Mastering CTMS Data Entry for Clinical Trials
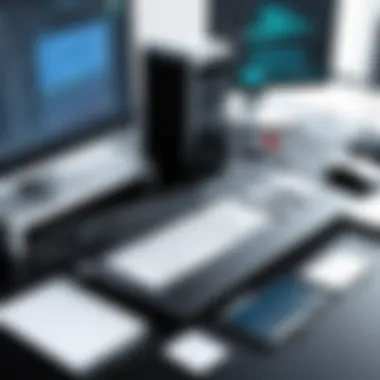
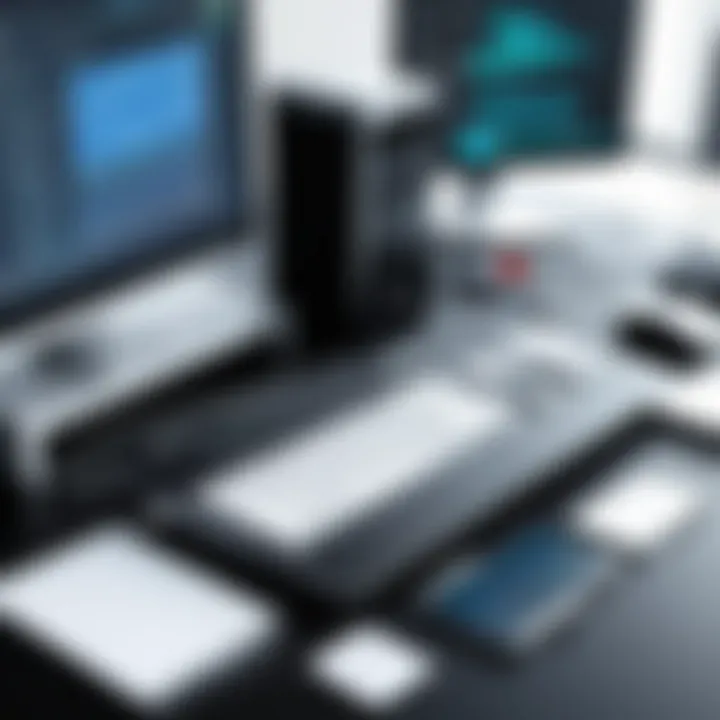
Intro
In the ever-evolving landscape of clinical research, the role of Clinical Trial Management Systems (CTMS) has become increasingly significant. Data entry, a fundamental aspect of CTMS, is critical to ensure that research outcomes are reliable, valid, and actionable. This guide delves into the various elements surrounding CTMS data entry, discussing its pivotal importance, the common challenges faced by professionals, and the best practices to adopt for improved accuracy and efficiency.
Understanding the basics of CTMS data entry can greatly enhance the workflow within clinical trials. Effective data management not only boosts the overall effectiveness of a trial but also plays a role in compliance with regulatory guidelines. With such high stakes involved, this comprehensive examination aims to provide insights that can significantly benefit clinical researchers and organizations.
Like any complex system, CTMS data entry comes with its hurdles. From data inconsistencies to user errors, these challenges can impede research quality and extend timelines. By highlighting best practices, we aim to empower professionals in refining their data entry strategies. Furthermore, we will explore the emerging trends that may reshape this field, shedding light on future advancements in the domain.
In summation, this guide serves as a resource for those aiming to enhance their understanding of CTMS data entry and improve their contributions to the clinical research landscape. The subsequent sections will provide a more detailed examination of relevant themes.
Intro to CTMS Data Entry
CTMS, or Clinical Trial Management System, serves as a pivotal platform in managing clinical trials. One of the core functions of CTMS is data entry. Understanding this process is vital for numerous reasons. First, the accuracy of data collected during clinical trials directly impacts the integrity of the research results. When data is entered incorrectly or not managed well, it can lead to misleading conclusions and affect patient safety.
Furthermore, effective data entry ensures compliance with regulatory requirements. Many governing bodies establish strict guidelines concerning data management in clinical trials. Failing to adhere to these guidelines can result in severe consequences, ranging from halted studies to legal liabilities.
