Understanding Jump Statistical Software: A Guide
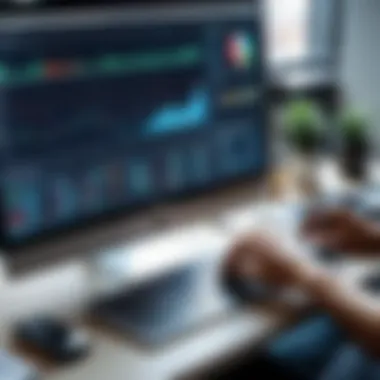
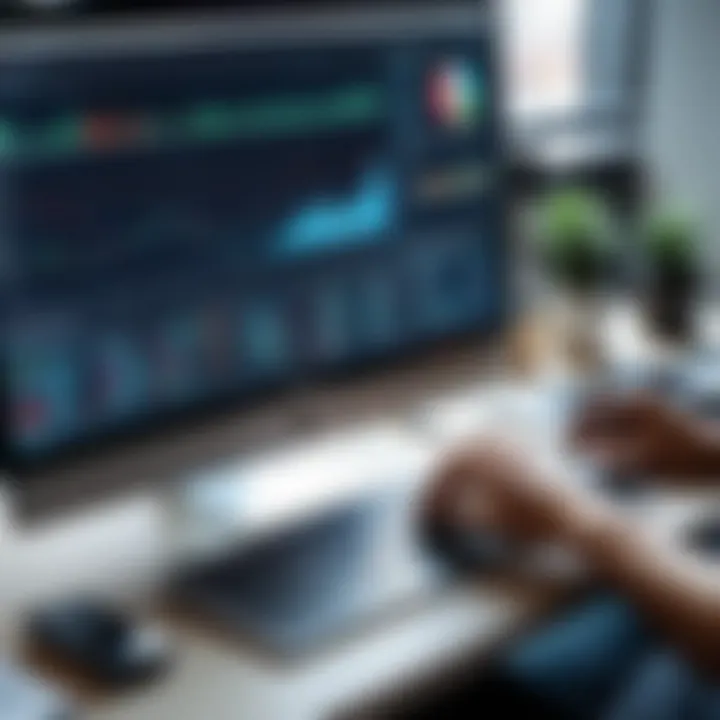
Intro
Jump Statistical Software, developed by SAS Institute, is a powerful tool for data analysis and visualization. It is widely used in various fields such as academia, industry, and research institutions. As the demand for data-driven decision-making grows, understanding the capabilities of software like Jump becomes essential for professionals aiming to leverage statistical tools effectively. This article will explore its functionalities, highlighting how Jump can meet diverse analytical needs, and providing insights into its practical applications.
Software Overview
Software category and its purpose
Jump belongs to a category of statistical software designed to facilitate data exploration, analysis, and visualization. Its primary purpose is to provide an interactive environment where users can analyze data visually and perform complex statistical tests without requiring extensive programming knowledge. This makes it suitable for a broad range of users, from novice analysts to seasoned researchers.
Key features and functionalities
Jump offers an array of features that cater to various analytical tasks:
- User-friendly interface: The software employs a drag-and-drop interface, making it accessible for users with different skill levels.
- Data visualization: Built-in tools allow for quick generation of graphs, plots, and charts to represent data visually.
- Statistical modeling: Jump supports a wide range of statistical techniques such as regression, ANOVA, and multivariate analysis.
- Customizable reports: Users can create reports that integrate analysis results, providing a comprehensive view of findings.
- Scripting capabilities: For advanced users, Jump provides the option to write scripts for custom analyses.
These features combined make Jump a versatile tool that enhances data analysis workflows and supports informed decision-making.
Comparative Analysis
Comparison with similar software products
When comparing Jump with other statistical software like R and SPSS, notable differences emerge. R is open-source and offers extensive packages for various analyses, but it requires coding skills. SPSS, on the other hand, is also user-friendly, yet it can be more restrictive in flexibility. Jump strikes a balance between ease of use and robust functionality.
