Understanding Decision Support Systems: Types and Trends
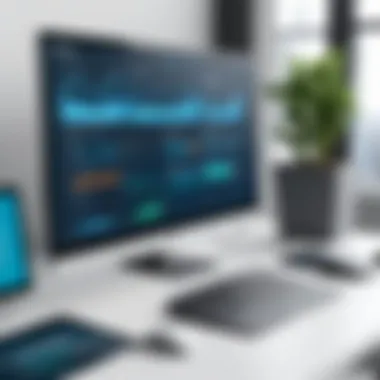
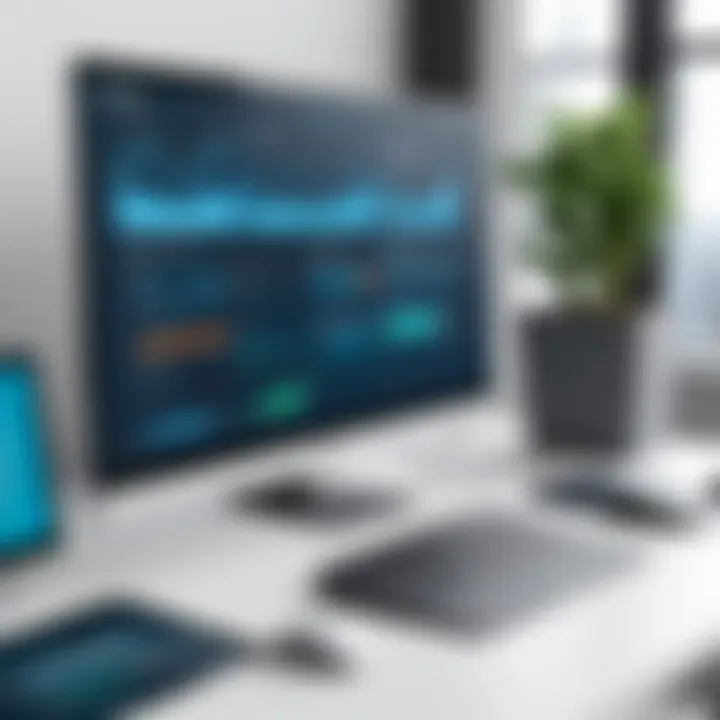
Intro
The landscape of decision-making has undergone a significant evolution in recent years. With an incessant influx of data, organizations find themselves at a crossroads where the need for swift and informed decisions is paramount. Happily, this is where Decision Support Systems (DSS) come into the spotlight. They are intricately woven into the fabric of data-driven decision-making, helping users sift through mounds of information to find insights that matter.
DSS can be seen as a bridge between raw data and actionable insight. They include an array of tools and technologies designed to assist with complex decision-making processes, equipping managers and analysts to transform data into significant information that drives strategic initiatives.
The essence of DSS lies in its ability to integrate data analytics, user-friendly interfaces, and existing organizational processes. As we delve deeper into this exploration, we will examine the finer points of DSS, unraveling their types, key features, and the methodologies that underpin their effectiveness.
Understanding Decision Support
Understanding decision support is crucial in navigating the complexities of modern organizational environments. In a world where information is proliferating at an unprecedented rate, the ability to process and interpret this data effectively determines the success of decision-making processes. Decision Support Systems (DSS) play an essential role in this arena, acting as a bridge between raw data and actionable insights.
Definition and Importance
A decision support system can be defined as an information system that supports business or organizational decision-making activities. Unlike traditional systems that focus purely on data transaction processing, DSS emphasizes a strong analytical capability. They allow managers to leverage both structured and unstructured data, providing powerful tools to analyze complex scenarios and forecast outcomes.
The importance of DSS cannot be overstated. They're essential for several reasons:
- Enhanced Decision-Making: By synthesizing data from various sources, DSS enables informed choices based on comprehensive insights.
- Speed and Efficiency: These systems speed up the decision-making process, allowing organizations to respond swiftly to market changes.
- Risk Analysis: DSS provide predictive modeling capabilities that help in assessing potential risks and outcomes, ensuring a proactive approach to decision-making.
In essence, decision support systems empower organizations to make sense of vast amounts of information whilst improving the quality and speed of decisions.
