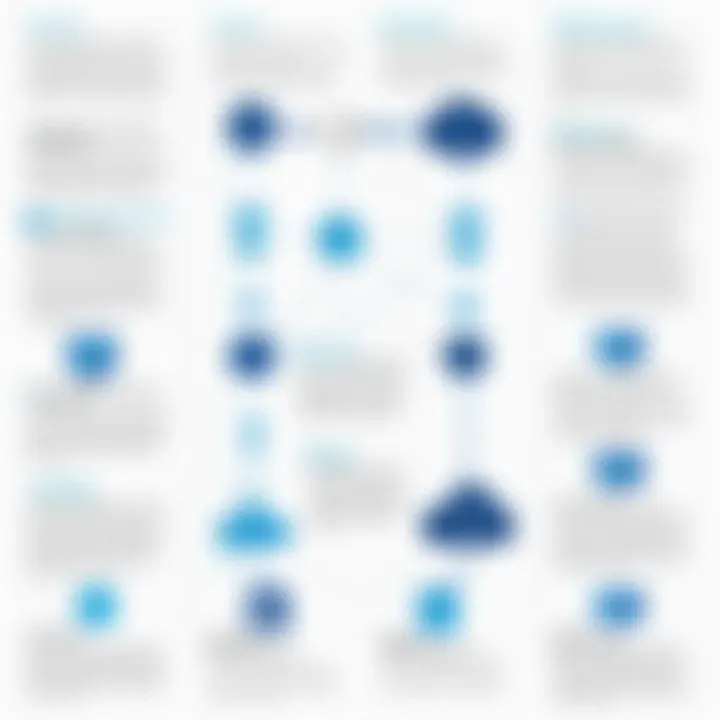
Resource Allocation and Expertise
Implementing effective data lineage requires adequate resource allocation and expertise. Organizations might face challenges in allocating sufficient technical resources to oversee data lineage initiatives. This involves hiring skilled professionals who understand both the technical aspects of data management and the regulatory landscape.
Furthermore, staff training is essential for fostering a culture of data awareness within an organization. Without a committed team equipped with the right skills, it becomes challenging to achieve successful data lineage implementation. Training existing staff or hiring new talent can be resource-intensive, requiring careful planning and management.
"Successful data lineage implementation hinges on a strategic approach to handling resources and expertise."
These challenges underscore the necessity for organizations to evaluate their current data management strategies. Recognizing and addressing these implementation challenges can ultimately lead to better data governance, compliance, and streamlined operations in the long run.
IBM Tools for Data Lineage Management
In the realm of data governance, the significance of proper tools for data lineage management cannot be overstated. These tools are essential for tracking data flow, ensuring compliance, and maintaining data quality. IBM offers a suite of tools tailored for this purpose. Each of these tools provides distinct features and advantages that address various aspects of data lineage management. Understanding their functionalities becomes crucial for organizations aiming to optimize their data processes and governance frameworks.
IBM Watson Knowledge Catalog
IBM Watson Knowledge Catalog is a robust solution designed for data governance and management. This tool allows organizations to catalog and organize their data assets effectively. By centralizing metadata, Watson Knowledge Catalog enhances visibility into data lineage.
Key features of this tool include:
- Automated Data Classification: Helps categorize data assets intelligently, making it easier to manage and locate.
- Collaboration and Sharing: Enables teams to work together on data assets, promoting a culture of collaborative data management.
- Business Glossary: Provides a comprehensive glossary that enriches data understanding across the organization.
Using this tool, organizations can improve data discoverability and enhance the trustworthiness of their data assets, essential for decision-making and analytics.
IBM InfoSphere Information Governance Catalog
IBM InfoSphere Information Governance Catalog serves as another key player in data lineage management. This catalog focuses on governance processes, allowing users to define and manage business terms and policies related to data. It emphasizes compliance and regulatory requirements.
Critical elements of the InfoSphere include:
- Data Lineage Visualization: Graphical representations of data flows help users understand where data originates and how it transforms.