Market positioning and user demographics
Jump is positioned primarily towards professionals in data analytics, including IT experts, researchers, and business analysts. Its appeal lies in its ability to simplify complex analyses while offering sufficient depth for detailed investigations. Many users appreciate its visually-oriented approach, which aids in understanding complex data relationships efficiently.
Preamble to Jump Statistical Software
Jump Statistical Software plays a vital role in the realm of statistical analysis and data visualization. Its significance arises from the ability to process complex data sets efficiently. For researchers, IT professionals, and business analysts, Jump offers a user-friendly interface combined with powerful statistical capabilities that enhance data interpretation and decision making.
The growing demand for data-driven insights in today’s world necessitates a tool that is both accessible and robust. With its comprehensive features, Jump is designed to cater to diverse analytical needs. The software allows users to explore data deeply, facilitating connections between datasets and identifying crucial trends.
Before delving into its core features, it is essential to understand the history and the primary purpose of Jump. This will provide context to the software’s applications and show how it has evolved over time to meet the challenges of modern data analysis.
Background and History
Jump was developed by SAS Institute Inc., founded in the early 1980s. Initially, the software aimed to simplify statistical analysis for various fields, including engineering, quality control, and educational research. Over the years, Jump has expanded its capabilities significantly, integrating advanced algorithms and enhanced visualization options. By maintaining a strong focus on user experience, the software has established a reputation for being intuitive and effective in handling statistical challenges.
The evolution of Jump reflects the broader trends in data science. As organizations increasingly rely on data for strategic decisions, Jump has adapted to incorporate new methodologies and technologies. This historical perspective helps users appreciate the thoughtful design and ongoing enhancements that characterize Jump today.
Purpose and Scope of the Software
Jump serves to bridge the gap between raw data and actionable insights. Its primary purpose is to provide a platform where users can conduct comprehensive statistical analyses without needing extensive programming knowledge. The user-centric design focuses on fostering an exploratory data analysis environment, encouraging users to interact with their data creatively.
The scope of the software extends across multiple industries, including academia, healthcare, and business analytics. Jump allows users to:
- Perform complex statistical procedures with ease.
- Create visually stunning data presentations.
- Collaborate with teams using cloud-based functionalities.
- Utilize advanced analytics features for more profound insights.
By integrating these capabilities, Jump not only simplifies the analytical process but also maximizes the potential for discovering patterns and trends within data. Thus, the importance of understanding Jump Statistical Software cannot be overstated. Recognizing its features paves the way for effective application in various professional contexts.
"Understanding the tools available is key to making data-informed decisions."
As we proceed through the sections, this comprehensive guide will outline core features, industry applications, and best practices associated with Jump, providing valuable insights for professionals looking to harness its full potential.
Core Features of Jump
The core features of Jump Statistical Software serve as the backbone of its functionality. These features define how users interact with the software and the specific tasks they can perform. Understanding them is crucial for any professional aiming to leverage advanced analytical tools in their work. They not only streamline the analytical process but also enhance the overall user experience. This section will cover three significant aspects of Jump: data import and management, statistical analysis capabilities, and visualizations and reports. Each of these features plays a pivotal role in facilitating efficient data analysis.