Historical Context
The evolution of decision support systems can be traced back to the early 1960s when businesses began to recognize the need for more sophisticated tools to handle decision-making processes. Originally, these systems mainly focused on data management and outputting predefined reports. However, as computer technology advanced, so did the capabilities of DSS.
During the 1970s and 1980s, DSS began to incorporate more advanced analytical models and user-friendly interfaces. This era saw the introduction of tools that could analyze data trends and patterns, providing decision-makers with insights they didn’t previously have.
The 1990s marked a significant turning point with the emergence of the internet, which revolutionized data access and sharing capabilities. Decision support systems evolved to integrate web-based data, enhancing their relevance and usability in organizations of all sizes.
Today, with the rise of big data and artificial intelligence, DSS have become more sophisticated than ever. They now incorporate machine learning algorithms that can continually learn and adapt, ultimately providing even deeper insights into decision-making. The journey from simple report generators to advanced analytical tools illustrates the incredible potential of decision support systems in facilitating better business outcomes.
Types of Decision Support Systems
Understanding the types of Decision Support Systems (DSS) is crucial for organizations aiming to enhance their decision-making processes. Each type serves a specific purpose, catering to varying business needs and scenarios. By recognizing the diverse functionalities and advantages of these systems, organizations can choose the most appropriate DSS to align with their operational demands.
Data-Driven DSS
Data-Driven DSS primarily focuses on harnessing vast amounts of data to support decision-making. This type of system is often equipped with sophisticated tools for data retrieval and extraction, transforming raw data into meaningful information. For instance, a retail company might utilize a data-driven DSS to analyze customer purchasing patterns. This could enable managers to identify trends and make informed decisions regarding inventory and marketing strategies.
Benefits of Data-Driven DSS include:
- Enhanced Decision Accuracy: Decisions based on concrete data tend to be more reliable.
- Timeliness: Quick access to real-time data can significantly speed up the decision-making process.
- Comprehensive Insights: Users can explore data from various angles, finding insights that may not be immediately apparent.
Model-Driven DSS
Model-Driven DSS relies on mathematical and analytical models to assist in decision-making. These systems simulate different scenarios, providing users with hypothetical results based on varying inputs. For example, a logistics company could use a model-driven DSS to evaluate different routing options for delivery trucks. The system would allow managers to input parameters such as cost, distance, and time constraints to derive the most efficient routing plan.
Some important points to consider regarding model-driven DSS include:
- Scenario Analysis: Users can assess potential outcomes before making a decision, minimizing risks.
- Support for Complex Problems: This type of DSS is particularly beneficial for tackling multifaceted issues where numerous variables are involved.
- Visualization Tools: Often, these systems come with tools to visualize model outputs, aiding in comprehension and communication of strategies.
Knowledge-Based DSS
Knowledge-Based DSS harnesses expert knowledge and specific business rules to aid decision-making. Different from data-driven and model-driven counterparts, this system taps into databases of experience and practices to suggest solutions. In healthcare, for instance, a knowledge-based DSS can analyze patient symptoms and recommend possible diagnoses and treatments.
Characteristics of this DSS include:
- Expert Systems: They utilize rules and logic established by field experts to guide decisions.
- Learning Ability: Many knowledge-based systems can adapt over time, incorporating new information and improving their recommendations.
- User Support: They often provide rationale for recommendations, enhancing user understanding of the decision-making process.
Hybrid Systems
Hybrid Systems combine the features of data-driven, model-driven, and knowledge-based DSS, providing a more versatile platform for decision support. Such systems offer a comprehensive approach by enabling analytics, simulations, and expert knowledge all within a single framework. For instance, a financial institution might use a hybrid DSS for risk assessment, applying data analytics for real-time market trends, models for forecasting, and knowledgeable input from financial analysts.
When considering hybrid systems, organizations should keep in mind:
- Flexibility: They can be customized to meet specific organizational needs, accommodating different decision processes.
- Integrated Insights: By leveraging various information types, users gain a holistic view of the problem at hand.
- Cost-Effectiveness: Although setting up a hybrid system might require a significant initial investment, the comprehensive decision support it offers can lead to long-term savings by making better decisions.
