Exploring Statistical Analytics: Methods and Impact
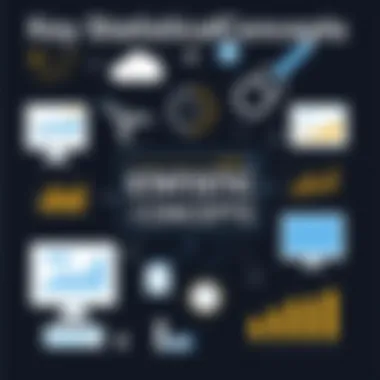
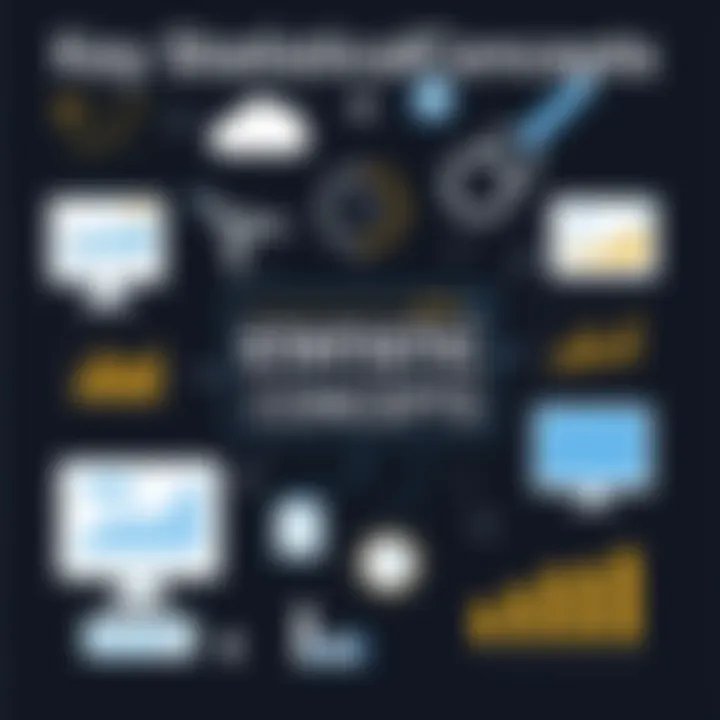
Intro
Statistical analytics stands as a cornerstone in the data-centric world. It embodies a systematic approach to understanding and interpreting dataâa skill set that has transformed decision-making processes across industries. Whether it's deciphering consumer behaviors, predicting market trends, or driving operational efficiencies, the relevance of statistical tools cannot be overstated.
In an arena where data is often described as the new oil, statistical analytics provides the refinery. Through meticulous examination and thoughtful exploration of data, we can extract insights with profound implications. As we dive deeper into this article, we will unravel the methodologies that shape statistical analytics, the tools that facilitate this journey, and how these elements coalesce to influence business strategy.
Besides bridging foundational concepts, we will look at the technological advancements that have reshaped analytical capabilities. The insights gained from statistical analytics are not just numbers; they represent stories about patterns and behaviors, illuminating paths previously obscured. As we consider future trends in this field, we aim to prepare professionals for the evolving landscape of data interpretation.
Understanding Statistical Analytics
Statistical analytics is an invaluable tool in todayâs data-driven landscape, offering insights that help businesses and organizations make informed decisions. The importance of understanding statistical analytics extends beyond basic numerical understanding. Instead, it melds data interpretation with practical application. As industries grapple with massive data influx, the ability to analyze and extract meaningful patterns is crucial. It provides a framework for understanding variances, trends, and relationships within data sets.
When one comprehends statistical analytics, it opens doors to a myriad of applications. From predicting customer behavior in retail to analyzing patient outcomes in healthcare, the benefits of these analytical methods can be profound. The most compelling aspect of statistical analytics is its ability to bridge the gap between raw data and actionable insights, thus refining decision-making processes across various sectors.
Incorporating statistical analytics into strategic planning can lead to substantial gains in performance accuracy and efficiency. However, a keen understanding of its core principles is necessary. Knowing how to correctly interpret data can safeguard against common pitfalls, like confirmation bias or misread statistical significance.
"Statistical analytics transforms raw numbers into actionable insights that can shape the future of industries."
Definition and Purpose
Statistical analytics involves the systematic application of statistical methods and tools to analyze data. At its core, it aims to discover underlying patterns or trends that inform decisions. The purpose of statistical analytics is multifold: understand market dynamics, evaluate outcomes of interventions, and forecast future trends. In essence, it acts as a compass, guiding businesses through the often turbulent waters of data.