Data Import and Management
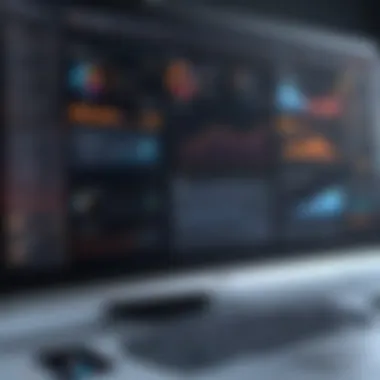
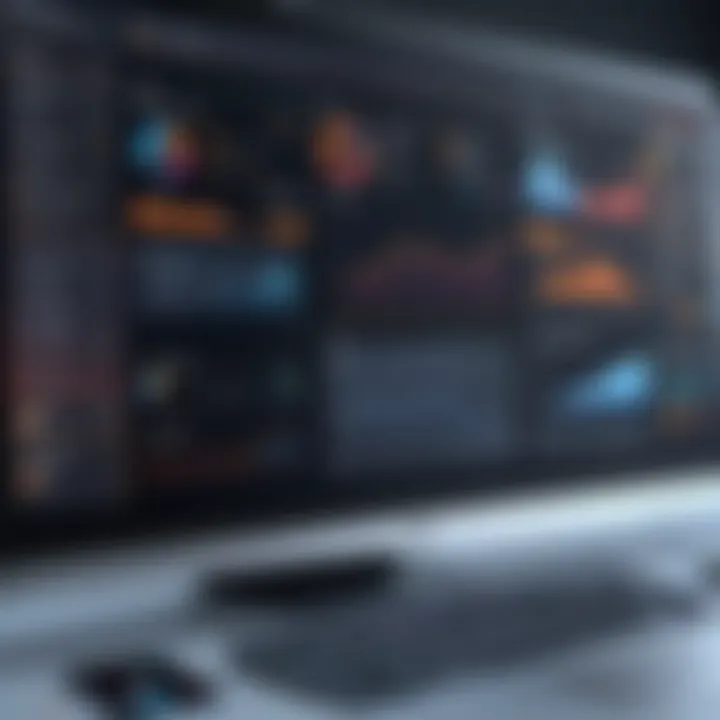
Data import and management are essential aspects of any statistical software. Jump excels in this area by supporting a wide variety of data formats. Users can easily import data from Excel spreadsheets, SAS datasets, and databases like SQL. This broad compatibility reduces the barriers to data entry and significantly speeds up the initial phases of analysis.
Once the data is imported, Jump offers robust management tools. Users can organize, clean, and manipulate their datasets within the software. Data filtering, sorting, and transformations are straightforward, giving users the power to prepare their data without needing extensive programming skills. This capability is particularly beneficial for professionals in fast-paced environments, where time is often a critical factor. Additionally, the software provides a user-friendly interface that minimizes the learning curve for those less experienced with statistical tools.
Statistical Analysis Capabilities
Jump's statistical analysis capabilities are among its strongest features. The software supports a wide range of statistical tests and methodologies, from basic descriptive statistics to complex predictive modeling. Users have access to methods such as regression analysis, ANOVA, and multivariate techniques, catering to the diverse needs of both researchers and business analysts.
What sets Jump apart is its interactive and intuitive design, allowing users to explore results dynamically. This is particularly important for business professionals who need to quickly assess the implications of their data. The software also features scripting capabilities, enabling advanced users to customize their analyses. This blend of ease of use and advanced functionality makes Jump a valuable tool for any statistical analysis.
Visualizations and Reports
Visualizations play a critical role in data analysis by turning numbers into comprehensible visual formats. Jump offers a rich suite of visualization tools, enabling users to create high-quality graphs, charts, and dashboards. The visuals are not just aesthetically pleasing; they are designed to convey insights clearly and effectively. Users can easily customize visual outputs to meet specific presentation needs.
Beyond standard graphics, Jump allows users to create tailored reports. These reports can be generated directly from the analysis output, making it simple to share findings with stakeholders. The ability to export reports in various formats enhances usability, especially in professional settings where presenting data is key.
Applications Across Industries
The relevance of Jump Statistical Software in various industries cannot be overstated. This software provides valuable tools suited to the needs of different sectors, enabling effective analysis, interpretation, and presentation of data. The ability to adapt to diverse environments ensures its position as a key player in data analysis and statistical applications. In this section, we will examine its specific contributions to academic institutions, business analytics, and the healthcare sector.
Academic and Research Institutions
In academic and research settings, Jump serves as a versatile tool for statisticians, researchers, and educators. One of its primary benefits is the ease of use when it comes to data analysis. Students and faculty can efficiently conduct statistical tests, whether for simple experiments or complex studies. The intuitiveness of the user interface allows for quick learning and exploration of sophisticated features, minimizing the transition period from theory to practical application.
Key functions include:
- Statistical tests: Traditional tests like t-tests and ANOVA, alongside modern methods like mixed models.