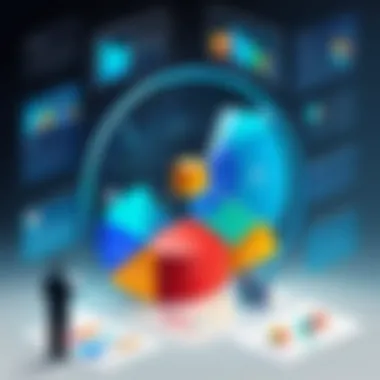
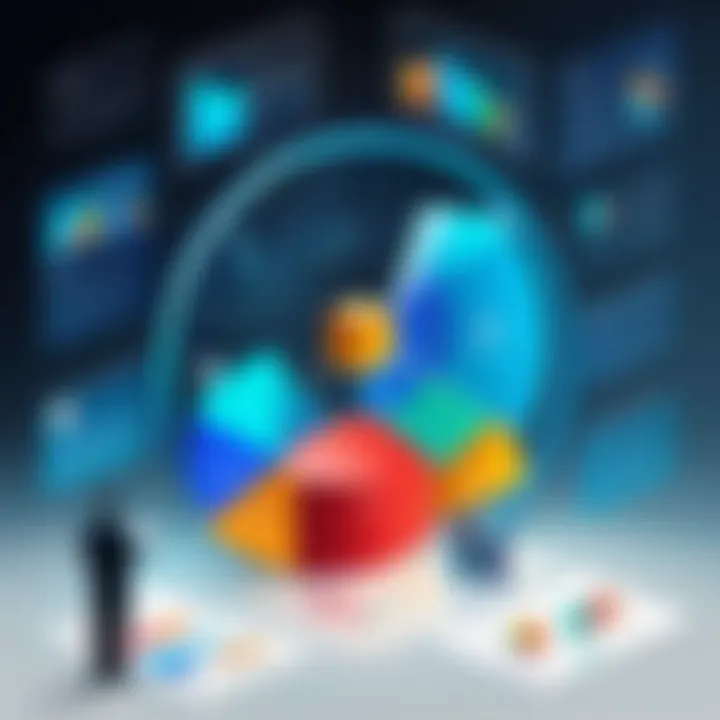
Hybrid systems are often seen as the Swiss Army knife of decision support, equipped to handle a wide range of situations effectively.
Components of Decision Support Systems
A thorough understanding of the components of Decision Support Systems (DSS) is pivotal for organizations looking to enhance their decision-making capabilities. These components form the backbone of a DSS, enabling it to function effectively and provide valuable insights. When organizations align their decision-making processes with robust components, they can tackle challenges, streamline operations, and ultimately achieve better outcomes.
Database Management
The database management component is critical to any effective Decision Support System. It acts as the reservoir of data, storing both historical and real-time information that decision-makers draw upon. A well-structured database allows for efficient data retrieval, ensuring that users have quick access to relevant information when needed. This can include diverse data types, such as sales figures, customer feedback, and inventory levels.
Furthermore, data mining and cleansing processes are essential to maintaining data quality. If the data is inaccurate or incomplete, the insights drawn could lead to poor decisions. Therefore, organizations must invest in reliable database management systems that support data integrity and facilitate easy updates. More than just storage, the database also needs to be scalable to handle increasing volumes of data as businesses grow.
Model Base Management
Model Base Management serves as the analytical engine in a Decision Support System. Here, various models are stored and manipulated to assist in complex decision-making processes. This might include statistical models, optimization models, or simulations that reflect different business scenarios. Each model holds a specific purpose, be it forecasting trends, evaluating risk, or assessing outcomes of various strategies.
When utilizing models, organizations often need to tailor them to match their unique circumstances. This customizability ensures the models reflect real-world conditions relevant to the decision at hand. As new data comes in, models can be adjusted or replaced, making model base management not just a function but a dynamic component that evolves alongside the business landscape.
"The true power of the Decision Support System lies in its capacity to simulate various scenarios and predict possible outcomes based on user-defined parameters."
User Interface Design
Last but not least, User Interface Design plays a vital role in the effectiveness of a Decision Support System. A clean and intuitive interface ensures that users can navigate the system with ease, accessing the necessary tools and data without hassle. This is especially important in a fast-paced environment where quick decisions are often critical.
Good user interface design considers user needs and anticipates the types of queries they may seek to resolve. Features such as dashboards, visualization tools, and drag-and-drop functionalities make data accessible and insights clear at a glance. User feedback should drive design choices, creating an interface that aligns with how users think and work. This enhances user adoption and reduces resistance, ultimately leading to more informed and timely decision-making.