- Policy Management: Establishes data policies that ensure compliance with internal and external regulations.
- Governance Frameworks: Supports the creation of governance frameworks tailored to the specific needs of an organization.
This tool is especially valuable in highly regulated industries where adherence to policies is imperative.
IBM Cloud Pak for Data
IBM Cloud Pak for Data is an integrated data and AI platform that streamlines the data lineage process. It delivers a comprehensive suite of tools that facilitate data integration, management, and analysis. The capabilities of this platform extend towards enabling effective data lineage practices.
Features of IBM Cloud Pak for Data include:
- Unified Data Environment: Offers a cohesive environment for data processing, analysis, and visualizations.
- AI-Driven Insights: Leverages artificial intelligence to extract insights from data, ensuring informed decision-making.
- Security and Compliance: Implements robust security measures and compliance checks throughout the data lifecycle.
Through these features, IBM Cloud Pak for Data not only simplifies data lineage tracking but also enhances overall data governance in a cloud-native environment.
"Effective data lineage management relies heavily on the right set of tools to ensure visibility and control over data assets."
Best Practices for Utilizing Data Lineage
Data lineage is essential for any organization that wants to manage its data effectively. Utilizing data lineage in the right way can lead to multiple benefits. Among these include enhanced visibility into data flow, better compliance strategies, and improved data governance. To achieve these benefits, organizations should adhere to best practices when implementing data lineage solutions. This section delves into some of those practices, helping organizations navigate the complexities inherent in data lineage.
Establishing Clear Objectives
When beginning any data lineage initiative, clarity in objectives is paramount. Organizations should define what they want to achieve through data lineage. Specific objectives could range from improving data quality to ensuring compliance with regulations, such as GDPR or HIPAA. By establishing clear objectives, teams can align their efforts and focus on key tasks. Without well-defined goals, there is a risk of misallocating resources or overlooking critical areas.
For effective alignment, organizations should ask:
- What specific issues are we trying to address?
- How will data lineage support our overall business strategy?
- What metrics will we use to measure success?
Continuous Monitoring and Assessment
Data environments are dynamic, which means the processes supporting data lineage need regular review. Continuous monitoring ensures that data flows are accurately recorded and aligned with the established objectives.
Organizations should adopt a cycle of constant assessment. This may involve auditing lineage data, evaluating data quality metrics, and re-assessing compliance standards. By doing so, it is easier to identify areas for improvement. A robust monitoring plan helps in proactively addressing issues before they escalate.
Key elements of a continuous monitoring plan include:
- Regular audits of data sources
- Feedback loops with business units
- Adapting to changes in data regulations
Stakeholder Engagement and Training
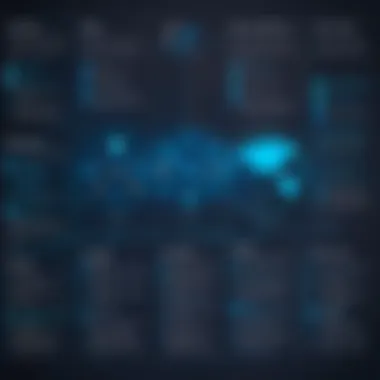
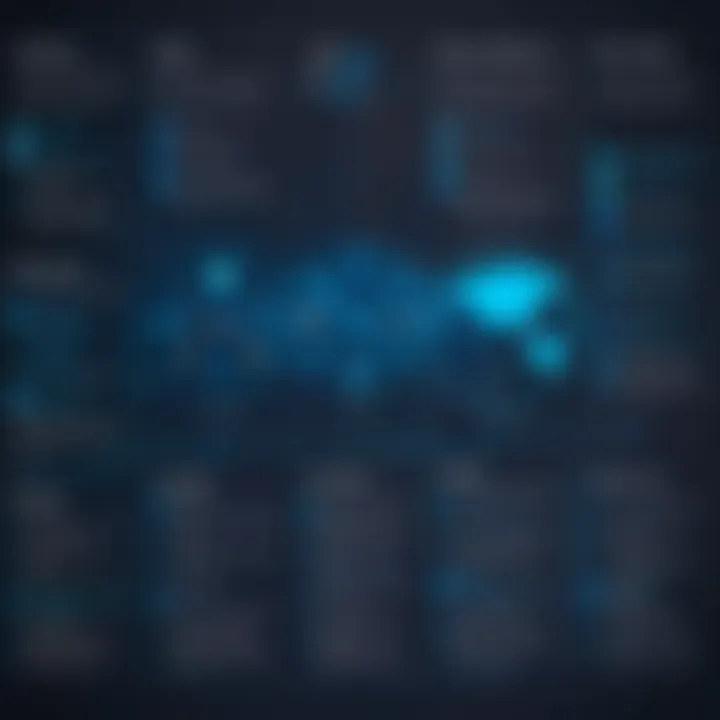
It is essential to involve stakeholders at all levels when it comes to data lineage initiatives. Engaging stakeholders leads to better support for data governance efforts. This support can stem from departments like IT, legal, and business operations. Training in data lineage concepts is equally crucial. Employees need to understand not just how to use data lineage tools but why their usage matters.