- Data management: Clean up, restructure, and analyze data quickly, facilitating exploratory data analysis.
- Visualization tools: Students and researchers can create plots and graphs to visualize results, enhancing presentations and reports.
Jump also supports collaboration among peers across institutions. Researchers can share analyses and findings in a coherent format, fostering an environment of peer review and feedback.
Business Analytics and Market Research
When it comes to business analytics, Jump Statistical Software equips organizations with tools to make data-driven decisions. The power of advanced statistical analysis lies in its ability to process large datasets, uncover trends, and help administrators dedicate resources efficiently. By employing Jump, businesses can derive significant insights from their operations.
In market research, the software allows companies to analyze consumer behavior and preferences. The ability to generate detailed reports helps in refining marketing strategies and product development. Some essential features for businesses include:
- Predictive analytics: Anticipate consumer demand and improve inventory management.
- Customer segmentation: Use clustering techniques to identify different market segments for personalized marketing efforts.
- Competitive analysis: Benchmark performance against industry competitors to identify strengths and weaknesses.
Through these applications, Jump provides businesses with a comprehensive platform for making informed strategic choices.
Healthcare and Clinical Research
In the healthcare sector, Jump plays a critical role in clinical research. Researchers can analyze health data to study disease patterns, treatment outcomes, and patient demographics. The insights gained from such analyses are crucial for developing interventions and improving patient care.
Healthcare applications of Jump include:
- Clinical trials: Analyze data from trials to ensure the efficacy and safety of new treatments before they reach the market.
- Epidemiology studies: Investigate and quantify the incidence of diseases, shedding light on potential causes and risk factors.
- Patient data analysis: Aggregate and interpret vast quantities of patient data, facilitating personalized medicine approaches.
Jump's ability to manage healthcare data also helps organizations comply with regulations and standards, ensuring data integrity and confidentiality.
"Jump Statistical Software provides a robust platform for analyzing data across various sectors, proving its adaptability and essential role in data analysis."
Comparative Analysis with Other Statistical Software
A comparative analysis of Jump Statistical Software relative to its competitors serves multiple purposes. It assists potential users in comprehending the distinct features, workflows, and overall value of Jump against other statistical tools. Given the evolution of data analysis needs, it's crucial to understand how different software packages can meet or falter in meeting those requirements. Evaluating Jump in a broader context illuminates its suitability for specific analytical tasks, guiding users toward informed choices.
Key Competitors Overview
Jump is not alone in the statistical software landscape. Multiple platforms vie for market share, each with unique offerings. Among these, notable competitors include:
- SAS: Renowned for its advanced analytics capabilities, SAS is often used in large organizations that require extensive data manipulation and analysis.
- SPSS: A popular choice in social sciences, SPSS features user-friendly interfaces and powerful statistical functions, catering to researchers and students alike.
- R: An open-source environment, R offers flexibility through its vast package ecosystem. It is favored by statisticians and data scientists for custom analyses.
- Python with Pandas and SciPy: Although Python is a programming language, its libraries have made it a powerful statistical tool, integrating data analysis with data science workflows.
This overview reveals a crowded market, but each product has its strengths that suit distinct purposes.
