Harnessing Predictive Analytics in Retail: Key Examples
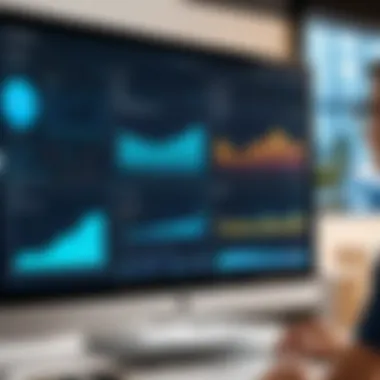
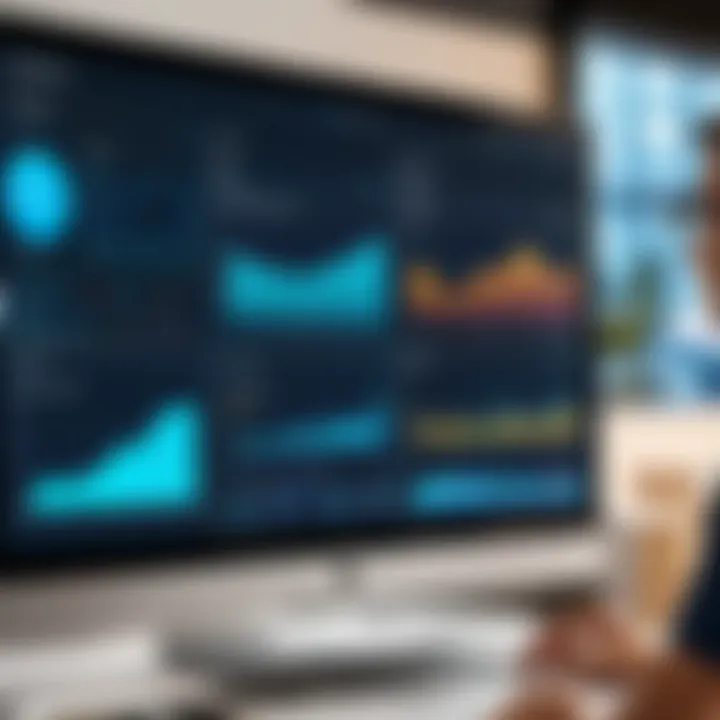
Intro
Predictive analytics has carved a significant niche within the retail sector, enabling companies to leverage vast amounts of data to inform their business strategies. Its fundamental objective is to predict future outcomes based on historical data. This process relies on statistical algorithms and machine learning techniques, allowing retailers to make more informed decisions about their operations. As the market continues to evolve, embracing predictive analytics becomes essential for retail organizations aiming to stay competitive.
This article delves into the multifaceted applications of predictive analytics in retail. By mapping out real-world scenarios, readers will grasp how businesses effectively utilize data analytics to enhance customer experience, streamline inventory management, and forecast market trends. Consequently, it sets the stage for understanding the vital role predictive analytics plays in transforming retail practices.
Software Overview
Software Category and Its Purpose
Predictive analytics software embodies a specialized category designed to empower businesses with foresight and informed decision-making capabilities. Its primary purpose is to analyze historical data to identify patterns that can predict future performance. This application can significantly impact various aspects like marketing, sales forecasting, and customer relationship management. Companies that implement predictive analytics can enhance their operational efficiency and effectiveness.
Key Features and Functionalities
Predictive analytics software encompasses a range of features and functionalities:
- Data Mining: This feature facilitates the extraction of relevant patterns from large datasets, helping retailers uncover insights that would be challenging to detect otherwise.
- Statistical Analysis: Utilizing statistical methods to evaluate data ensures robust forecasts, improving decision-making processes.
- Machine Learning Integration: This enhances the software's adaptability by enabling it to learn from new data, refining its predictions over time.
- Visualization Tools: Clear visual representations of data trends and insights play a crucial role in facilitating understanding among users.
- Scenario Analysis: This allows users to model different business scenarios to see potential outcomes based on varying inputs, guiding strategic planning.
Adopting predictive analytics software presents retailers with opportunities for growth and optimization, thus reinforcing its importance in the current retail landscape.
Prelims to Predictive Analytics
Predictive analytics plays a crucial role in modern retail as businesses strive to understand customer behavior and optimize operations. It utilizes statistical algorithms and machine learning techniques to analyze historical data and identify patterns that can forecast future trends. This capability allows retailers to strategically enhance decision-making processes, providing significant advantages in a highly competitive market.