Methodologies in Decision Support
Methodologies in decision support systems are at the heart of effective data-driven decision-making. They provide the frameworks and processes that guide the analysis, interpretation, and presentation of data, ultimately aiding organizations in making more informed choices. As businesses continue to navigate complexities in the marketplace, understanding these methodologies becomes paramount.
The right methodology can unlock valuable insights from raw data. However, choosing the right approach isn’t a matter of picking from a menu; it involves a careful assessment of both the organizational context and the specific challenges at hand. Each methodology carries its own strengths and weaknesses, and the successful implementation of any decision support system hinges on effectively aligning these methodologies with an organization’s objectives.
Data Mining Techniques
Data mining is a fundamental component of decision support systems. It involves extracting patterns from large datasets to unearth hidden relationships and trends that may not be immediately apparent. The primary techniques include clustering, classification, regression, and association rule learning.
- Clustering helps categorize data into groups based on similarities, making it simpler to analyze.
- Classification assigns categories to data points, which aids in predicting outcomes based on known information.
- Regression identifies relationships between variables, useful in forecasting trends.
- Association rule learning uncovers interesting relationships between items in large datasets.
In practice, these techniques can be applied in various industries. For example, retail businesses often use clustering to segment customer types, influencing marketing strategies.
Statistical Analysis Methods
Statistical analysis provides a robust framework for understanding data sets and making inferences about them. It encompasses hypotheses testing, confidence intervals, and various statistical tests such as t-tests or ANOVA. These methods are essential to validate findings, whether for market research or operational efficiency assessments.
Statistical analysis can often reveal whether a trend is genuine or merely incidental. By rigorously testing assumptions and examining variances, organizations can make informed predictions about future behaviors and outcomes. A common scenario would be analyzing customer feedback trends over quarters, where statistical methods would help separate significant shifts from random, non-significant changes.
Predictive Analytics
Predictive analytics leverages statistical techniques and data mining to forecast future events. It transforms vast volumes of historical data into actionable insights. The process typically involves building models that analyze existing data patterns and apply them to predict future behaviors or trends.
Adopting predictive analytics can drastically enhance decision-making. For instance, a healthcare organization might use it to predict patient admission rates, allowing for resource allocation and staffing adjustments. This foresight can lead to improved service delivery and reduced costs.
Predictive analytics is powerful but requires a robust understanding of both the data and the context in which it operates. Successful implementation often includes iterative improvement processes, where models are continually refined based on new data and outcomes.
In summary, integrating these methodologies into decision support systems is critical for harnessing the power of data. Organizations can transform complex insights into actionable strategies, driving improved performance and informed decisions.
Integration of DSS in Business Processes
The integration of Decision Support Systems (DSS) within business processes is not just a trend but a vital element in modern organizational strategy. A well-structured DSS can serve as the backbone for informed and effective decision-making, streamline workflows, and enhance overall productivity. Its role in shaping the fabric of businesses today cannot be overstated, especially as competition intensifies and the need for swift, data-driven decisions becomes paramount.
Key benefits of integrating DSS into business processes include improved efficiency, better alignment of resources with organizational goals, and enhanced communication across departments. When these systems are harnessed correctly, they empower businesses to react promptly to market changes and internal challenges.
Aligning Strategy with Technology
Aligning technology with business strategy is essential; this ensures that a company's goals are met through the smart use of resources. A DSS bridges the gap between data availability and strategic decision-making. For instance, a retail chain may use DSS to analyze sales data patterns, forecast inventory needs, and guide promotional activities. This allows the company to not only anticipate customer demands but also tailor their strategies accordingly.
"Strategy is not just about where you're going, it's about aligning every tool at your disposal to realize that vision."