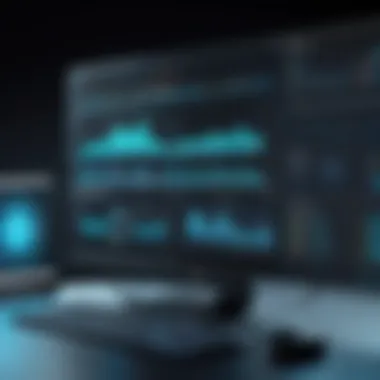
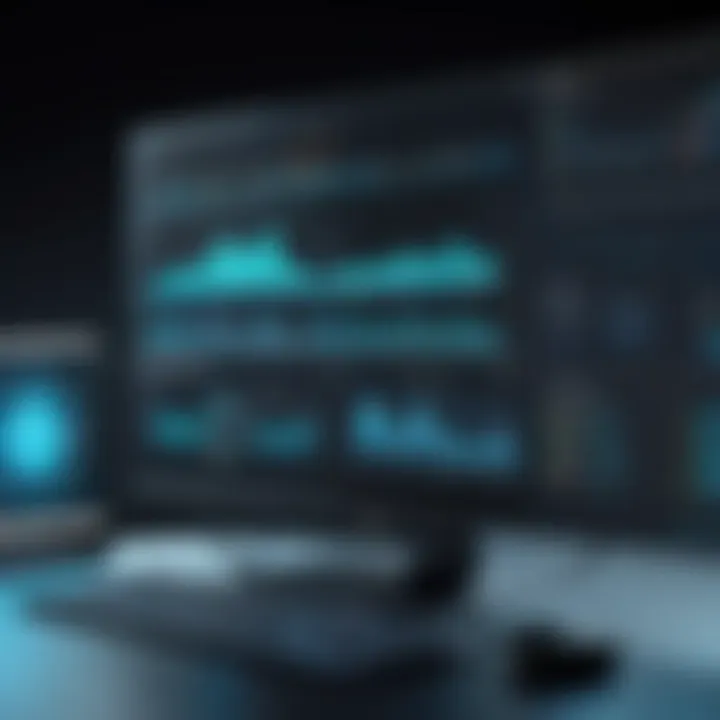
Strengths of Jump Over Competitors
Jump’s strengths emerge from its user-friendly interface, robust visualizations, and dynamic data manipulation capabilities.
- Interactive Data Exploration: Unlike many competitors, Jump allows users to interactively explore data, facilitating a more intuitive understanding of underlying patterns and relationships.
- Visual Analysis Capabilities: Jump's emphasis on visual analytics helps users to see the results of their analyses in immediate and comprehensible formats. This contrasts with some competitors, where data visualization may require additional effort or steps.
- Simplicity of Use: The learning curve for Jump is generally less steep compared to SAS or R. Non-technical users can achieve significant analytical results more quickly, a factor that can be crucial in time-sensitive environments.
- Integration Options: Jump enables users to integrate with other data sources and tools, enhancing its utility across different platforms and software ecosystems.
These advantages position Jump as a strategic option for teams that value efficiency and accessibility in statistical analysis.
Limitations and Areas for Improvement
Despite its strengths, Jump is not without limitations. Understanding these areas is essential for making an informed decision:
- Cost: Jump’s licensing can be prohibitively expensive for smaller organizations or individual users, potentially limiting its audience.
- Programming Capabilities: Jump lacks the deep programming capabilities present in tools like R or Python. This restricts advanced users who may seek to perform highly customized analyses or automate complex workflows.
- Community Support: While Jump has an active user base, its community is smaller compared to those of R or Python, potentially diminishing the availability of third-party resources and forums for troubleshooting.
- Advanced Statistical Techniques: For some specialized statistical techniques, Jump may not offer the breadth of features found in more established competitors.
These considerations should provide potential users with a clearer picture of where Jump excels and where it may fall short in comparison to other statistical software.
Best Practices for Using Jump
Utilizing Jump Statistical Software effectively requires understanding its best practices. This knowledge not only enhances the user experience but also maximizes the software’s capacities. Implementing these best practices ensures that you extract the most accurate insights from your data analysis. By adhering to established methods, users can streamline their workflows, reduce errors, and improve the quality of their outputs.
Effective Data Preparation
Data preparation is critical for any statistical analysis. When working with Jump, the initial groundwork lays the foundation for valid results. It involves assessing and formatting data before analysis. Users should focus on cleaning the data, which can involve removing duplicate entries and handling missing values appropriately. Having a well-structured dataset ensures that subsequent analyses yield reliable conclusions.
Ensuring the correct data types for each variable is also vital. Jump allows easy manipulation of datasets, which means taking advantage of the software's data management tools is essential. Moreover, keeping organized folders or projects can provide clarity during analysis. Maintain a clear naming convention for datasets and files, so that it is easy to locate them later.