Organizations should develop a training program that covers data lineage basics, tools available, and their specific applications in day-to-day operations. Engaged and well-informed stakeholders contribute to a culture that prioritizes data quality and governance.
Organizations might consider:
- Regular training sessions on new tools
- Workshops focused on best practices
- Creating an internal forum for knowledge sharing
Engaging stakeholders while continuously training them sets a foundation that strengthens the entire data lineage approach.
Case Studies on Effective Data Lineage
Examining case studies on effective data lineage offers valuable insights into how organizations have successfully implemented data lineage practices. These practical examples reveal the relevance, benefits, and challenges encountered during the implementation process. Case studies serve as a form of proof for the effectiveness of data lineage in achieving improved data governance, compliance, and organizational efficiency.
Industry-Specific Implementations
Different industries face unique challenges and requirements when it comes to data management. Case studies illustrate how various sectors leverage IBM Data Lineage to address their specific needs. For instance, in the financial sector, regulatory requirements necessitate meticulous tracking of data movement. One bank implemented IBM's data lineage tools to enhance transparency and compliance. By mapping the flow of transactions from initiation to reporting, they significantly improved their ability to meet stringent regulations.
In the healthcare industry, managing patient data integrity is critical. A healthcare organization adopted IBM Data Lineage to maintain accurate records across multiple systems. The implementation allowed them to trace patient data back to its source, ensuring data accuracy and compliance with healthcare regulations like HIPAA. This case highlights how effective data lineage practices can lead to improved patient care and regulatory adherence.
Lessons Learned and Best Practices
Through these case studies, several lessons and best practices emerged:
- Clear Objectives: Organizations should define specific goals for their data lineage initiatives. A banking institution realized that having targeted goals helped them focus their efforts and resources more effectively.
- Engaging Stakeholders: Involving key stakeholders, from IT teams to business units, ensures buy-in and a better understanding of the processes. A manufacturing firm showed improvements by involving departments in the data lineage mapping.
- Regular Assessments: Continuous monitoring of the data lineage process is essential. An energy company employed routine assessments to identify gaps in data flow, leading to timely corrections.
- Utilizing Automation: Automation can accelerate the data lineage mapping process. Using tools from IBM, a retail company streamlined their data processes, reducing resource allocation significantly.
"Implementing data lineage provides organizations with a clearer view of their data landscape, which ultimately leads to better decision-making and compliance management."
Through these insights, it becomes evident that effective data lineage is more than a destination; it is an ongoing journey that requires continuous improvement and adaptation to evolving data landscapes. By carefully analyzing these case studies, organizations can better understand how to effectively implement data lineage tailored to their specific contexts.