Definition and Importance
Predictive analytics refers to the practice of using data, statistical algorithms, and machine learning techniques to identify the likelihood of future outcomes based on historical data. In retail, this means leveraging vast amounts of data collected through various channels including sales transactions, customer interactions, and online browsing activities. The importance of predictive analytics cannot be overstated. It empowers retailers to make informed decisions that can directly impact their bottom line. By anticipating customer demands, brands can manage inventory effectively, reduce costs, and improve customer satisfaction. This directly correlates with profitability and operational efficiency, making predictive analytics an indispensable tool.
Key Techniques in Predictive Analytics
Several techniques are employed in predictive analytics in retail. Leveraging each technique appropriately can lead to transformative outcomes. Some of the most relevant techniques include:
- Regression Analysis: This statistical method is used to understand the relationship between variables. For example, retailers can predict sales based on promotional pricing or seasonal trends.
- Time Series Analysis: This involves analyzing data points collected over time to identify trends. Retailers can pinpoint peak shopping times or seasonal purchasing behavior, aiding in inventory and staffing decisions.
- Machine Learning: Algorithms that adapt and learn from new data. For example, retail businesses employ machine learning to provide personalized product recommendations based on customer browsing history and purchase behavior.
- Association Rule Learning: This technique identifies relationships between different items bought together, thus helping retailers create bundled offers or targeted promotions.
The integration of these techniques into retail operations enables a data-driven approach to business strategies, leading to improved customer experiences and successful outcomes.
"The future of retail lies heavily in understanding prediction. Companies that can forecast customer trends will lead the market."
In summary, predictive analytics is not just about forecasting. It represents a paradigm shift in how retailers operate, ensuring that they can respond quickly to changing market dynamics and consumer preferences.
The Role of Predictive Analytics in Retail
Predictive analytics has significantly reshaped the operations within the retail sector. Retailers now leverage advanced data analysis techniques to gain insights into customer behavior, market trends, and inventory management. This section addresses several specific elements that highlight the crucial role predictive analytics plays in enhancing profitability and creating value for both businesses and consumers.
Enhancing Customer Experience
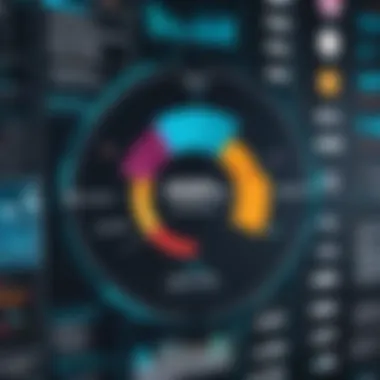
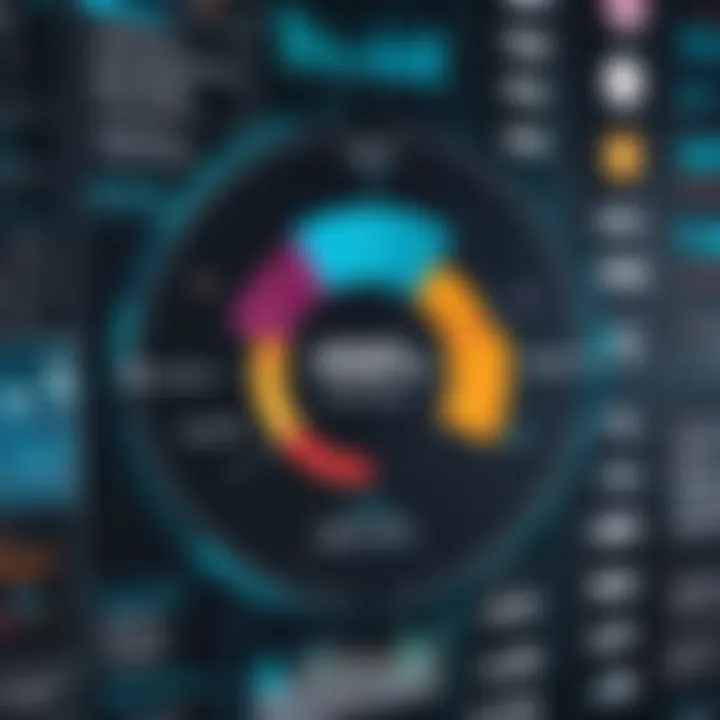
Understanding customer preferences and behaviors is essential for any retail business. Predictive analytics allows retailers to analyze purchasing patterns, demographic data, and even sentiment analysis from social media. By integrating these data sets, companies can tailor their offerings to meet the demands of their consumers. For example, if analysis indicates that a particular demographic is buying more eco-friendly products, the retailer can spotlight these items in promotional materials, improving customer engagement.