A concise definition might align statistical analytics with descriptive statistics, inferential statistics, and predictive analytics. Each serves its purpose when it comes to extracting insights from vast amounts of information. Descriptive statistics reflects basic characteristics, while inferential statistics draws conclusions about populations based on sample data. Predictive analytics uses patterns in data to forecast outcomes, providing a crucial advantage in strategic planning.
Understanding the purpose behind each analytical method empowers users to choose the right techniques for their specific scenarios. The definition and purpose underpin every application of statistical analysis in real-world situations, underscoring its relevance in decision-making processes today.
History and Evolution
History has been kind to statistics, tracing roots back to ancient civilizations, with early records in Mesopotamian clay tablets. However, it wasn't until the Renaissance and the Enlightenment that the discipline of statistics began to evolve into a more structured realm of study. Early statisticians focused mainly on demographic data collection, shaping what would become a vital element in governance and economics.
As time moved forward, the 19th and 20th centuries ushered in significant advancements. The development of key statistical methods, such as regression analysis by Sir Francis Galton, marked a major turning point. These breakthroughs laid the groundwork for modern statistical analytics. The advent of computers and software drastically accelerated this evolution, allowing for complex calculations on a scale previously unimaginable.
More recently, the growth of big data and machine learning has propelled statistical analytics to new heights. Businesses leverage large data sets not just for descriptive insight but for predictive and prescriptive analytics as well. Now, analytical models infuse everyday decision-making in diverse fields from finance to sports analytics. The path to todayâs sophisticated statistical analytics has been long and multifaceted, reflecting the ever-growing needs of data in the modern era.
Core Concepts in Statistical Analytics
Statistical analytics serves as the backbone of insightful data interpretation. It allows analysts to distill vast amounts of raw data into understandable and actionable insights. In any endeavor, grasping the core concepts is not just an academic exercise; itâs a vital skill that directly influences successful outcomes. Understanding these elements can streamline decision-making, improve predictions, and ultimately lead to more precise strategies, whether in business, healthcare, or social sciences.
Descriptive Statistics
Descriptive statistics provides a foundational overview of the data at hand. Its main purpose is to summarize and describe the key features of a dataset, which in turn gives context for further analysis.
Measures of Central Tendency
When you talk about measures of central tendency, you're diving into statistics that attempt to find the center of a dataset. It includes mean, median, and mode. The beauty of these measures lies in their ability to provide a quick snapshot of the data distribution. For example, businesses often depend on the average sales figures (mean) to gauge performance.
- Key Characteristic: These measures simplify complex data into a single number.
- Benefits: It helps in making quick decisions or offering insights into general trends.
- Unique Feature: While the mean might give a fair representation, it can be misleading in the presence of outliers. Thus, the median often comes into play when data lacks normal distribution.
Though beneficial, one must be cautious when using these metrics without considering the underlying data characteristics.
Measures of Dispersion
While measures of central tendency provide a focal point, measures of dispersion expose the spread or variability of the data. These include range, variance, and standard deviation. They help analysts understand how much the data points deviate from the central value, which is a critical aspect in many analyses.
- Key Characteristic: Dispersion metrics highlight data variability, revealing how consistent or scattered the data is.
- Benefits: Knowing the dataâs spread aids in risk assessment and resource allocation in businesses.
- Unique Feature: The standard deviation offers an intuition about how spread out the data points are relative to the mean, which can unearth underlying patterns.
However, while they provide essential context, these measures can also obscure important features if the data is not sufficiently examined.
Data Visualization Techniques
Data without context can be perplexing; that's where data visualization techniques come into the picture. Techniques such as histograms, pie charts, and box plots offer visual representations that can drastically enhance understanding. Data visualization is pivotal because it transforms numbers into stories, making it easier to grasp the overall trends and patterns at a glance.
- Key Characteristic: Effective visualizations convey complex data in a simpler manner, making them more accessible.
- Benefits: Visuals can reveal trends that might be lost in raw figures, driving home critical points to stakeholders.
- Unique Feature: Interactive dashboards allow users to drill down into specific data points, providing tailored insights.
That said, the choice of visualization should align with the data's nature and the audience's needs lest it complicate rather than clarify.
Inferential Statistics
Diving into inferential statistics moves beyond mere description; it allows analysts to make predictions or generalizations about a population based on a sample. This method is particularly valuable in hypothesis-driven research, where a researcher wants to test assumptions over a larger group.
Hypothesis Testing
Hypothesis testing serves as a systematic approach to evaluating claims. It often utilizes a null hypothesis to assert that there's no effect or difference, making a case for the opposite claim through statistical grounds. For example, a company might test if a new marketing strategy boosts sales.