The alignment process involves a series of steps:

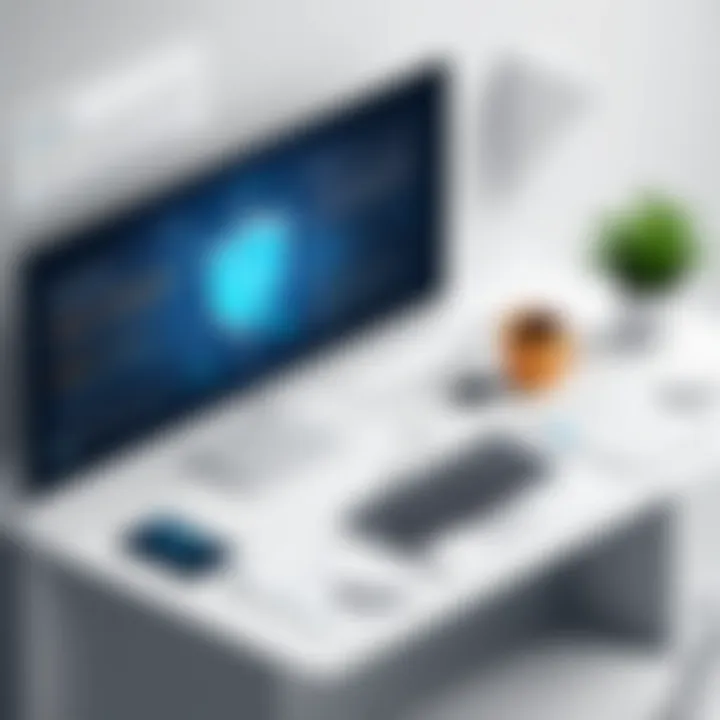
- Identifying business goals: Understand what the organization aims to achieve.
- Evaluating current technology: Assess the existing systems in place and their capabilities.
- Integrating DSS: Ensure that the selected DSS complements and enhances the overall strategy instead of complicating it.
- Training personnel: Equip staff with the necessary skills to leverage the DSS effectively.
Enhancing Collaboration
A solid decision support system contributes significantly to collaborative environments within a business. In places where many heads might be better than one, DSS provides a common platform for sharing insights and making collective decisions. For example, if a healthcare organization uses a DSS, team members can collaboratively evaluate patient data, treatment options, and resource allocation.
By breaking down silos, a DSS fosters open communication. Here’s how collaboration is enhanced:
- Shared data access: All relevant stakeholders can access the same information, minimizing discrepancies.
- Multi-disciplinary teamwork: Diverse groups can work on problems together without the limits of geography or time.
- Real-time updates: Decisions can be adjusted based on the latest data, ensuring the team is always on the same page.
Streamlining Operations
Streamlining operations is another area where DSS plays a crucial role. Inefficiencies often stem from poor data management and lack of coherent processes. By adopting a DSS, businesses can automate routine tasks, analyze large datasets for insights, and remove redundancies. This not only saves time but also directs attention towards more strategic initiatives.
Consider manufacturing, where data is crucial in monitoring production lines. A well-integrated DSS can help managers track performance metrics in real-time, enabling quick adjustments to prevent downtime.
Some methods to streamline operations include:
- Automating reporting: Get immediate insights rather than waiting for periodic updates.
- Centralizing data: Store and manage data in a unified location to improve accessibility.
- Predictive analytics: Anticipate operational challenges before they arise, allowing for proactive measures.
Through effective integration of DSS, organizations can transform their approach to decision-making, strengthen their strategies, foster collaboration, and enhance their operational efficiency.
Challenges in Implementing Decision Support Systems
When organizations consider the introduction of Decision Support Systems (DSS), they often focus on the potential benefits. However, the road to successful implementation is fraught with challenges that must be navigated with care. These challenges significantly impact how effectively a DSS can function within a corporate structure. Understanding these hurdles is critical for professionals seeking to maximize the advantages of these systems while minimizing setbacks.
Data Quality Issues
Data is the lifeblood of any Decision Support System. If the data feeding into the system is flawed or incomplete, it leads to poor decisions — and nobody wants to ride that rollercoaster. Data quality issues come in various forms:
- Inaccurate Data: Mistakes during data entry or data migration can introduce inaccuracies.
- Inconsistent Formats: Different data sources may present information in varying formats, making aggregation and analysis more complex.
- Outdated Information: Relying on old data can completely skew results, leading executives down the wrong path.
Organizations must establish robust data governance practices to ensure incoming data meets quality standards before it enters the DSS.
"The integrity of data is paramount; garbage in, garbage out often rings true in decision-making processes."
User Resistance and Adoption
Even if a DSS is technically sound, if the users are not on board, then it's like driving a car without a steering wheel. The human factor can often be the most problematic. User resistance may stem from a variety of causes:
- Fear of Change: Established processes are hard to let go of. Employees might resist new tools that disrupt their familiar workflow.