Moreover, personalized recommendations created by predictive analytics can significantly enhance the shopping experience. Businesses like Amazon utilize algorithms to suggest products based on previous purchases, which keeps customers returning for more. This ability not only enhances satisfaction but can also improve conversion rates, leading to better business outcomes.
Optimizing Inventory Management
Inventory management is another critical area where predictive analytics excels. Retailers face the challenge of maintaining optimal stock levels without overstocking or running out of popular items. By utilizing predictive analytics, businesses can forecast demand with precision, helping to minimize wastage and costs associated with excess inventory.
Software solutions from companies like SAP and Oracle provide tools that offer predictive capabilities. They analyze historical sales data and market conditions to help businesses anticipate demand spikes or declines. This insight allows retailers to make more informed purchasing decisions, ensuring a balance between supply and demand, and enhancing operational efficiency.
Forecasting Sales Trends
Accurate sales forecasting is fundamental for strategic planning. Retailers can leverage predictive analytics to analyze seasonal trends, customer purchasing behaviors, and broader market movements. By doing so, companies like Walmart can prepare for fluctuations in demand, optimizing their resources and aligning marketing efforts accordingly.
Sales trend predictions help businesses adjust their product offerings, marketing strategies, and staffing needs well in advance. Retailers also use this data to strategize promotional campaigns, ensuring they align with the predicted buying patterns of their customers. This proactive approach enhances overall business stability and positions firms better to meet market demands.
"Predictive analytics transforms retail operations, enabling informed decisions and enhanced efficiency across various facets of the retail experience."
Real-World Examples of Predictive Analytics in Retail
The role of predictive analytics in retail is not merely theoretical. It finds expression in various real-world applications that enhance operational capabilities and improve customer experiences. This section highlights seminal examples of how major retailers utilize predictive analytics to achieve remarkable results. These case studies illustrate the transformative power of data-driven decision-making, demonstrating tangible outcomes that can influence businesses significantly.
Target's Inventory Optimization
Target has notably harnessed predictive analytics to optimize its inventory management. By analyzing historical sales data and integrating it with local demographic information, Target can anticipate demand more accurately. This helps the company maintain optimal stock levels, reducing excess inventory while ensuring that in-demand products are always available. An example of this approach is when Target predicted the needs of specific neighborhoods based on customer purchase patterns. As a result, the company experiences fewer stockouts and increased sales, driving overall efficiency in operations.
Walmart's Demand Forecasting
Walmart employs predictive analytics not just for inventory, but to forecast customer demand accurately. By using a combination of sales data, seasonality trends, and macroeconomic indicators, Walmart can create detailed forecasts for various products across its vast network of stores. This predictive capability allows for precise stocking of items, improving customer satisfaction. During peak shopping seasons, Walmart effectively uses these analytics to ensure sufficient supply meets anticipated demand, enhancing operational performance and boosting bottom lines.
Amazon's Recommendation System
Amazon's recommendation system exemplifies the power of predictive analytics in enhancing customer engagement. By analyzing purchase history, browsing behavior, and customer reviews, Amazon delves into complex databases to suggest personalized product offerings. This not only improves the shopping experience by helping customers discover relevant products but also significantly boosts sales through cross-selling and upselling. The algorithm can adapt in real-time, allowing Amazon to present recommendations tailored to individual users, proving that personalized experiences can drive higher conversion rates.
Sephora's Personalized Marketing Strategies
Sephora utilizes predictive analytics to refine its marketing strategies to meet consumer preferences. By leveraging customer data from various sources, including loyalty programs and social media interactions, Sephora can identify trends and customer sentiments. This information is then used to tailor marketing campaigns, ensuring they resonate with target audiences. For example, by predicting which products may appeal to individual customers, Sephora can effectively deliver personalized email and online promotions, thus enhancing customer retention and engagement.
Macy's Price Optimization Techniques
Macy's employs predictive analytics for dynamic price optimization. Through comprehensive analysis of market trends, historical sales data, and competitor pricing, Macy's can adjust prices in real-time to optimize sales and profit margins. This method allows the retailer to be responsive to market conditions, offering promotions when needed or maintaining higher prices for in-demand products. The outcome is improved sales performance while ensuring that pricing strategies remain competitive and aligned with consumer expectations.