- Key Characteristic: It provides a framework for us to test assumptions with quantifiable evidence.
- Benefits: It enables decision-makers to rely on data rather than assumptions, which reduces the likelihood of biases affecting outcomes.
- Unique Feature: The p-value associated with hypotheses helps determine the strength of evidence against the null hypothesis.
Though robust, practitioners must remember that failing to reject the null hypothesis doesnât confirm itâs true; it just highlights the insufficiency of evidence.
Confidence Intervals
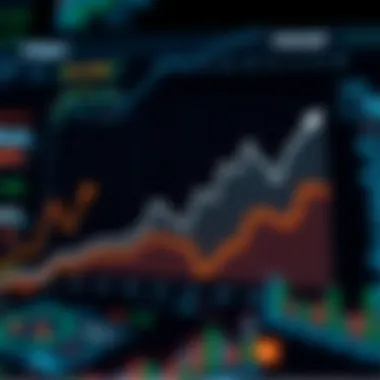
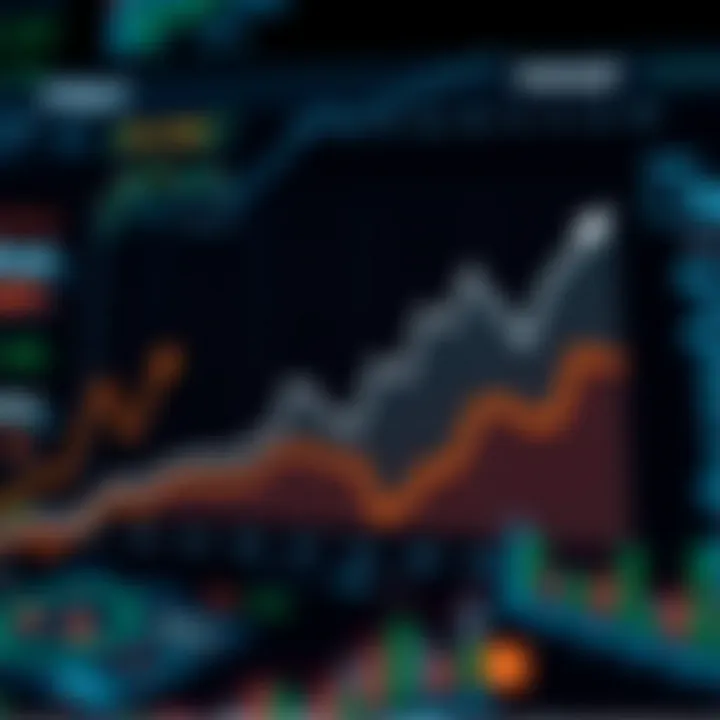
Confidence intervals are another integral concept, providing a range within which we expect the true population parameter to lie. They offer a means to express the uncertainty associated with an estimate, a nuance that can be critical when presenting findings to stakeholders.
- Key Characteristic: It gives not just a point estimate but a range, allowing for uncertainty to be expressed.
- Benefits: Better informs risk assessments and makes decision-making more transparent by illustrating potential variability.
- Unique Feature: The width of the interval directly correlates with sample size and confidence level; a larger sample can lead to tighter, more reliable intervals.
However, these intervals hinge on sample randomness and can mislead if the assumptions are not met or if the sample size is too small.
Regression Analysis
Regression analysis is a powerful statistical technique that allows one to investigate the relationship between variables. Often, this forms the basis for predictive modeling, as it helps to ascertain how the dependent variable changes when any of the independent variables are varied.
- Key Characteristic: It quantifies the relationship, showing how changes in one variable might affect another.
- Benefits: Businesses utilize regression to forecast sales based on multiple factors, such as advertising spend or market trends.
- Unique Feature: The capability to adjust for multiple variables simultaneously sets regression analysis apart from simpler predictive methods.
Still, analysts need to be cautious of misapplying this technique, as incorrect assumptions can lead to misguided conclusions.
Key Tools and Software for Statistical Analytics
Statistical analytics hinges heavily on the tools and software that empower professionals to analyze data effectively. Whether it's for crunching numbers in a business setting or conducting research in social sciences, the right software can make a world of difference. This section aims to shed light on various statistical tools and their unique contributions to this field. Understanding these tools is crucial because they provide the means to turn raw data into actionable insights that drive decision-making processes.
Statistical Software Packages
R Programming
R Programming has carved out a niche among statisticians and data analysts. This open-source language is renowned for its versatility and robust statistical capabilities. One of the standout characteristics of R is its expansive package ecosystem, where users can access various statistical techniques and graphical techniques almost effortlessly.