- Training Gaps: If users haven't properly been trained to navigate the new system, frustration ensues.
- Perceived Complexity: A DSS that is not intuitive may deter users from fully utilizing its features.
To combat user resistance, organizations should foster a culture of openness, providing thorough training and clearly communicating the value of the DSS in terms of making their work easier instead of harder.
Costs and Resource Allocation
The financial aspect of implementing a Decision Support System cannot be overlooked. Costs can quickly balloon beyond initial estimates, leaving organizations scrambling to adjust their budgets. Key concerns when it comes to costs include:
- Initial Investment: The upfront costs for software, hardware, and training can be significant.
- Ongoing Maintenance: Apart from initial costs, there are ongoing operational expenses involved in maintaining and updating the systems.
- Resource Allocation: Organizations must also consider staffing needs, which might include hiring skilled personnel or reassigning current staff to manage the DSS effectively.
Balancing these expenses with the expected return on investment (ROI) can indeed be a tricky business. Organizations must carefully analyze both the short and long-term financial impacts of their decisions concerning DSS.
Ethical Considerations in Decision Support
Understanding the ethical implications surrounding Decision Support Systems (DSS) is crucial. Organizations employing these systems must navigate a complex landscape of responsibility as decisions increasingly rely on automated processes. As a cornerstone of modern business intelligence, DSS must be utilized not only for their capability to enhance efficiency but also for the ethical considerations they bring to the table. Factors like data integrity, equity, and accountability are just a few aspects that require careful attention when implementing these systems.
Bias in Data and Algorithms
Bias within data and algorithms can significantly distort the outcomes produced by decision support systems. When biased data is fed into a DSS, the decisions it promotes may reflect social inequalities, unintentionally favoring certain groups over others. This phenomenon can stem from various sources, including:
- Historical patterns in the data that perpetuate stereotypes.
- Limited diversity in data sets, failing to represent the entire population.
- Misalignment of algorithmic objectives with ethical standards.
To illustrate, consider a hiring algorithm that learns from previous recruitment outcomes. If past hiring decisions favored candidates from specific demographics, the DSS may inadvertently continue this trend, resulting in less diversity in the workplace. A good practice is to audit datasets regularly, ensuring they reflect the diversity of the current social landscape. Moreover, implementing testing frameworks can HELP identify and mitigate any potential bias before deploying these systems in real-world scenarios.
Accountability and Transparency
In a world where decision-making increasingly lies within computational frameworks, accountability becomes paramount. Organizations can find themselves with difficult questions about who is responsible for the choices made by DSS. Establishing mechanisms for transparency ensures that all stakeholders understand how decisions are formulated. Factors that contribute to improved accountability include:
- Clear documentation of the algorithms used in the DSS.
- Regular assessments to gauge the system's performance in real-time scenarios.
- Communication protocols to inform affected parties about decisions influenced by the system.
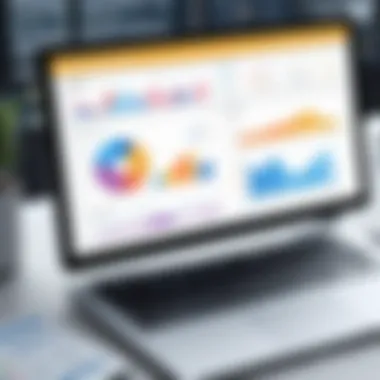
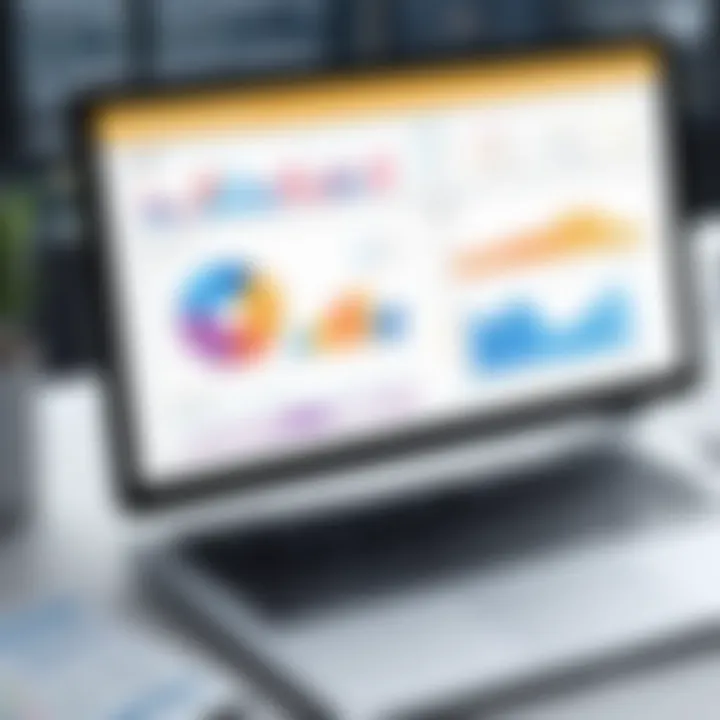
Transparency leads to trust, which is fundamental when integrating DSS into business practices. When companies openly share their methods and data usage, they cultivate a sense of shared responsibility among team members and stakeholders. This not only builds the credibility of the systems but also encourages ethical awareness among users.