Interpreting Output and Results
After running analyses, understanding the outputs is crucial. Jump provides a variety of visualizations and statistical outputs, which require careful interpretation. First, users should familiarize themselves with output types, ranging from summary statistics to hypothesis testing results. Recognizing the significance levels and confidence intervals is key to making informed decisions.
Utilizing Jump's graphical capabilities can also enhance interpretation. Graphs and plots provide a visual aspect that often clarifies complex data. Users should ensure they understand how to customize these graphics to convey the right message. Additionally, documenting findings as you interpret them promotes better communication with stakeholders and team members.
Key Point: A thorough understanding of output improves decision-making accuracy in data-driven contexts.
Integrating Jump with Other Tools
Jump can be integrated with various data analysis and visualization tools. Effective integration enhances overall analytical capabilities. For example, exporting outputs to software like Microsoft Excel or Tableau allows for more advanced reporting features. Similarly, jumping data between applications aids in smooth workflow transitions.
Understanding the import and export functionalities within Jump is necessary for this integration. It can reduce time wasted on data entry and formatting. Users should also look into APIs and other plugins that could enhance Jump’s functionality. For IT professionals, this can be an area to explore for productivity enhancement and automation.
In summary, mastering these best practices in using Jump will not only lead to more accurate results but also facilitate a more efficient analytical process. As users become adept at data preparation, output interpretation, and tool integration, they will find that Jump provides a robust platform well-suited for various analytical tasks.
User Experiences and Case Studies
User experiences and case studies provide a critical lens through which the practical effectiveness of Jump Statistical Software can be assessed. Real-world applications and user feedback not only illustrate how the software functions in various environments, but they also highlight its strengths and limitations. By examining this section, readers can gain insights into how Jump adapts to specific analytical tasks and the satisfaction level among its users.
Real-World Applications
Jump has been utilized across a range of industries, which confirms its versatility and robustness. In academics, for instance, researchers frequently apply Jump for complex survey data analysis, simplifying intricate statistical procedures into accessible outputs. This feature becomes particularly useful for academic institutions where time and resources are often constrained.
In business analytics, companies like Procter & Gamble leverage Jump for market research and customer satisfaction studies. Its ability to quickly manipulate large datasets helps organizations make informed decisions based on actionable insights.
Moreover, in healthcare, Jump plays a pivotal role in clinical trials. Pharmaceutical companies utilize Jump to analyze patient data, ensuring that findings are both effective and compliant with regulatory standards. These varied examples underline the software's applicability in diverse environments, showcasing its adaptability and efficacy.
Feedback from the User Community
The feedback from users serves as a valuable resource, guiding potential new users in their software selection process. Many communities, such as those found on platforms like Reddit or specialized forums, offer candid reviews about their experiences with Jump. Users often commend the intuitive interface and the quality of visualizations, noting that these features significantly enhance their analytical capabilities.
However, some users have pointed out areas for improvement. For instance, while Jump supports extensive functionality, aspects like customization of reports are sometimes limited. This feedback is crucial, as it helps developers prioritize enhancements.
Considerations for Software Selection
When organizations consider acquiring statistical software, several key aspects must be analyzed to ensure that the choice aligns with their specific needs. First, understanding the unique requirements can help narrow down options and facilitate a more efficient selection process. Also, it is vital to assess both the short-term and long-term goals of using the software. This helps in determining how the software will contribute to the organization’s overall objectives and productivity.