The community supporting R is vast and vibrant. Users often share and develop packages that enhance its functionality. This makes R a favored choice for statistical modeling, complex calculations, and data visualization. However, newcomers might find the learning curve steep, as its syntax can be intimidating compared to more straightforward programming languages. Nonetheless, the customizability R offersâespecially in crafting specific analysesâis hard to beat.
Python Libraries
Exploring the realm of Python Libraries, one finds a treasure trove of tools tailored for statistical analysis. With libraries such as Pandas, NumPy, and SciPy, Python has made a strong case for itself in the statistical analytics field. What's appealing about Python is not just its straightforward syntax but also its ability to integrate seamlessly with various other technologies, like web applications.
The critical characteristic of Python Libraries lies in their general-purpose nature. They cater to data manipulation, numerical analysis, and even continuous statistical modeling. One unique feature of these libraries is how well they support integration with machine learning frameworks. However, given the breadth of Python's capabilities, users may feel overwhelmed by the sheer volume of options at their disposal.
SPSS and SAS
Focusing on SPSS and SAS, these are industry giants in statistical software. SPSS is particularly user-friendly, often preferred in academic settings for its intuitive interface, which simplifies complex statistical analyses. Conversely, SAS, while also offering impressive functionality, is renowned for its robustness in handling large datasets and advanced analytics.
A significant advantage of SPSS is its drag-and-drop interface, catering well to those who may not have extensive programming knowledge. However, its flexibility is somewhat limited compared to programming-centric tools like R and Python. SAS, on the other hand, provides an extensive range of features but can be pricey and may pose accessibility challenges for smaller organizations.
Emerging Analytical Tools
As the landscape of data evolves, so do the tools designed to handle it. Emerging Analytical Tools are breaking new ground and helping professionals adapt to ever-changing demands. These tools enhance the capabilities of traditional software, presenting new methods for interpretation and application of statistical analysis.
Data Mining Tools
Data Mining Tools become a focal point for extracting valuable information from vast datasets. These tools utilize various algorithms to identify patterns and relationships that often remain hidden in raw data. A distinguishing feature of data mining is its ability to predict outcomes based on earlier trends, making it invaluable in marketing and finance.
One significant benefit of these tools is their automation capabilities, facilitating quicker analysis. However, users should be wary of over-relying on these tools without proper context, as algorithms may identify correlations that do not imply causation.
Machine Learning Frameworks
In the context of Machine Learning Frameworks, the complexity of statistical analysis takes a quantum leap. Such frameworks complicate the analytics process as they apply statistical techniques to enable systems to learn from data. This characteristic makes them essential for predictive analytics, particularly in sectors like finance and healthcare.
A unique feature of machine learning frameworks, like TensorFlow or PyTorch, is their ability to improve model accuracy over time as more data becomes available. However, the downside could be their resource intensity; running sophisticated algorithms might require substantial computational power and technical expertise, which can be a barrier for smaller organizations.
Visualization Platforms
Finally, looking at Visualization Platforms, the significance of data representation in statistical analytics cannot be overstated. These platforms allow users to convey complex data insights visually, making interpretations easier for a broader audience. Tools like Tableau and Power BI democratize data by transforming raw numbers into understandable visuals.
Highlighting trends and patterns through visual means enhances the storytelling aspect of data analysis. Yet, while these platforms excel in visual appeal, caution is necessary; oversimplifying data for aesthetic reasons can lead to misinterpretations.
Statistical analytics relies heavily on these tools and platforms to synthesize and interpret complex data efficiently. Knowledge of these tools and their unique features equips professionals with the means to extract meaningful insights from data, enabling strategic decision-making across diverse fields.
Applications of Statistical Analytics
Understanding the real-world implications of statistical analytics is critical for professionals across various fields. This section examines its pivotal role and how it drives informed decision-making across multiple domains.
Business Decision-Making
Market Analysis
Market analysis is like the compass guiding businesses toward understanding their landscape. It involves examining data to identify trends, customer preferences, and market conditions. A key characteristic of market analysis is its ability to provide actionable insights. Companies can leverage this information to refine their marketing strategies, effectively target their audience, and ultimately boost profitability. Notably, a unique aspect of market analysis is its predictive capabilityâusing historical data to forecast future trends. This isn't without its challenges; misleading data can lead to incorrect interpretations, but when done right, the benefits far outweigh the drawbacks.