"Transparency is not just good for the public good; it often leads to better decision-making processes within organizations."
By addressing both bias and accountability, organizations can lay the groundwork for ethical deployment of decision support systems. As they move toward the future, establishing solid ethical frameworks will guide them in making informed, fair, and justified decisions.
Future Trends in Decision Support Systems
The landscape of decision support systems is rapidly evolving, influenced by technological advancements and changing organizational needs. Understanding these future trends is essential for businesses looking to stay ahead in the game. By examining what lies ahead, organizations can strategically position themselves to enhance decision-making processes and ultimately improve performance.
The growth of data and the necessity for efficient decision-making processes compel organizations to adapt and innovate. This section reviews two significant trends shaping the future of decision support systems, particularly the integration of AI and machine learning, along with the push for real-time decision-making.
AI and Machine Learning Integration
Artificial Intelligence and machine learning are carving out a significant role in decision support systems. These technologies facilitate close collaboration between data analytics and operational processes, transforming raw data into actionable insights. As the algorithms behind machine learning become more sophisticated, organizations find themselves better equipped to predict outcomes and optimize strategies effectively.
- Enhanced Predictive Capabilities:It’s not just about analyzing data anymore but leveraging it to forecast future trends and behaviors. Machine learning models analyze past data and adapt their predictions based on new information, providing a more dynamic approach to decision-making.
- Automation of Routine Decisions:By automating mundane decisions, organizations can free up human resources for strategic tasks. For example, an e-commerce company can use AI to automatically determine optimal inventory levels, thereby streamlining operations without constant human intervention.
- Adaptive Learning:Machine learning systems continuously learn from new data inputs, enhancing their accuracy over time. This adaptive capability is crucial in industries where customer preferences, market trends, and competition are ever-changing.
"The integration of AI into decision support systems is not merely a trend; it's a transformation that redefines how companies use data."
- Personalization in Decision-Making:As organizations harness the power of AI, they can tailor decision-making processes to fit individual and segmented needs. This means a financial institution can provide personalized loan offerings based on a customer’s unique situation and behavior analysis, leading to more satisfied customers.
Real-Time Decision-Making
The demand for real-time decision-making is another trend gaining traction as organizations grapple with the fast pace of today’s business environment. Making decisions quickly can offer a competitive advantage, particularly in industries such as finance, retail, and logistics. Real-time decision-making allows businesses to respond and pivot swiftly in response to changing conditions.
- Immediate Data Processing:With advancements in cloud computing and big data technologies, organizations can process vast amounts of data instantaneously. This capability ensures timely insights into key performance indicators, enabling quicker reactions to market changes.
- Decision-Making at All Levels:Real-time systems allow not only executives but also middle management and operational staff to participate in data-driven decisions. This democratization of decision-making fosters a sense of ownership across the organization.
- Agility and Responsiveness:In sectors like emergency services or supply chain management, the ability to make fast and informed decisions can save both time and resources. For instance, logistics companies can reroute shipments based on real-time traffic data, optimizing delivery performance while enhancing customer satisfaction.
As organizations look to the future, embracing AI and a real-time approach to decision-making appears to be essential. These trends not only provide the tools for safeguarding their present but also pave the way for a more innovative and informed future.