In addition, the rise in data volume and complexity requires professionals to adopt best practices for data entry. This not only includes data collection procedures but also emphasizes the importance of proper training and technological integration.
Overall, mastering CTMS data entry is crucial for researchers and professionals involved in clinical trials. It enhances the quality of research outcomes while safeguarding the interests of all stakeholders involved in the process.
What is CTMS?
A Clinical Trial Management System is a software application designed to facilitate the planning, tracking, and management of clinical trials. It integrates various aspects of clinical research, including patient recruitment, data collection, and regulatory compliance. By centralizing data, CTMS enables researchers to maintain an organized, streamlined approach to managing trials. This reduces the scope for error and enhances the overall efficiency of the research process.
The CTMS approach allows for real-time data entry and monitoring, making adjustments more feasible as necessary. Moreover, there are various modules within CTMS that cater to specific needs such as trial design, budget management, and reporting. This software can significantly reduce administrative burden and improve decision-making.
The Role of Data Entry in Clinical Trials
Data entry in clinical trials plays a non-negotiable role in ensuring the validity of the research. Every trial generates vast amounts of data, from patient results to adverse event reports. Accurate data entry is what transforms raw data into usable insights that can inform medical decisions.
When professionals understand the importance of precise data entry, they can mitigate risks associated with human error and system integration issues. Additionally, well-executed data entry enhances data quality, which is essential for producing credible results. It allows researchers to analyze data trends and draw conclusions that can have far-reaching implications in the medical field.
In summary, CTMS data entry is not just a task to be checked off. It is a critical component that influences the trajectory of clinical trials, reinforcing the need for professionals in this industry to prioritize accuracy and efficiency in every entry.
The Importance of Accurate Data Entry
Accurate data entry in Clinical Trial Management Systems (CTMS) serves as the backbone for reliable clinical research. It ensures that the information captured during clinical trials is both precise and meaningful. Inaccurate or inconsistent data can lead to misguided decisions that could compromise patient safety, affect the credibility of research findings, and ultimately hinder the advancement of medical science.
Data entry is not just a mere administrative task; it significantly impacts almost every aspect of a clinical trial. Organized and accurate data facilitates seamless communication among various stakeholders, including researchers, regulatory bodies, and sponsors. It aids in maintaining the integrity of the research and supports the overall goals of clinical trials. Therefore, understanding the importance of accuracy in data entry is paramount for professionals in the field.
Impact on Research Integrity
Research integrity is a vital principle that underpins the credibility of clinical trials. Accurate data entry protects the validity of findings by ensuring that all collected information is true and represents what actually occurred during the study. When data entry is compromised, the results may become skewed, leading to incorrect conclusions.
The implications of compromised data can be profound:
- Misinterpretation of Results: Flawed data can mislead researchers and regulatory agencies.
- Loss of Trust: If stakeholders detect errors or inconsistencies, it can erode trust in the research process.
- Regulatory Consequences: Non-compliance due to poor data integrity can lead to sanctions or delays in approval.
"Accurate data entry is foundational for maintaining high research integrity, which is essential for advancing medical knowledge."