Risk Management
In the increasingly volatile business environment, risk management stands as a linchpin in ensuring sustainability. Using statistical analytics, organizations can identify, evaluate, and mitigate risks effectively. A standout feature is its systematic approach to quantify risks, allowing companies to make data-driven decisions rather than gut feelings. This analytical framework not only enhances decision-making but also promotes a culture of safety within organizations. However, while it's insightful, there can be a tendency for over-reliance on statistical predictions, which might overlook qualitative factors that could impact risk.
Performance Measurement
Measuring performance accurately is fundamental for any business seeking growth. Statistical analytics plays a crucial role here, providing metrics that help assess efficiency, productivity, and outcomes. The cornerstone of performance measurement lies in its focus on key performance indicators (KPIs) that drive business activities. With tools like dashboards, organizations can visualize real-time performance data, leading to sharper insights. Yet, while this approach is powerful, it can often lead to an overwhelming amount of dataâfiguring out whatâs truly relevant can be a daunting task.
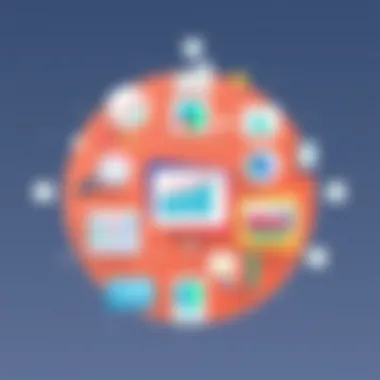
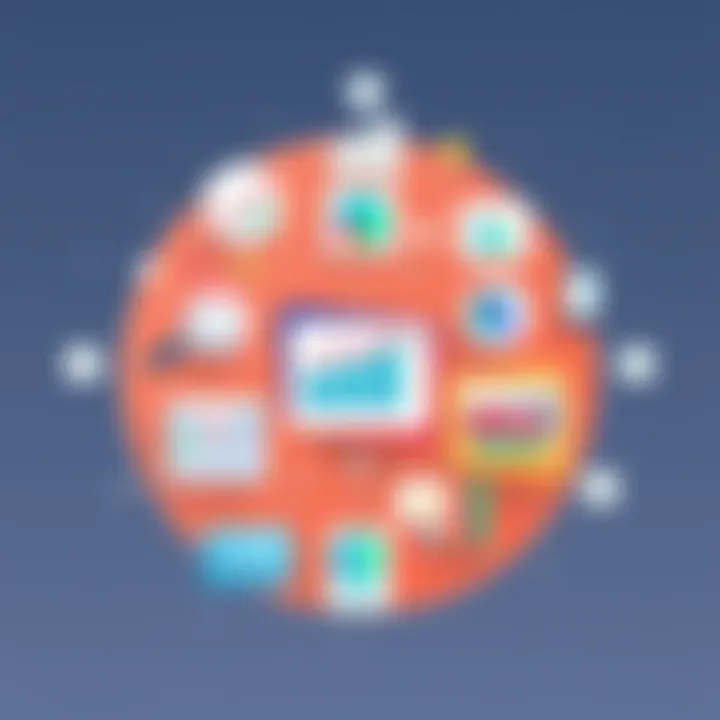
Healthcare and Medicine
Clinical Trials
Clinical trials are the backbone of medical advancements. Using statistical analytics, researchers can assess the efficacy and safety of new treatments or medications. A striking aspect of clinical trials is their structured approach to gathering and analyzing data from diverse participant groups. This method not only ensures robustness but also enhances reproducibility in research. However, the complexity of these trials raises ethical concerns, particularly regarding participant consent and data handling.
Epidemiological Studies
In the quest for public health improvement, epidemiological studies wield significant power. By analyzing health data trends, these studies help identify disease patterns and risk factors across populations. A defining characteristic of epidemiological research is its potential for broad applicationâinsights derived can influence policy and prevention strategies. Despite this, challenges exist, as confounding variables can distort findings, making careful design and analysis crucial.
Healthcare Operations
Using statistical analytics in healthcare operations enhances service delivery and operational efficiency. It helps streamline processes, manage resources, and improve patient satisfaction. One unique feature is predictive analytics, which can forecast healthcare demands based on current trends, allowing for proactive resource allocation. Nevertheless, while these insights are advantageous, reliance solely on data analytics can sometimes lead to neglect of the human aspects of care.
Social Sciences Research
Survey Methodology
Surveys are a popular tool for gathering data in social sciences. They allow researchers to obtain insights from diverse populations quickly. A key attribute of survey methodology is its versatilityâsurveys can be tailored to extract various types of information, from opinions to behaviors. Despite their widespread use, surveys can be susceptible to biases in responses, making the design phase vital to success.