"Real-time adaptation in pricing based on predictive analytics reflects a retailer's ability to use data effectively to respond to market dynamics."
Through these examples, it is clear that predictive analytics is integral to retail strategy. By leveraging data for decision-making, retailers can not only enhance their operations but also foster deeper connections with their customers. Each retailer's distinct approach exemplifies the flexibility and necessity of predictive analytics in todayโs competitive market.
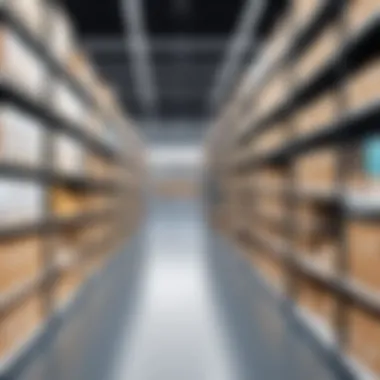
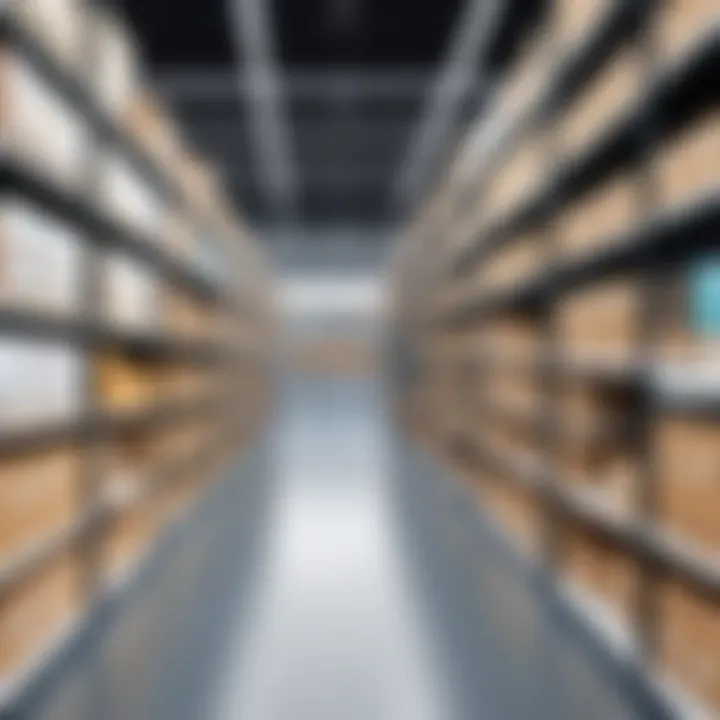
Benefits of Implementing Predictive Analytics in Retail
Predictive analytics is not merely a trend in the retail sector; it is a fundamental shift in how organizations approach decision-making, customer interactions, and operational efficiency. The benefits of implementing predictive analytics in retail are multi-faceted. Organizations that use predictive analytics can derive strategic advantages. These benefits range from informed decision-making to increased revenue and enhanced customer retention. By employing data-driven insights, businesses transform their operations and customer experiences.
Informed Decision Making
Informed decision-making is a powerful benefit achieved through predictive analytics. Retailers can analyze past sales data, market trends, and customer behaviors to better anticipate future outcomes. This capability enables companies to pivot their strategies based on insightful data rather than relying on gut feelings.
With the right tools, businesses can access dashboards that visualize complex data trends. For example, if a retailer identifies a consistent drop in sales during specific months, they can adjust their marketing efforts or inventory strategies accordingly.
Most importantly, informed decision-making leads to precision. It eliminates guesswork, allowing retail leaders to create accurate plans and allocate resources efficiently. As a result, businesses respond agilely to market fluctuations.
Increased Revenue and Profitability
Another significant advantage of predictive analytics is its potential to enhance revenue and profitability. Retailers can optimize pricing strategies using data insights about customer spending habits, seasonal trends, and competitor pricing. For example, Macy's uses price optimization techniques effectively by analyzing sales patterns to establish attractive price points.
By implementing dynamic pricing models, retailers can adjust prices in real-time based on demand projections. This not only attracts more customers but also maximizes profit margins. When smart analytics align products with the right price at the right moment, sales naturally increase.
Moreover, predictive analytics can assist with inventory management. By forecasting demand accurately, retailers avoid overstocking or stockouts, both of which can harm revenue. Therefore, the financial benefits of predictive analytics extend beyond mere sales increases; it encompasses overall profitability.