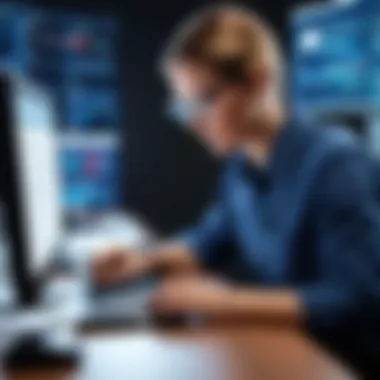
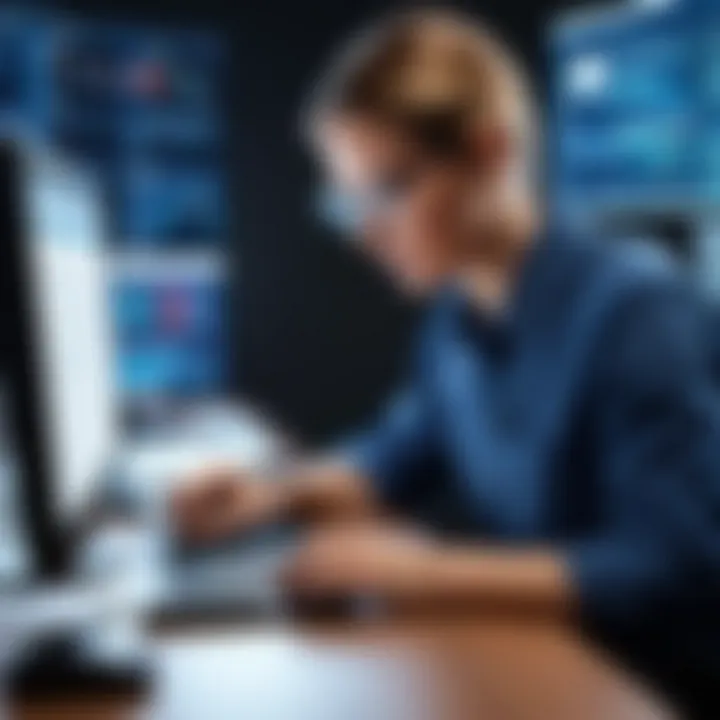
In addition, precise data entry aids in reproducibility, a concept that is central to scientific research. If data is recorded accurately, other researchers can replicate findings more easily, contributing to the body of knowledge in a trustworthy manner. This reproducibility strengthens the scientific method and enables continuous improvement in clinical practices.
Regulatory Compliance and Data Quality
Regulatory compliance is critical in clinical research, as various governing bodies, such as the FDA, require adherence to strict guidelines. Accurate data entry plays a crucial role in achieving compliance with these regulations. Maintaining data quality ensures that all necessary information is up to standard and that it satisfies both governmental and ethical requirements.
High-quality data is characterized by:
- Validity: Data must accurately reflect the true status and outcomes of the trial.
- Reliability: Consistent results should emerge regardless of the timing or circumstances under which they are collected.
- Completeness: All necessary data points must be captured to provide a holistic view of the study.
When professionals prioritize accurate data entry, they help ensure compliance with various regulations, thus facilitating smoother interactions with regulatory agencies. This compliance can result in faster approvals and streamlined processes, allowing vital research to reach patients more efficiently.
In summary, the importance of accurate data entry cannot be overstated. It is a cornerstone for research integrity and is essential for meeting regulatory standards. For organizations aiming to improve their clinical trial outcomes, investing in accurate data entry practices is an invaluable step.
Common Challenges in CTMS Data Entry
The process of data entry in Clinical Trial Management Systems (CTMS) presents a range of challenges that can complicate clinical trials. These challenges can impact the efficiency and accuracy of data management. Understanding these obstacles is essential for professionals in the field, as it helps to create strategies to overcome them.
Data Volume and Complexity
Clinical trials generate vast amounts of data, encompassing participant information, results, and regulatory documents. This sheer volume can lead to difficulties in managing and accurately entering data into CTMS. The complexity of the data also plays a role; it often includes various formats, such as text, numbers, and multimedia. This diverse data landscape makes standardization difficult. As a result, systems may face performance issues due to overload, which can further hinder the accuracy of data entry.
In this context, implementing robust data management protocols is critical. Utilizing appropriate software solutions can streamline the data entry process. Moreover, organizing data into manageable sections can help reduce the burden on data entry professionals.
Human Error and Data Inconsistency
Human error remains a significant challenge in CTMS data entry. In a high-pressure environment, mistakes can easily occur. Small errors, such as incorrect data entry or mislabeling, can lead to significant issues later in the research process. These errors can compromise the validity of trial results and necessitate costly corrections.
To mitigate human error, organizations should invest in training programs. Regular training can help enhance the skills of data entry personnel. Introducing double-check systems or cross-verification processes can also significantly reduce inconsistencies in data. Attention to detail is crucial in this field; an emphasis on accuracy will greatly improve data reliability.