Demographic Studies
Demographic studies help us understand the characteristics of populations. By analyzing data such as age, gender, income, and education, these studies reveal trends and inform public policy. A defining characteristic of demographic analysis is its ability to spot shifts in population dynamics. However, one must be cautious with overgeneralizations, as demographic data may not present the full picture of societal nuances.
Behavioral Analysis
Understanding behavior is essential in numerous fields, from marketing to psychology. Behavioral analysis employs statistical techniques to decipher patterns in human actions. What stands out is its application for predicting future behaviors based on past actions. Even so, while this approach can be groundbreaking, it's important to remember that human behavior is complex and not always predictable solely through data.
Harnessing statistical analytics in its various applications serves as a catalyst for informed decision-making and innovative solutions across diverse fields. By staying attuned to the analytics landscape, professionals can navigate complexities and seize opportunities more effectively.
Challenges in Statistical Analytics
Statistical analytics is powerful in shaping decisions, but it doesn't come without its hurdles. Addressing the challenges intrinsic to statistical analytics is crucial to optimize its effectiveness and reliability. These challenges can stem from several sources, whether it's the quality of the data being analyzed, the interpretation of the results, or the communication of those findings. Tackling these hurdles head-on can significantly enhance the quality of analyses and, by extension, the decisions based on them.
Data Quality Issues
Data quality can make or break the accuracy of statistical insights. Poor-quality data leads to faulty conclusions. Thus, professionals must navigate several specific aspects of data quality issues.
Handling Missing Data
Missing data is an issue every analyst has to face at some point. Itâs the absence of values that are supposed to be in the dataset, which can skew results and lead to unreliable conclusions. A key characteristic of handling missing data is recognizing when values are absent and understanding the reason behind it. Ignoring this can lead to a distorted view of reality.
Common methods for addressing missing data include:
- Deletion: Remove any records with missing information, although this can reduce sample size.
- Imputation: Estimate and fill in missing values using averages or predictive models, preserving the dataset's integrity.
The advantage of imputation is that it allows for a more comprehensive analysis. However, being overly reliant on it without understanding the missingness can lead to misleading information.
Dealing with Outliers
Outliers are those pesky data points that seem to stray far from the norm. They can either represent genuine anomalies or signal errors in data entry. The challenge is determining which is which. Detecting and deciding how to treat outliers can play a critical role in maintaining the validity of statistical analyses.
Some methods to handle outliers include:
- Capping: Limiting the values of outliers to a certain range can smooth the data.
- Transformation: Sometimes applying logarithmic or square-root transformations helps in reducing their impact.
The uniqueness of dealing with outliers lies in their dual nature. Properly managed, they can reveal critical insights about data distribution. Ignoring them outright could mask potentially informative trends or challenges.
Ensuring Data Integrity
Data integrity refers to the accuracy and consistency of data throughout its lifecycle. It is a pivotal characteristic that ensures the reliability of results derived from statistical analytics. Without it, any conclusions drawn can lose their value.
To maintain data integrity, consider:
- Regular audits: Conducting routine checks for data accuracy can help identify discrepancies.
- Access controls: Limiting who can modify data can prevent unwarranted changes.
The unique feature of ensuring data integrity is that it not only safeguards the results but also upholds trust in the analysis. However, extensive security measures can lead to increased complexity in data handling.
Interpreting Results
Once analysis is complete, the real challenge lies in interpreting the results accurately. A misunderstanding here can lead to disastrous conclusions and misinformed decisions.
Misinterpretation Risks
Misinterpretation of statistical results can occur when analysts rush to correlate findings without understanding the underlying factors involved. One significant characteristic of these risks is the possibility of confirming biases, where only supporting evidence is acknowledged while ignoring contradictory data.
Risks stemming from misinterpretation can lead to:
- Incorrect decisions: Choices based on flawed analytics can have significant repercussions.
- Loss of credibility: Continuous misinterpretation can erode trust among stakeholders.