Enhanced Customer Retention
Lastly, enhanced customer retention stands out as a vital benefit of predictive analytics. Understanding customers on a granular level allows retailers to develop targeted marketing strategies. Threading data analytics into customer relationship management leads to more personalized experiences.
For example, Amazon's recommendation system exemplifies this benefit. By analyzing past purchases and browsing habits, the platform presents suggestions that resonate with individual customers. This tailored approach makes customers feel valued, encouraging ongoing engagement and loyalty.
In addition, predictive techniques can identify at-risk customers. Algorithms can analyze behavior patterns that may indicate a likelihood of churn. Recognizing these customers allows retailers to enact retention strategies in advance, preserving long-term relationships.
"Predictive analytics provides the insights necessary for a strategic advantage in the competitive retail landscape."
Challenges in Adopting Predictive Analytics
The incorporation of predictive analytics in retail is not without its challenges. Retailers face various obstacles that can hinder effective adoption. Recognizing these challenges is crucial, as they influence the overall success of implementing predictive analytics. Understanding the potential pitfalls allows organizations to develop strategies that mitigate risks and maximize opportunities in the use of data to enhance retail operations.
Data Quality and Integration Issues
One of the most significant challenges retailers encounter is ensuring the quality of their data. Data quality directly affects the models and insights derived from predictive analytics. Inaccurate or incomplete data can lead to misleading predictions and poor decision-making. For example, sales data might be recorded differently across various channels, complicating the overall analysis.
Additionally, data integration remains a critical issue. Retailers often collect data from various sources, including point-of-sale systems, customer interactions, and online platforms. Integrating these disparate data sources into a coherent and usable format is essential to create a holistic view of operations. Failure to do so can result in fragmented insights, making it hard to draw reliable conclusions.
Skill Gap in Analytics
Another challenge relates to the skill gap present in many organizations. While predictive analytics can provide significant benefits, not all retail professionals are equipped with the necessary skills to leverage these tools effectively. Many retailers lack personnel who understand data science, statistical analysis, and machine learning methods.
Training existing staff or hiring specialists can be resource-intensive and may not be feasible for all businesses. As a result, companies might struggle to develop robust predictive models or interpret the data they generate accurately. Addressing this skill gap is vital for retailers to make the most out of their investment in predictive analytics.
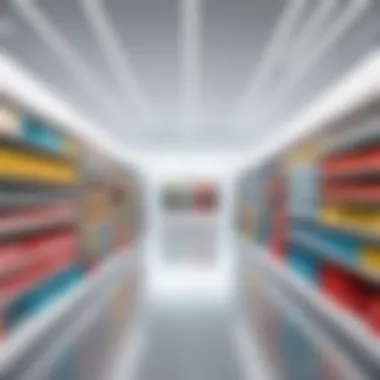
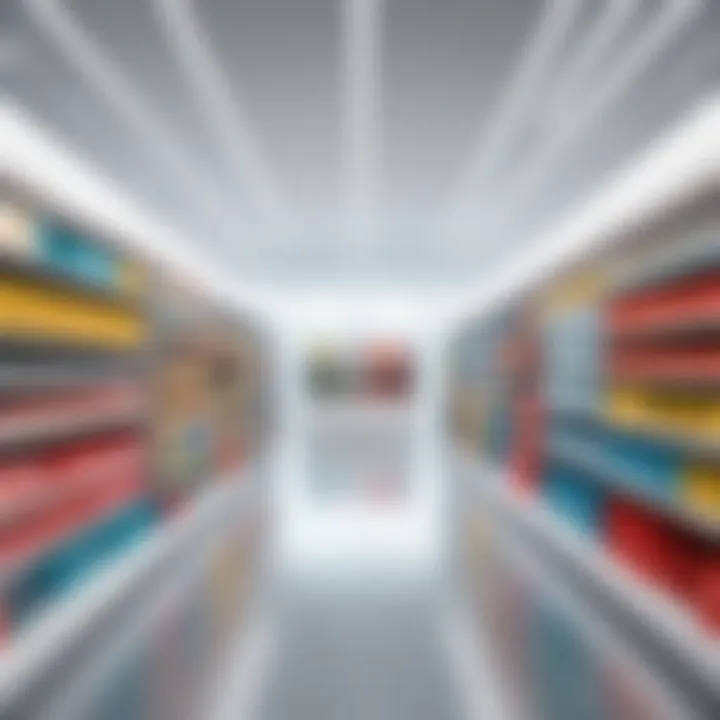
Privacy Concerns with Data Usage
Privacy concerns are a pervasive challenge in the retail sector. Customers are increasingly aware of how their data is used, leading to heightened expectations for transparency and data security. Retailers must navigate complex regulations surrounding data usage, such as GDPR in Europe or CCPA in California, which mandate that organizations protect customer data and use it responsibly.