System Integration Issues
Different platforms and tools involved in clinical trials often face integration challenges. Data needs to flow seamlessly between various systems, such as electronic health records, lab systems, and the CTMS. Lack of integration can lead to data silos, where information is trapped in individual systems and not shared effectively.
These integration issues can result in data loss, inconsistencies, and duplicated efforts. Solutions require the adoption of standardized data formats and integration protocols. Establishing a clear framework for communication among system components is essential. By addressing these integration challenges, the integrity of the data entry process can be enhanced.
"Addressing common challenges in CTMS data entry is essential for ensuring data accuracy and compliance with regulatory standards."
In summary, understanding the common challenges in CTMS data entry allows organizations to put more effective measures in place. By focusing on data volume and complexity, minimizing human errors, and tackling system integration issues, the overall quality of data entry in clinical trials can be significantly improved.
Best Practices for CTMS Data Entry
Enhancing CTMS data entry is a multifaceted endeavor that can significantly influence the overall quality and integrity of clinical trials. By adopting best practices, organizations can streamline processes, improve data accuracy, and maximize research outcomes. This section highlights key elements necessary for effective data entry within CTMS.
Standardization of Processes
Establishing standardized processes is fundamental for ensuring consistency across data entry tasks. Standardization helps to eliminate variability, reducing the risk of errors that may arise from different team members or departments applying disparate methods. A few practices to consider include:
- Develop uniform templates for data entry, ensuring that everyone uses the same format.
- Create detailed guidelines for data entry which serve as a reference for team members.
- Utilize clear definitions to describe data points and outcomes, ensuring that all users interpret the data consistently.
By focusing on standardization, organizations can cultivate a reliable environment prone to fewer mistakes, thus enhancing the quality of the data collected.
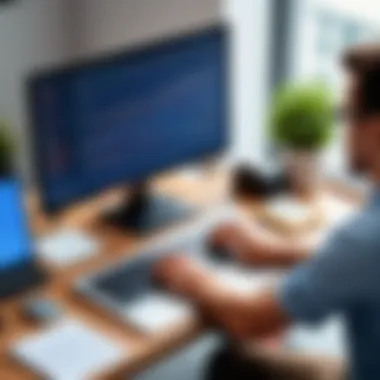
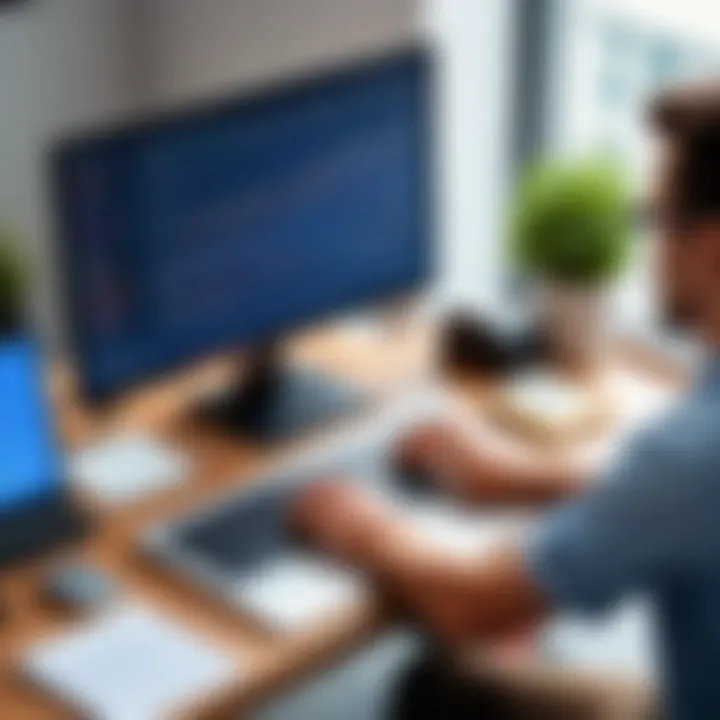
Regular Training and Skill Development
Humans are often the weakest link in a data entry process, making regular training essential. Conducting comprehensive training sessions ensures that team members are well-versed in both the CTMS software and the specific data entry standards in use. Key considerations are:
- Initial onboarding should include extensive training focused on the CTMS.