Future Trends in Data Lineage
The landscape of data lineage is continually evolving. As organizations leverage large volumes of data, understanding trends becomes vital for effective data management. Future trends in data lineage provide insights into how companies can better govern their data, ensure compliance, and improve overall data quality. These trends are influenced by technological advancements and the increasing focus on data regulations.
The Role of Artificial Intelligence
Artificial intelligence (AI) is poised to revolutionize data lineage practices. By utilizing machine learning algorithms, organizations can automatically trace data lineage. This not only speeds up the tracking process but also reduces human errors. AI can help in recognizing patterns and anomalies in data flows that are often overlooked.
Moreover, AI can enhance metadata management by automatically generating metadata. It can classify data sources and suggest lineage paths based on learned behavior. This evolution reduces the burden on data governance teams and provides a more accurate picture of data movement and transformation.
Greater Emphasis on Automation
Automation is becoming a central theme in data management. The increasing complexity of data environments demands efficient methods for monitoring data lineage. Automated systems can help in continuously tracking data flows, providing real-time insights.
Benefits of automation include:
- Improved Efficiency: Reduces time spent on manual tracking.
- Increased Accuracy: Minimizes human error in lineage documentation.
- Real-time Monitoring: Enables quicker identification of data issues or discrepancies.
With robust automation tools, organizations can enhance compliance efforts and streamline access to data lineage information, fostering a culture of transparency and accountability within data governance.
Evolving Compliance Requirements
The regulatory environment is becoming more stringent. Businesses must navigate complex compliance frameworks that require a clear understanding of data lineages, such as GDPR or HIPAA. As regulations evolve, having comprehensive data lineage capabilities is no longer optional. It is essential for demonstrating accountability.
Organizations need to ensure they have ways to easily report on data flow and transformations to comply with mandates. Failure to adapt could lead to significant penalties. Future compliance requirements will likely focus more on data provenanceโknowing where the data originated and how it was altered throughout its lifecycle.
"Understanding how to leverage these future trends is key to optimizing data management strategies."
Finale
The conclusion serves as the essential closure of this article, reinforcing the significant insights gained regarding IBM Data Lineage. Data lineage is more than a mere technical concept; it embodies the framework through which organizations can understand and optimize their data. Within IBMโs ecosystem, this becomes a formidable asset in ensuring effective data governance, enhancing compliance, and increasing overall data quality.
Summarizing Insights on Data Lineage
In summary, the implementation of data lineage practices, particularly with IBM's tools, leads organizations to substantial advantages. Key insights revealed include:
- Visibility: Data lineage offers clarity in data movement and transformation, allowing professionals to pinpoint data origins and understand its journey through various systems.
- Assessment: Continuous observation facilitates the identification of data quality issues, enabling timely corrections that enhance reliability.
- Governance: A robust lineage strategy ensures adherence to regulations and standards, thus mitigating risks associated with data misuse.
Moreover, adopting these practices cultivates a culture of accountability in data management. The interconnection between data flow and its consequences on business intelligence cannot be overstated, making data lineage a critical focus for modern organizations.
Call to Action for Implementation
For IT professionals and business leaders looking to harness the full potential of their data, acting upon the insights provided is crucial. Here are a few recommended steps to consider:
- Assess Current Systems: Evaluate the existing data landscape to identify gaps in visibility and control.
- Invest in Tools: Implement solutions like IBM Watson Knowledge Catalog or IBM InfoSphere Information Governance Catalog which provide advanced data lineage capabilities.
- Educate Teams: Promote training on data lineage concepts within the organization to ensure all team members understand its importance and functionality.
- Establish Goals: Define clear objectives supporting data lineage initiatives, thereby aligning these goals with broader organizational strategies.
- Monitor Progress: Regularly review the effectiveness of implemented practices to ensure continuous improvement and adaptation to evolving data environments.
The time to embrace data lineage is now. With the right approach, organizations will not only enhance their data management practices but will also empower informed decision-making across all levels of operation.