Assessing Organizational Needs
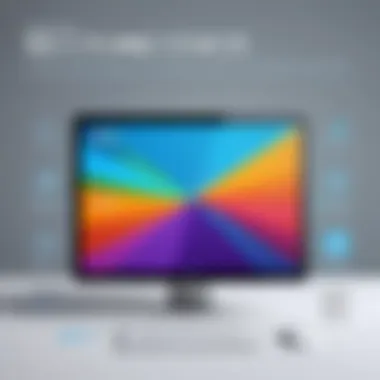
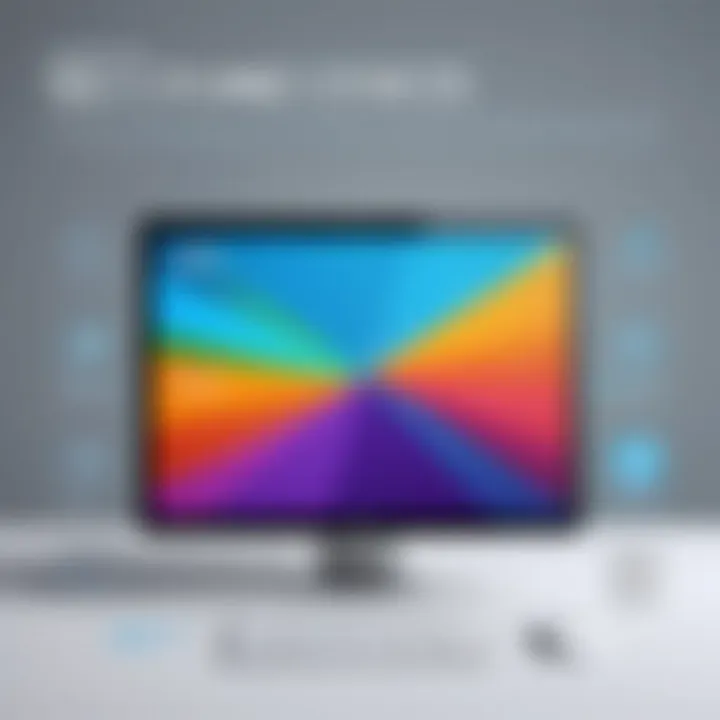
Assessing organizational needs is the foundation of choosing statistical software. Different companies might have various analytical requirements based on their industry, size, and overall data strategy. For example, a healthcare institution may prioritize robust predictive modeling tools, while a marketing firm might focus more on data visualization features.
To accurately evaluate needs, stakeholders should gather input from relevant departments. Conducting surveys or interviews with end-users provides insight into what capabilities they find most valuable. Furthermore, analyze existing workflows to identify gaps where Jump software can provide improvements. This tailored approach leads to a more effective selection process, ensuring the software directly benefits the organization.
Budgeting and Cost Analysis
Budgeting is another critical piece of the software selection framework. Potential users should consider not only the software's initial purchase price but also ongoing costs associated with licenses, updates, and potential additional modules. A thorough cost analysis can help the organization understand these factors and prepare financially.
Organizations should evaluate total cost of ownership, which may include:
- License fees: One-time or subscription-based charges.
- Training and onboarding: Costs involved in ensuring staff are capable of using the software efficiently.
- Maintenance and support: Any ongoing assistance required from vendors for smooth operation.
By addressing these financial aspects upfront, decision-makers can avoid unexpected expenses later and ensure they choose a solution that is sustainable.
Vendor Support and Documentation
The level of vendor support is critical when implementing any software solution. A responsive vendor can make a significant difference in the overall user experience. Users should consider:
- Availability of technical support: Ensure that the vendor provides timely assistance, particularly during critical analysis periods.
- Quality of documentation: Comprehensive manuals or online resources can empower users to troubleshoot and utilize features efficiently.
- Training resources: If the vendor provides workshops or online tutorials, this can facilitate a smoother onboarding process for users.
Understanding these elements can greatly enhance the effectiveness of the software, reducing frustrations and improving the overall user experience.
Future Trends in Statistical Analysis Software
The landscape of statistical analysis software is continually evolving. As organizations rely more on data to drive decisions, understanding these trends is essential. Future trends in statistical analysis software not only shape the functionalities delivered to users but also influence how analysts and data scientists approach their tasks. This article will focus on critical advancements, particularly in machine learning and user interface enhancements. Both elements contribute significantly to leveraging Jump Statistical Software effectively.
Adoption of Machine Learning
The integration of machine learning into statistical analysis software represents a seismic shift in data analytics. This trend is crucial, as organizations seek to transform vast amounts of raw data into actionable insights. Machine learning algorithms allow for advanced predictive analysis, automating the detection of patterns that may go unnoticed in traditional analyses.
Jump is adopting these capabilities through several avenues:
- Predictive Modeling: Users can now create models that predict future outcomes based on historical data. This feature dramatically increases a user's ability to make informed decisions.