- Continuous skill development workshops, which can focus on updates in technology or changes in data management regulations.
- Feedback mechanisms that allow team members to share experiences and areas of difficulty, subsequently using this information to adjust training accordingly.
A well-trained team is more adept at recognizing discrepancies and reduces the likelihood of data quality issues.
Implementing Validation Techniques
To further ensure accuracy, validation techniques should be embedded within the data entry process. Implementing these methods can aid in swiftly identifying errors before they become embedded within the data set. Some effective techniques include:
- Automated data checks, which can identify discrepancies in real-time as data is entered.
- Validation rules set within the CTMS that enforce data integrity, such as format checks and range checks.
- Regular audits of data entries that help pinpoint systemic issues or recurring problems.
Validation techniques not only confirm data accuracy but also contribute to compliance with regulatory standards.
Adopting best practices in CTMS data entry goes beyond mere improvement; it establishes a solid foundation for effective clinical research and data integrity.
In sum, the power of best practices cannot be overstated. Standardization provides a clear roadmap, training equips the team with knowledge, and validation enhances quality assurance. Collectively, these elements create a robust environment that fosters successful outcomes in clinical trials.
Technological Innovations in Data Entry
The landscape of CTMS data entry is evolving rapidly due to technological innovations. These advancements are reshaping how data is collected, processed, and interpreted. Understanding these innovations is crucial for professionals in the clinical research field. They can lead to improved accuracy, efficiency, and user experience, all essential for the demands of modern clinical trials.
Automation and Machine Learning
Automation plays a pivotal role in streamlining data entry processes. By minimizing manual input, automation reduces the likelihood of human errors that can compromise data integrity. The integration of machine learning algorithms further enhances this process. These algorithms can learn from historical data patterns and optimize data entry tasks over time. For instance, they can identify anomalies or suggest corrections in real-time, offering a more robust framework for data management.
Some benefits of automation and machine learning in CTMS data entry include:
- Increased Speed: Automated tools can process data much faster than manual entries.
- Enhanced Accuracy: Machine learning reduces human errors, leading to cleaner datasets.
- Resource Optimization: Automating routine tasks allows data entry professionals to focus on more complex issues.
- Scalability: As trial sizes grow or change, automated systems can scale up accordingly without a decline in performance.
However, organizations must approach automation with caution. There is a need for proper validation of algorithms and an understanding of potential biases that might influence data output.
Data Visualization Tools
Data visualization tools are essential in the context of CTMS data entry, making complex data more accessible and comprehensible. These tools transform raw data into graphical representations, enabling stakeholders to gain insights quickly and effectively. Using data visualization, trends and patterns can be easily identified, fostering better decision-making.
Key aspects of data visualization tools in CTMS include:
- Dashboards: These offer real-time performance indicators, helping teams to monitor ongoing trials closely.
- Interactive Reports: Users can drill down into specific datasets, allowing for more granular exploration of the data.
- Collaboration Features: Many modern visualization tools include mechanisms for sharing insights across departments, enhancing communication.
Incorporating these tools requires training and a shift in perspective regarding data utilization. However, the long-term benefits outweigh the initial challenges, making it a worthwhile investment.
"Innovations in technological tools bring forth enhanced capabilities for data entry, offering potent solutions to long-standing issues in clinical trials."
In summary, technological advancements in automation, machine learning, and data visualization not only improve data entry practices but also contribute significantly to the overall integrity of clinical trials. Stakeholders must embrace these innovations to stay competitive and improve research outcomes.