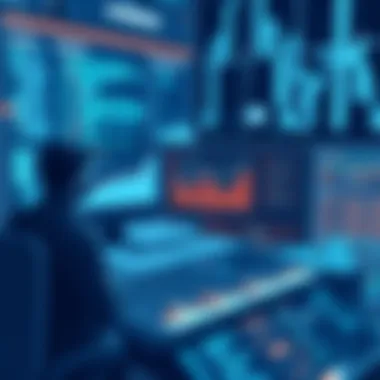
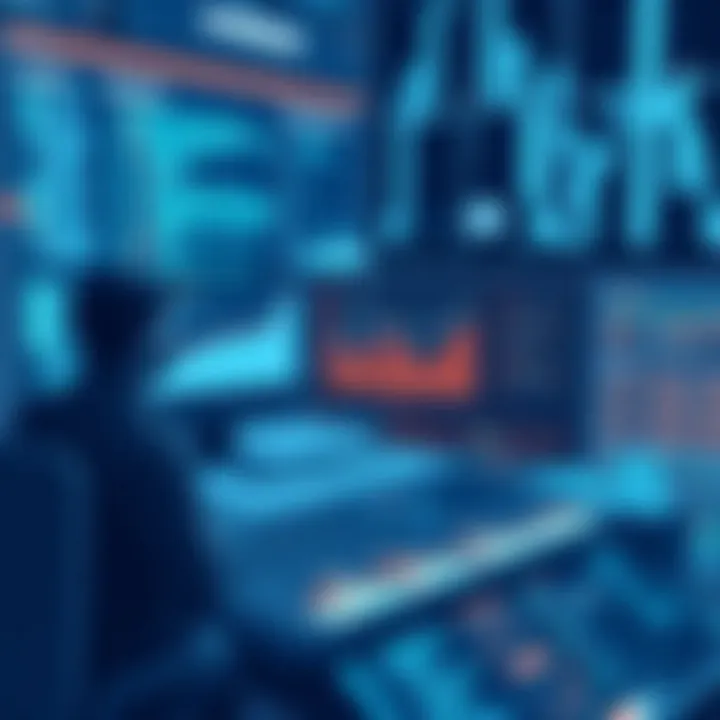
The advantage of recognizing these risks is that it prompts a more thorough approach to analysis and interpretation, ensuring critical thinking prevails.
Statistical Significance vs. Practical Significance
Statistical significance indicates whether a result is likely due to something other than chance. However, just because a result is statistically significant doesn't mean it's practically significant or meaningful in a real-world sense. This distinction is a valuable aspect of statistical analytics that often gets overlooked.
In practice, one might encounter situations where:
- A finding is statistically significant but has minimal real-world impact.
- Practical significance considers the effect size, providing richer context to the number crunching.
Recognizing this difference encourages analysts to dig deeper rather than settle for numbers alone.
Communicating Findings Effectively
Clear and concise communication of findings is the linchpin in ensuring that statistical insights lead to actionable decisions. The key characteristic of effective communication is the ability to translate complex statistics into relatable insights tailored to the audience.
To improve communication:
- Utilize visual aids: Graphs and charts can convey complex data in an easily digestible format.
- Avoid jargon: Using plain language ensures that findings are accessible to stakeholders of varied expertise.
The unique feature of effective communication lies in its potential. Seamlessly sharing findings not only enhances understanding but also promotes informed decision-making. However, oversimplifying results can lead to misunderstandings about the nature and implications of the data.
In statistical analytics, overcoming these challenges is essential to harnessing the full potential of data and fostering reliable insights that drive strategic decisions.
Future Trends in Statistical Analytics
The field of statistical analytics is at a crossroads, facing rapid evolution driven by advancements in technology and changing business needs. Understanding future trends is crucial for organizations aiming to leverage data effectively. This section explores key trends that are shaping the landscape of statistical analytics today, focusing on why they matter and how they can benefit various sectors.
Integration with Artificial Intelligence
AI-Driven Analytics
AI-driven analytics represents a seismic shift in how businesses interpret and utilize data. The core characteristic of AI-driven analytics is its ability to process large volumes of data at unprecedented speeds, enabling organizations to draw insights that were previously unattainable. For practitioners, one appealing aspect of this technology is its predictive capabilities. Unlike traditional methods which often analyze past data, AI algorithms can identify patterns and predict future outcomes based on vast datasets. This can lead to proactive decision-making, optimizing strategies before issues arise.
Moreover, AI-driven analytics excels in automating many of the time-consuming tasks associated with data analysis. This unique feature allows data professionals to focus on interpretation and strategy rather than on manual data gathering. However, challenges exist, particularly in the form of algorithmic bias, where the AI's training data may lead to flawed models. Addressing these disadvantages is critical for maintaining ethical standards in analytics.
Predictive Modeling
Predictive modeling is a specific subset of statistical analytics that benefits significantly from machine learning and AI technologies. Its defining characteristic is the creation of models that forecast future probabilities based on historical data. Predictive modeling is attractive to businesses and organizations due to its ability to minimize risks and maximize opportunities by providing actionable insights.
The unique feature of predictive modeling lies in its iterative nature: models can continuously improve as they are exposed to new data. This adaptability makes them particularly suited for volatile environments, where conditions change rapidly. However, itâs essential to remember that predictions are inherently uncertainâexcessive reliance on them can lead to misguided strategies if not complemented with human judgment and contextual understanding.