Selecting the Right Decision Support Software
The choice of a Decision Support Software (DSS) can greatly influence the effectiveness of decision-making within an organization. Finding the right tool involves carefully analyzing various aspects of the software that align with organizational goals. Not every solution fits every need, so understanding the specific demands of your business is key. When you're amidst a bustling market brimming with options, it's easy to feel like a fish out of water, but a focused approach can lead you to the right fit.
Assessing Organizational Needs
Before diving into vendor selection, it’s crucial to undertake a thorough assessment of organizational needs. This starts with identifying the core objectives that the DSS needs to address. Ask essential questions like:
- What decisions need support?
- Which data sources are currently available?
- What type of analysis is required?
- Who will use the system?
By painting a clear picture of requirements, organizations can avoid the pitfalls of wrong choices that might lead to wasted resources or inadequate decision feedback. In many scenarios, employees end up frustrated with software that complicates rather than simplifies the decision-making process. Therefore, allocating time to map out processes and gather input from potential users can yield invaluable insights.
Comparative Analysis of Features
Once the organizational needs are understood, focus shifts to a comparative analysis of features offered by various DSS tools. It helps in establishing a clear benchmark to evaluate options. Key attributes to consider include:
- Data Integration: Does the software seamlessly merge with existing data sources?
- User Interface: Is it user-friendly and intuitive? Users must be able to navigate the system without feeling overwhelmed.
- Analytical Capabilities: Does it offer robust analytical tools to meet current and future requirements? This could range from basic reporting to advanced predictive analytics.
- Scalability: Can the software grow alongside your organization? A scalable solution prevents future headaches as needs evolve.
Developing a comparison checklist can help keep track of pros and cons, ensuring that the ultimate choice is data-driven rather than gut-feeling based. It may be beneficial to involve stakeholders from various departments in this analysis, ensuring a well-rounded perspective.
Vendor Considerations and Evaluation
Once a shortlist of choices is prepared, the next step focuses on vendor considerations and evaluation. Every vendor carries its unique strengths and weaknesses, which can greatly influence the overall satisfaction with the DSS. Here are crucial factors to evaluate:
- Reputation and Experience: Research vendor history. A well-established company with a proven track record is usually a safer bet.
- Customer Support: Ensure that the vendor offers robust support post-implementation. This is particularly crucial for tackling any issues quickly to avoid disruptions in decision-making.
- Pricing Structure: Analyze the pricing model. Hidden costs can sneak up, so it’s vital to have a clear understanding of the total cost of ownership.
- Customization Options: Determine whether the provider allows tailoring solutions to fit specific industry needs. This feature can significantly enhance the value of the DSS.
In closing, the task of selecting the right decision support software is not one to be taken lightly. Careful thought and planning can save organizations from headaches down the road. Remember, a wise decision today paves the way for smoother operations and smarter choices in the future.
The End
The examination of Decision Support Systems (DSS) showcases not only the technical intricacies but also the pivotal role they play in modern organizations. In an era where data-driven decisions can make or break a business, understanding the elements of DSS gives professionals a competitive edge. An effective conclusion synthesizes the primary insights from the entire discourse and rekindles the relevance of decision support systems to the reader.
Summary of Insights
To navigate through the complexities of decision-making, practitioners must first acknowledge the fundamental components of DSS: database management, model base management, and the user interface design. These elements collectively ensure that the right information gets to the right people at the right time. The article has illuminated how various types of DSS—ranging from data-driven models to knowledge-based systems—each contribute unique strengths and capabilities.
"An informed decision, underpinned by a robust DSS, is arguably the most significant factor in achieving business success in the 21st century."
Furthermore, the discussion on methodologies such as data mining and predictive analytics highlighted not only the relevance but also the adaptability of DSS in accommodating evolving business strategies. The ethical dimensions around bias in algorithms and accountability also stand as critical considerations. These insights serve as a guiding compass for organizations as they invest in decision support technologies.
Looking Ahead
As we peer into the future, several trends begin to emerge that will shape the landscape of decision support systems. The integration of AI and Machine Learning will enhance the ability of DSS to predict outcomes and recommend actions. Real-time decision-making capabilities are no longer just a want, but a need for businesses looking to stay ahead in a fiercely competitive environment.
Additionally, organizations need to prepare for the challenges posed by rapid technological advancements. This includes the need for ongoing training to minimize user resistance and ensuring that data security is tightly managed.