The Future of CTMS Data Entry
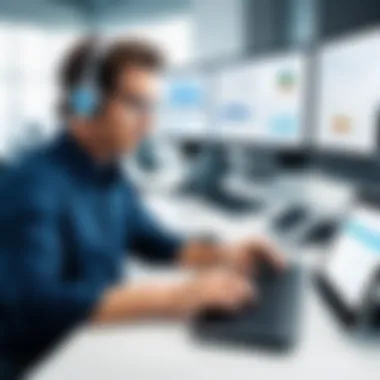
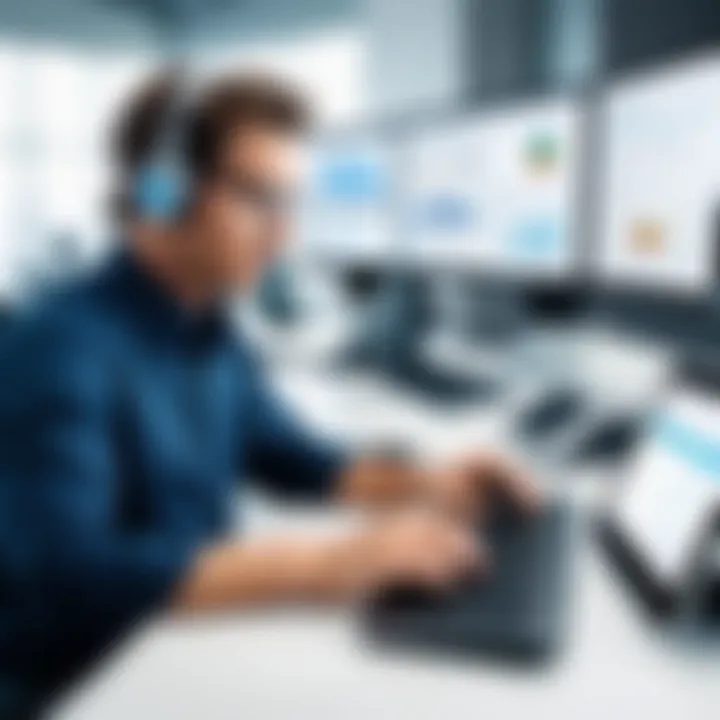
Understanding the future of Clinical Trial Management System (CTMS) data entry is crucial for professionals in clinical research. As the landscape evolves, so do the tools and techniques used in data entry. Advancements in technology will greatly influence how data is collected, processed, and analyzed. This progression not only enhances efficiency but also improves accuracy, a vital factor in research integrity.
Emerging Trends in Clinical Trials
The clinical trials sector is seeing rapid changes driven by technology. Several key trends are shaping the future of CTMS data entry:
- Decentralized Clinical Trials (DCTs): These trials move much of the data collection out of traditional clinical settings. This transition requires robust data management systems that integrate diverse sources of information.
- Patient-Centric Approaches: More emphasis is placed on patient experiences within trials. Systems that allow for direct patient data input can improve the quality and relevancy of the information collected.
- Real-Time Data Collection: With advances in mobile technology and IoT, real-time data collection becomes feasible. This timely access to data ensures quicker decision-making and increased agility during trials.
- Use of Wearable Devices: Devices that monitor health metrics are increasingly common. Data from these sources needs integration into CTMS for comprehensive analysis.
These trends not only change how data is entered but also highlight the importance of flexible and adaptive CTMS solutions that can integrate new data sources seamlessly.
Predictions for Data Management
The future of data management within CTMS will likely revolve around several predictions:
- Increased Automation: As AI and machine learning tools continue to advance, more data entry tasks can be automated. This reduces human error and speeds up the data collection process.
- Enhanced Data Security: With the rise of cyber threats, data security measures will become more sophisticated. Systems must evolve to ensure compliance with regulations like GDPR and HIPAA.
- Cloud-Based Solutions: More organizations will adopt cloud-based CTMS solutions. This allows for greater collaboration, accessibility, and ease of updates in systems.
- Integration of Advanced Analytics: Data entry will not only focus on collection but also on analysis. Advanced analytics will provide insights that inform decision-making in real-time.