Automated Data Insights
Automated data insights represent another beacon of innovation in statistical analytics. This process minimizes the amount of manual analysis required, making data interpretation faster and often more accurate. The key characteristic of automated insights is their ability to provide real-time analysis, allowing organizations to stay one step ahead of the competition.
One significant advantage of this approach is the democratization of data access; stakeholders from various levels can obtain insights without needing extensive technical training. However, this can also be a double-edged swordâover-reliance on automated systems may obscure the need for critical thinking and thorough analytical skills. Finding the balance between automation and human intuition will be vital in coming years.
Cloud-Based Statistical Solutions
Scalability and Accessibility
Cloud-based statistical solutions have revolutionized access to data analytics tools. Their primary advantage is scalability; organizations can adjust their resources based on needs without incurring high overhead costs. This flexibility allows businesses of all shapes and sizes to harness the power of statistical analytics without making huge financial commitments.
Accessibility is another important aspect. With cloud services, teams can access data from anywhere, which fosters a culture of agility and collaboration. However, the reliance on Internet connectivity may pose challenges for organizations working in regions with limited bandwidth.
Collaborative Analytics
Collaborative analytics is about breaking down silos in data use within organizations. The key characteristic here is real-time collaboration, where team members can work together on the same data set regardless of physical location. This approach allows for diversity of thought and expertise during analysis, leading to more rounded insights.
One unique feature is the ability for cross-departmental collaborations, meaning marketing can engage with finance or product development teams in a shared space. While beneficial, maintaining clear communication during collaboration is critical to avoid misinformation or misinterpretation of data.
Cost-Effectiveness
Cost-effectiveness is a vital consideration in future trends. By utilizing cloud-based statistical solutions, organizations can often reduce their IT costs. Subscriptions to services can replace the need for expensive infrastructure and software purchases, making advanced analytics more accessible.
However, the cost-effectiveness of cloud solutions should be continually evaluated. Depending on usage and needs, monthly subscription fees can add up. Organizations must strike a balance between what they pay and the value they receive from these services.
The future of statistical analytics is not just about crunching numbers but understanding the stories and insights hidden within them. The trends we observe today will shape how decisions are made tomorrow, ensuring that data-driven approaches remain at the forefront of effective strategy.
Closure and Final Thoughts
As we wrap up this exploration into statistical analytics, it's essential to recognize just how pivotal this field is within today's data-centric environment. Statistical analytics transcends mere number-crunching; it is essentially the backbone of decision-making in various sectors. From businesses to healthcare, and even social sciences, decisions based on statistical analysis drive strategic planning and forecasting.
Summary of Key Points
- Importance of Data-Driven Decisions: Every point discussed throughout this article illustrates the necessity for businesses and professionals to lean on statistical analyses to guide their decisions. Without these insights, organizations risk making uninformed choices that can lead to significant financial drawbacks.
- Core Concepts Established: We delved into descriptive and inferential statistics, emphasizing how these concepts form the foundation of analytical practices. Understanding these two branches aligns one's skills with industry standards, aiding in effective data interpretation.
- Tools and Techniques: The exploration of software packages like R and Python highlighted how technology enhances analytic capabilities. This not only streamlines workflows but also opens doors for advanced analytics, contributing to more nuanced insights.
- Challenges Ahead: Addressing issues like data quality and misinterpretation is vital. Professionals must stay vigilant, ensuring their analyses are not only accurate but also relevant.
Recommendations for Practitioners
- Embrace Continuous Learning: The field of statistical analytics is ever-evolving. Practitioners should pursue ongoing education, whether through formal courses or self-guided research, to stay current with trends and technologies. Online platforms like Coursera and edX offer invaluable resources.
- Invest in Quality Data Collection Methods: Ensure that the data you rely on is collected and processed methodically. High-quality data leads to reliable resultsâafter all, garbage in means garbage out.
- Communicate Effectively: Statisticians must not only crunch numbers but also narrate the stories behind them. Use visuals and clear language to present findings, ensuring they resonate with your audience, whether they are technical experts or business executives.
- Integrate AI and Emerging Technologies: Keep an eye on how artificial intelligence can enhance statistical methods. AI-driven analytics can unearth patterns that traditional methods might overlookâconsider exploring these tools in your analytical arsenal.
This exploration into statistical analytics proves that itâs not about merely accumulating data; itâs about harnessing this data to drive informed decisions.
In summation, embracing the principles of statistical analytics holds the key to thriving in a data-driven world. It's crucial for professionals across various domains to understand and apply these analytics skills to navigate their respective landscapes effectively.