Failing to address privacy concerns can result in reputational damage and legal consequences. Retailers need to establish robust data governance frameworks that protect consumer privacy while still allowing for the effective use of data in predictive analytics.
In summary, while predictive analytics presents numerous opportunities for retail, the challenges of data quality, skill gaps, and privacy must be carefully managed to harness its full potential.
Future Trends in Retail Predictive Analytics
The retail sector stands on the brink of significant transformation due to ongoing advancements in predictive analytics. The future is not just about analyzing past data but also about anticipating future possibilities. This section will explore essential trends in predictive analytics that are shaping how retailers operate and engage with customers. These trends highlight crucial elements like integration of technology, the rise of real-time analytics, and increased personalization strategies.
Integration of Artificial Intelligence
Artificial Intelligence (AI) is one of the most transformative elements in predictive analytics. Retailers are increasingly employing AI to analyze large datasets quickly and with greater accuracy. By implementing machine learning algorithms, businesses can uncover patterns and trends that were previously hidden. This integration allows for more sophisticated forecasting methods that improve inventory management and demand prediction.
With AI, the capability to process unstructured data, such as customer reviews or social media insights, enhances decision-making processes. Retailers can respond to market changes more swiftly. Moreover, AI-driven chatbots improve customer service by offering personalized recommendations based on previous buying behavior, thus driving sales conversions.
Expansion of Real-Time Analytics
The demand for real-time analytics is becoming paramount in the retail world. Customers expect immediate responses to their needs, which requires retailers to have access to up-to-the-minute data. Real-time analytics enables businesses to monitor shopper behavior as it happens, allowing for adaptive marketing strategies that can change in the moment.
This capability leads to more responsive inventory management. Retailers can assess stock levels in real time, preventing stockouts or overstock situations. Furthermore, decision-makers can adjust promotional offers based on live customer interactions. As a result, businesses not only improve customer satisfaction but also enhance operational efficiency.
Personalization Through Advanced Analytics
Personalization remains a key trend that retailers can no longer overlook. Advanced analytics allows retailers to segment their customer base more effectively. By analyzing customer interactions across different channels, retailers can tailor their marketing strategies to match individual preferences. This personalized approach generates a sense of connection between the brand and the customer, leading to higher engagement and loyalty.
Predictive analytics tools assist in customizing offerings on an individual level, enhancing customer experiences significantly. For instance, retailers like Amazon employ this strategy to suggest products based on browsing history and past purchases. Further, this tactic can extend to personalized email marketing campaigns, increasing the likelihood of conversions.
"The ability to predict customer behavior empowers retailers to position themselves strategically in a competitive market."
As retailers embrace these future trends, predictive analytics will become more critical in refining their approaches to customer engagement and operational processes. The smart integration of technologies and techniques will set the tone for a new era in retail.
Ending
In this article, we have explored the vital role of predictive analytics in the retail sector. The insights show that leveraging data can significantly impact core business functions. It helps organizations understand customer preferences, manage inventory, and forecast sales accurately. The effective use of predictive analytics not only enhances operational efficiency but also improves the overall customer experience.
Summary of Key Insights
Predictive analytics is not just a trend; it represents a fundamental shift in how retail operates. Some key insights identified throughout the article include:
- Data-Driven Decisions: Retailers increasingly rely on data to drive strategies, minimizing guesswork.
- Customer-Centric Approaches: Businesses prioritize personalization, aiming to meet individual customer needs.
- Efficiency Gains: Optimizing inventory management reduces excess stock, contributing to lower costs.
- Forecasting Accuracy: Advanced techniques enable better predictions of market dynamics, allowing businesses to adapt swiftly.
These elements collectively demonstrate how predictive analytics serves as a powerful tool in modern retail.
Implications for Retail Businesses
The implications for retail businesses are profound. Firstly, adopting predictive analytics can lead to enhanced profitability through informed decision-making. Companies like Amazon and Target showcase the direct benefits when data is effectively utilized.
Moreover, retailers must consider the continual evolution of technology. Remaining aware of advancements in artificial intelligence and machine learning is crucial. This awareness can further enhance predictive capabilities.