- Automated Data Processing: Implementing machine learning can streamline tasks that require extensive data cleansing and processing. This reduces the time analysts spend on repetitive tasks.
- Scoring and Clustering: Machine learning techniques such as classification and clustering allow users to segment datasets, discovering underlying structures which can lead to more targeted analyses.
With these features, Jump enhances its relevance in sectors like finance, marketing, and healthcare. It also caters to the growing demand for non-technical users to harness data analytics more effectively. The software's machine learning functionalities therefore hold a promising future, ensuring it can meet evolving analytical needs.
Enhancements in User Interface and Accessibility
User experience is becoming increasingly important in statistical software. As users range from domain experts to those with less technical background, the push for a more intuitive interface is vital. Enhancements in user interface and accessibility are transforming how users interact with Jump Statistical Software.
- Improved Navigation: Developers are focusing on simplifying navigation. Clear layouts and intuitive guide systems ensure users can find the features they need without frustration.
- Customizable Dashboards: Customizability allows users to prioritize the display of information that is most relevant to their specific needs, which can enhance user satisfaction.
- Accessibility Features: Addressing inclusivity, Jump is implementing features that accommodate a broader array of users, including those with disabilities. This can include text-to-speech functions or keyboard navigation options.
The enhancements in user interface and accessibility not only make Jump more user-friendly but also enhance its adoption across various sectors. As more users, both seasoned analysts and novices, engage with the software, providing a seamless experience has become paramount.
Important Note: Keeping an eye on these trends not only prepares organizations for future challenges but also positions them advantageously in the data-driven economy.
Finale
The conclusion section holds significant weight in this article as it encapsulates the essence of Jump Statistical Software’s relevance to a modern analytical landscape. Summarizing the key insights shared throughout this guide provides readers with a clear understanding of how Jump stands out among its contemporaries and fulfills various analytical need.
Jump Statistical Software is not merely a tool for statistical analysis; it has evolved into an integral part of decision-making processes across multiple sectors. By summarizing the core features, practical applications, and comparative analysis, the conclusion articulates the necessity of integrating such a powerful statistical software into one's technical repertoire.
Moreover, the conclusion allows for introspection on the key elements discussed. The benefits derived from Jump’s features, such as robust data visualization and insightful statistical analysis capabilities, highlight how effectively it supports analytical endeavors. For decision-makers, understanding these aspects can lead to informed choices that enhance productivity and accuracy in their projects.
Summarizing Key Insights
In this section, the prominent insights gathered throughout the article are distilled. Key takeaways include:
- Versatility Across Fields: Jump's applications range from academic research to business analytics and healthcare, showcasing its adaptability in various environments.
- User-Friendly Interfaces: The design of Jump facilitates a smooth user experience, making it approachable even for those with limited statistical backgrounds.
- Comprehensive Statistical Tools: Jump provides an extensive brack of statistical tests and graphical methods, ensuring users can conduct thorough analyses without switching between multiple software.
- Potential for Machine Learning Integration: As software evolves, Jump’s potential for incorporating machine learning algorithms positions it favorably in a competitive market.
These insights illuminate not just the functionalities of the software, but its practical implications in real-world scenarios.
Final Recommendations for Prospective Users
For organizations considering the implementation of Jump Statistical Software, specific recommendations include:
- Assessing Individual Needs: Before selection, evaluate the specific analytical tasks that the software must support. Understand how Jump specifically aligns with those goals.
- Trial Periods: Take advantage of any trial offerings to familiarize the team with Jump’s interface and tools. This practical experience is invaluable for comprehending its applicability.
- Training and Resources: Ensure that the team has access to training materials and user support. This is essential for maximizing the software’s potential.
- Consideration of Updates: Keep abreast of updates, as statistical software often evolves to include new features and enhancements in machine learning capabilities.
Understanding Jump through this structured analysis equips prospective users with the knowledge necessary to make informed decisions. By carefully considering organizational needs and weighing them against the capabilities of Jump, users can leverage its strengths to drive effective analytical practices.