"The future of CTMS data entry is not just about technology; it's about a transformation in how we understand and utilize data in clinical research."
In summary, the future of CTMS data entry is intertwined with technological advancements and the evolving needs of the industry. Embracing these changes will be decisive for the success of clinical trials in the coming years.
Case Studies on Effective Data Entry Practices
Case studies provide a crucial lens through which best practices in CTMS data entry can be examined. These real-world examples illustrate how various organizations have faced and overcome the challenges of data management. By studying these cases, professionals can gain insights into effective methodologies, reduce the learning curve, and improve their own practices significantly.
Successful Implementations in the Industry
Successful implementations of CTMS data entry systems are abundant across numerous sectors in clinical trials. One notable example is the partnership between PharmaTech Solutions and a major pharmaceutical company. They developed a CTMS specifically tailored to enhance data entry efficiency. The system reduced manual entry errors by incorporating automated data validation processes.
Another illustrative case comes from Clinical Trials Corp, which revamped its entire data management procedure to embrace cloud-based technologies. This shift not only provided real-time access to data for all stakeholders but also simplified data collection methods, encouraging higher compliance with data entry protocols across the board.
Implementation of these systems provided the following benefits:
- Reduction of Data Entry Errors: Automation minimized human involvement in repetitive tasks.
- Faster Data Access: Cloud-based systems enabled quick retrieval, enhancing overall project timelines.
- Improved Collaboration: Teams could work cohesively across different locations with consistent data visibility.
Lessons Learned and Key Takeaways
From analyzing various successful implementations, several key lessons emerge that can guide future projects. Here are some important takeaways:
- Invest in Training: Even the best systems can fail without comprehensive training for users. Continuous education is critical for maximizing the potential of any data entry system.
- Adopt Flexibility: Systems should remain adaptable to changes in protocols or regulatory requirements. This flexibility can significantly extend the lifespan and efficiency of the CTMS.
- Focus on User Experience: A user-friendly interface encourages adherence to data entry practices. Engaging users in the system design can lead to more effective workflows.
"The integration of effective data entry practices must not only be about technology but also emphasize the human element in data management."
End
The conclusion serves as a crucial part of this comprehensive guide on CTMS data entry. It consolidates the insights shared throughout the article, emphasizing the importance of precise data handling in clinical trials. This not only preserves the integrity of research but also assures that regulatory bodies can trust the data presented. Accurate data entry ensures that studies yield valid and reliable results, subsequently influencing decision-making in medical and clinical practice.
Summarizing the Key Points
In reviewing the article, several key points emerge:
- CTMS Definition: A Clinical Trial Management System is essential for managing clinical trials effectively. It streamlines data entry and monitoring processes.
- Importance of Accuracy: Precision in data entry impacts research outcomes dramatically. Accuracy is not just a best practice; it's a necessity for regulatory compliance and research validity.
- Challenges Faced: The volume and complexity of data, coupled with potential human error, can lead to inconsistencies that undermine research integrity.
- Best Practices: Standardization of processes, regular training, and validation techniques enhance data quality and efficiency.
- Technological Advances: Innovations like automation and data visualization tools are reshaping how data is managed, presenting both opportunities and challenges.
- Future Trends: As clinical trials evolve, understanding emerging trends provides a roadmap for success in data entry practices.
Looking Forward in CTMS Data Entry
The landscape of CTMS data entry is changing rapidly. The future may witness:
- Increased Automation: Enhancements to automation in data entry will likely reduce time and human error. This streamlining can lead to faster study completions.
- Use of AI: Artificial intelligence will play a larger role in data management, analyzing vast amounts of data more accurately than traditional methods.
- Regulatory Adaptations: As new technologies emerge, regulatory standards may evolve, requiring continuous adaptation in data entry practices to maintain compliance.
- Collaborative Platforms: There might be a shift towards more integrated systems where various stakeholders can access and enter data collaboratively in real time.
- Enhanced Training Programs: Ongoing education and skill development will ensure that professionals are prepared for these changes, allowing them to leverage new tools effectively.